Meta-Learning and Self-Supervised Pretraining for Storm Event Imagery Translation
2023 IEEE HIGH PERFORMANCE EXTREME COMPUTING CONFERENCE, HPEC(2023)
摘要
Recent advances in deep learning have provided impressive results across a wide range of computational problems such as computer vision, natural language, or reinforcement learning. However, many of these improvements are constrained to problems with large-scale curated datasets which require a lot of human labor to gather. Additionally, these models tend to generalize poorly under both slight distributional shifts and low-data regimes. In recent years, emerging fields such as meta-learning and self-supervised learning have been closing the gap between proof-of-concept results and real-life applications of machine learning by extending deep learning to the semi-supervised and few-shot domains. We follow this line of work and explore spatiotemporal structure in a recently introduced image-to-image translation problem for storm event imagery in order to: i) formulate a novel multi-task few-shot image generation benchmark in the field of AI for Earth and Space Science and ii) explore data augmentations in contrastive pretraining for image translation downstream tasks. We present several baselines for the few-shot problem and discuss trade-offs between different approaches. Our implementation and instructions to reproduce the experiments, available at https://github.com/irugina/meta-image-translation, are thoroughly tested on MIT SuperCloud, and scalable to other state-of-the-art HPC systems.
更多查看译文
关键词
few-shot learning,self-supervised learning,meta-learning,generative adversarial networks
AI 理解论文
溯源树
样例
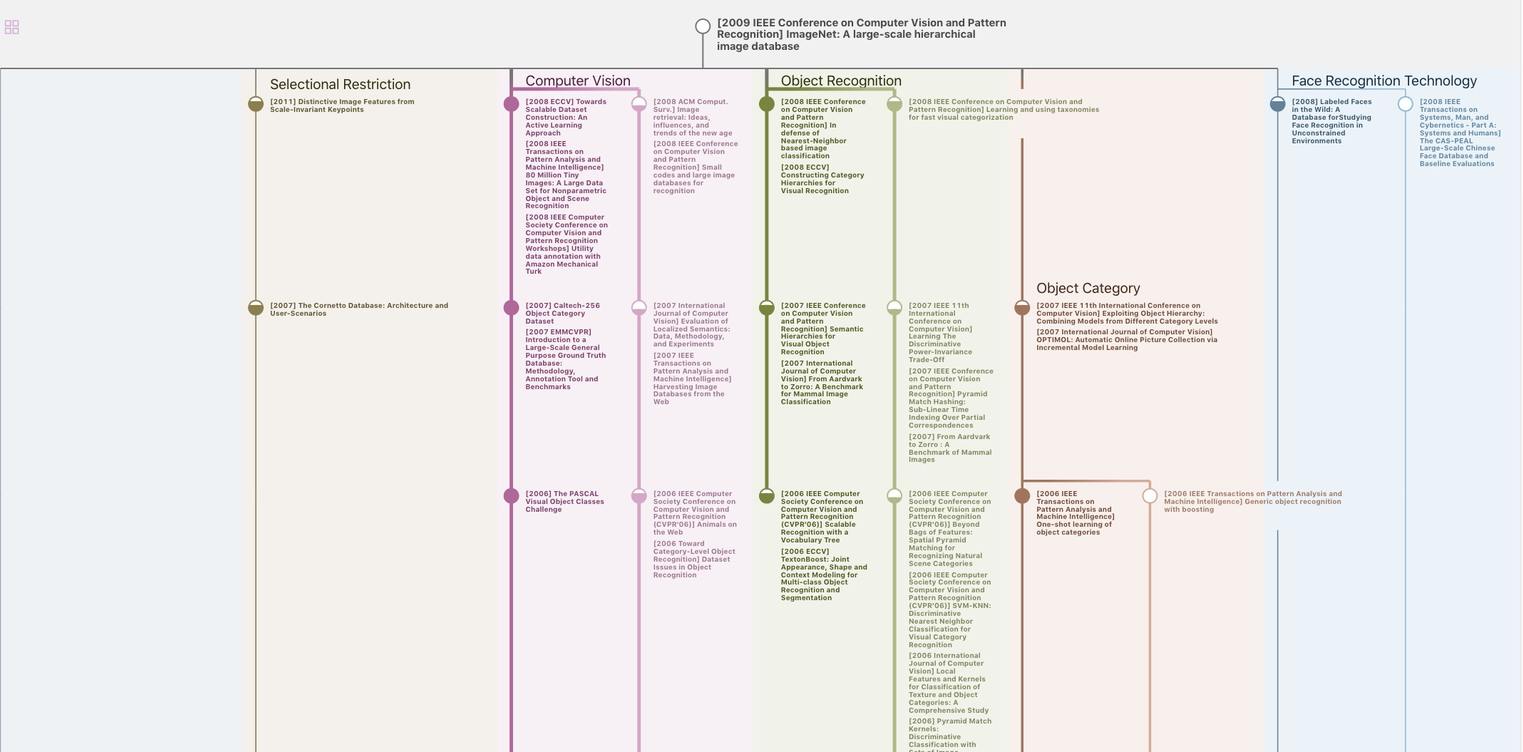
生成溯源树,研究论文发展脉络
Chat Paper
正在生成论文摘要