Adapting Vision Foundation Models for Plant Phenotyping
2023 IEEE/CVF INTERNATIONAL CONFERENCE ON COMPUTER VISION WORKSHOPS, ICCVW(2023)
摘要
Foundation models are large models pre-trained on tremendous amount of data. They can be typically adapted to diverse downstream tasks with minimal effort. However, as foundation models are usually pre-trained on images or texts sourced from the Internet, their performance in specialized domains, such as plant phenotyping, comes into question. In addition, fully fine-tuning foundation models is time-consuming and requires high computational power. This paper investigates the efficient adaptation of foundation models for plant phenotyping settings and tasks. We perform extensive experiments on fine-tuning three foundation models, MAE, DINO, and DINOv2 on three essential plant phenotyping tasks: leaf counting, instance segmentation, and disease classification. In particular, the pretrained backbones are kept frozen, while two distinct finetuning methods are evaluated, namely adapter tuning (using LoRA) and decoder tuning. The experimental results show that a foundation model can be efficiently adapted to multiple plant phenotyping tasks, yielding similar performance as the state-of-the-art (SoTA) models specifically designed or trained for each task. Despite exhibiting great transferability over different tasks, the fine-tuned foundation models perform slightly worse than the SoTA task-specific models in some scenarios, which requires further investigation.
更多查看译文
AI 理解论文
溯源树
样例
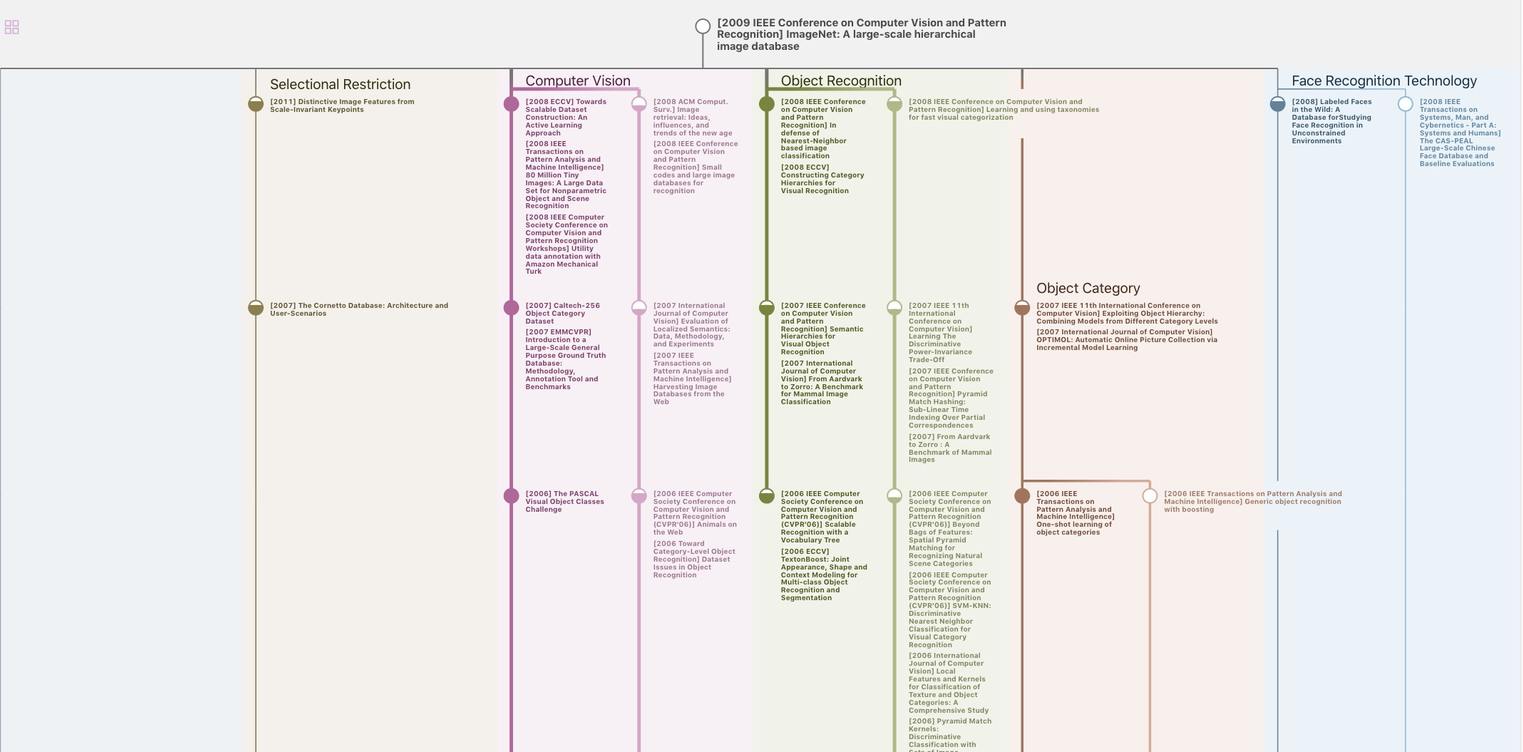
生成溯源树,研究论文发展脉络
Chat Paper
正在生成论文摘要