Visual Explanations: Activation-based Acute Lymphoblastic Leukemia Cell Classification
2023 IEEE INTERNATIONAL CONFERENCE ON DEVELOPMENT AND LEARNING, ICDL(2023)
摘要
The saliency methods are widely used for generating heatmaps that emphasize the important portions of an input image for deep networks on a specific classification task. Interpretability is crucial for deploying deep neural networks in real-world applications. However, the heatmaps produced by current visual explainable methods may contain or visualize particulars differently. To analyze and compare the visualization of different methods, such as Gradient-based, Activation-based, Perturbation-based, and Region-based methods, we empirically evaluated them on the acute lymphoblastic leukemia (cancer cell) classification task using state-of-the-art convolutional neural networks. We also visualized the essential pathological features (salient parts) that are the reasons for the classification results on the classification of normal versus malignant cell (CNMC) dataset.
更多查看译文
关键词
Explainable AI,Post-hoc Interpretability,Visual explanations,Cancer cell classification
AI 理解论文
溯源树
样例
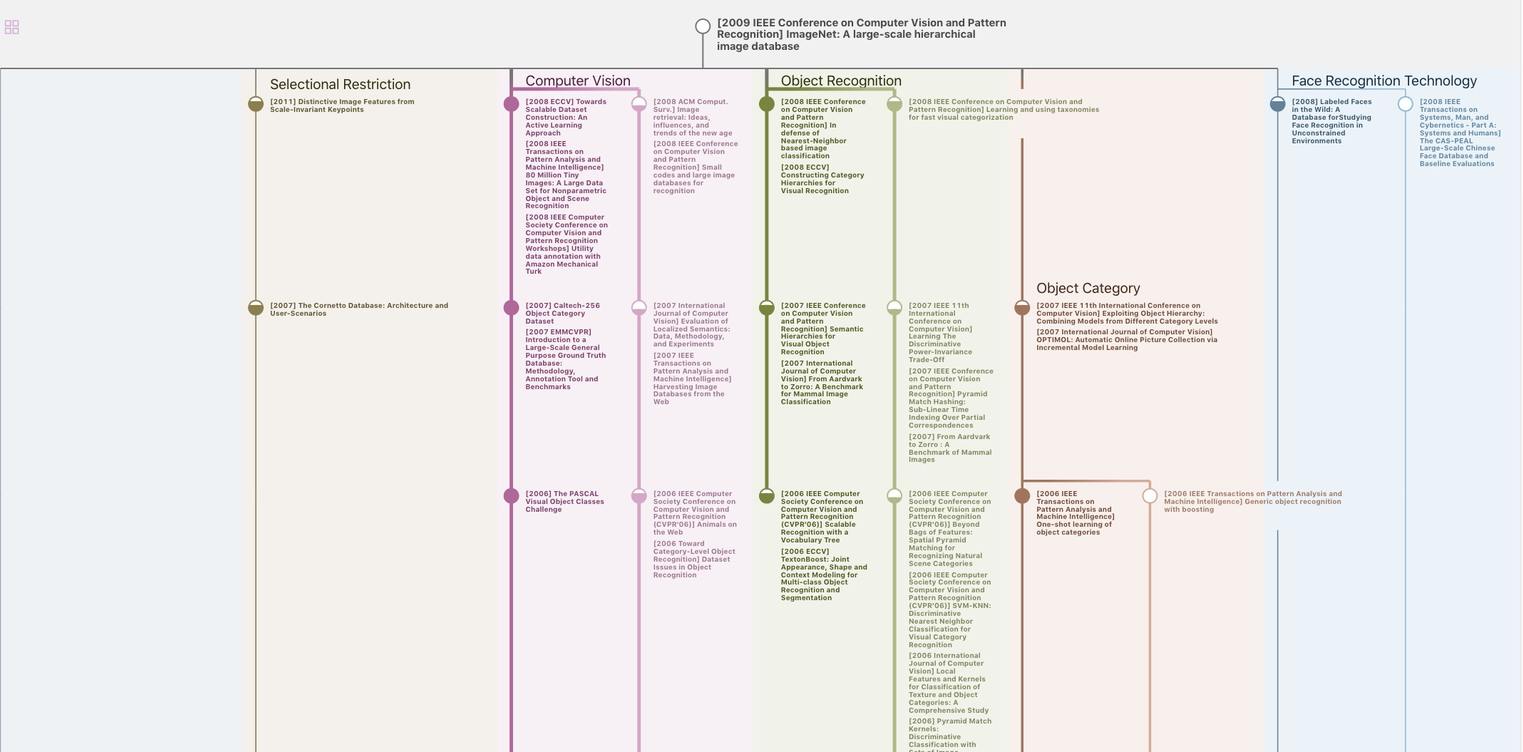
生成溯源树,研究论文发展脉络
Chat Paper
正在生成论文摘要