Federated Learning Based Satellite-Marine Integrated Training for Marine Edge Intelligence.
2023 IEEE Intl Conf on Dependable, Autonomic and Secure Computing, Intl Conf on Pervasive Intelligence and Computing, Intl Conf on Cloud and Big Data Computing, Intl Conf on Cyber Science and Technology Congress (DASC/PiCom/CBDCom/CyberSciTech)(2023)
摘要
Marine communication refers to the communication conducted between land and ocean or within the ocean. It constitutes a crucial component in achieving global network coverage. Within the existing marine-edge environments, a vast amount of edge data is generated. Leveraging the stability of ocean buoys, maritime edge training methods are poised to play a pivotal role in marine communication. However, due to the formidable challenges associated with ocean infrastructure development and the inherent characteristics of dispersed and privacy-sensitive ocean data, conventional edge training methodologies find substantial obstacles in implementation. To address these challenges, we propose a federated learning based Satellite-Marine integrated training approach, aiming to enhance marine-edge intelligence while safeguarding privacy. Firstly, we perform unsupervised clustering of marine-edge data, dynamically partitioning the edge network and designating marine-edge servers as central nodes. Subsequently, within each edge server, edge data is cached, and deep residual networks along with deep learning models are established to facilitate feature extraction from the marine-edge data. Finally, satellites are employed as global network hubs, enabling vertical federated learning to achieve aggregation of edge features. Experimental results demonstrate that, in the realm of regression prediction, compared to support vector machine and random forest models, the proposed network model in this study exhibits reduced error and higher efficiency.
更多查看译文
关键词
Edge training,integrated Satellite-Marine,vertical federated learning,deep learning
AI 理解论文
溯源树
样例
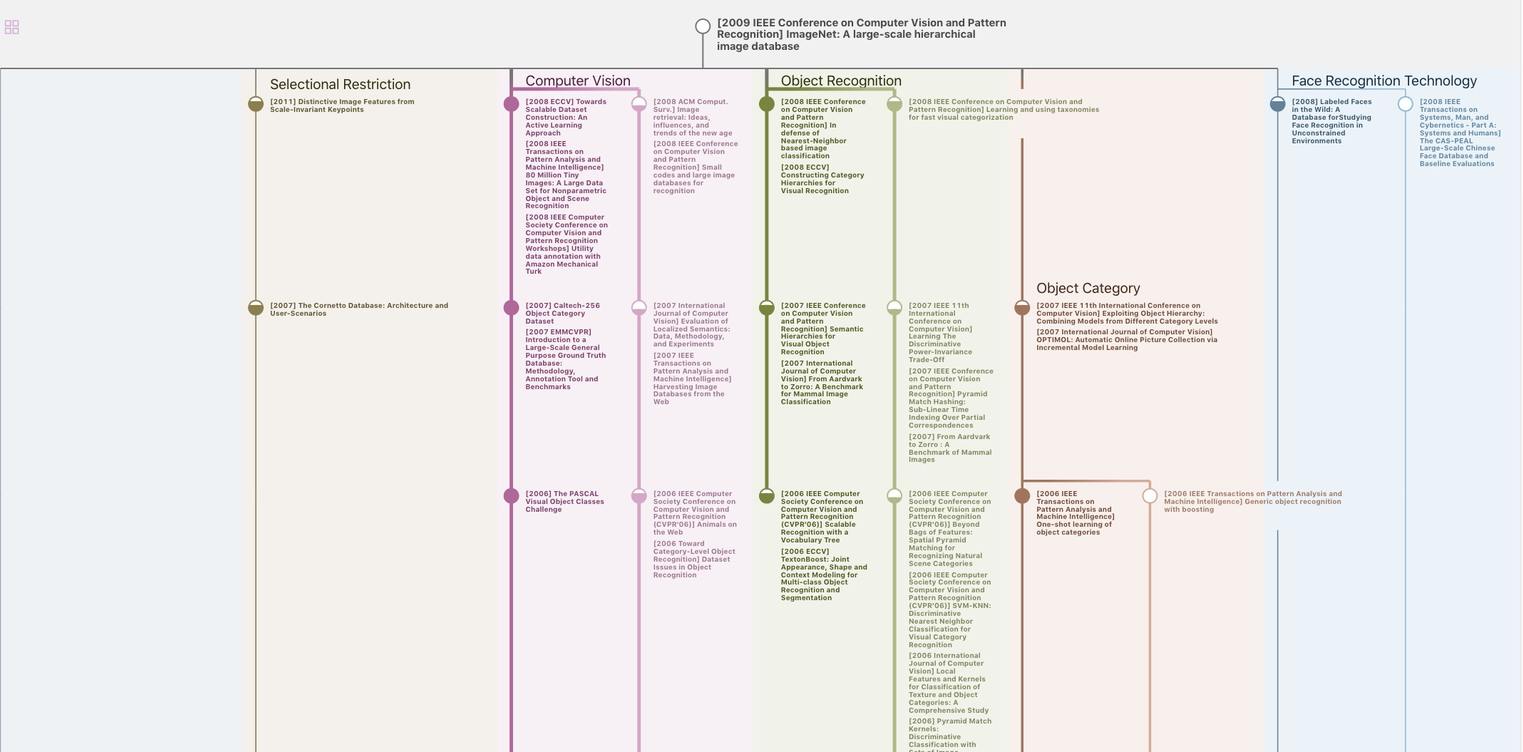
生成溯源树,研究论文发展脉络
Chat Paper
正在生成论文摘要