Real-World Robot Control and Data Augmentation by World-Model Learning from Play
2023 IEEE INTERNATIONAL CONFERENCE ON DEVELOPMENT AND LEARNING, ICDL(2023)
摘要
Building generalist robots that can perform well in complex environments requires high generalization ability. Imitation learning and reinforcement learning are two promising approaches, but they have a tradeoff between data collection cost and data richness. To overcome this tradeoff, we focus on "play data" collected by humans teleoperating a robot out of curiosity. Specifically, we propose a framework for real-world robot control and data augmentation based on world-model learning from play data. We demonstrate that our framework can be used to train robots to perform goal-conditioned object-manipulation tasks in the real world. Furthermore, we show that imagination in the world model can be used to create novel combinations of actions that were not included in the original play data. These findings suggest that further learning from the augmented data may enable robots to acquire a higher generalization ability.
更多查看译文
AI 理解论文
溯源树
样例
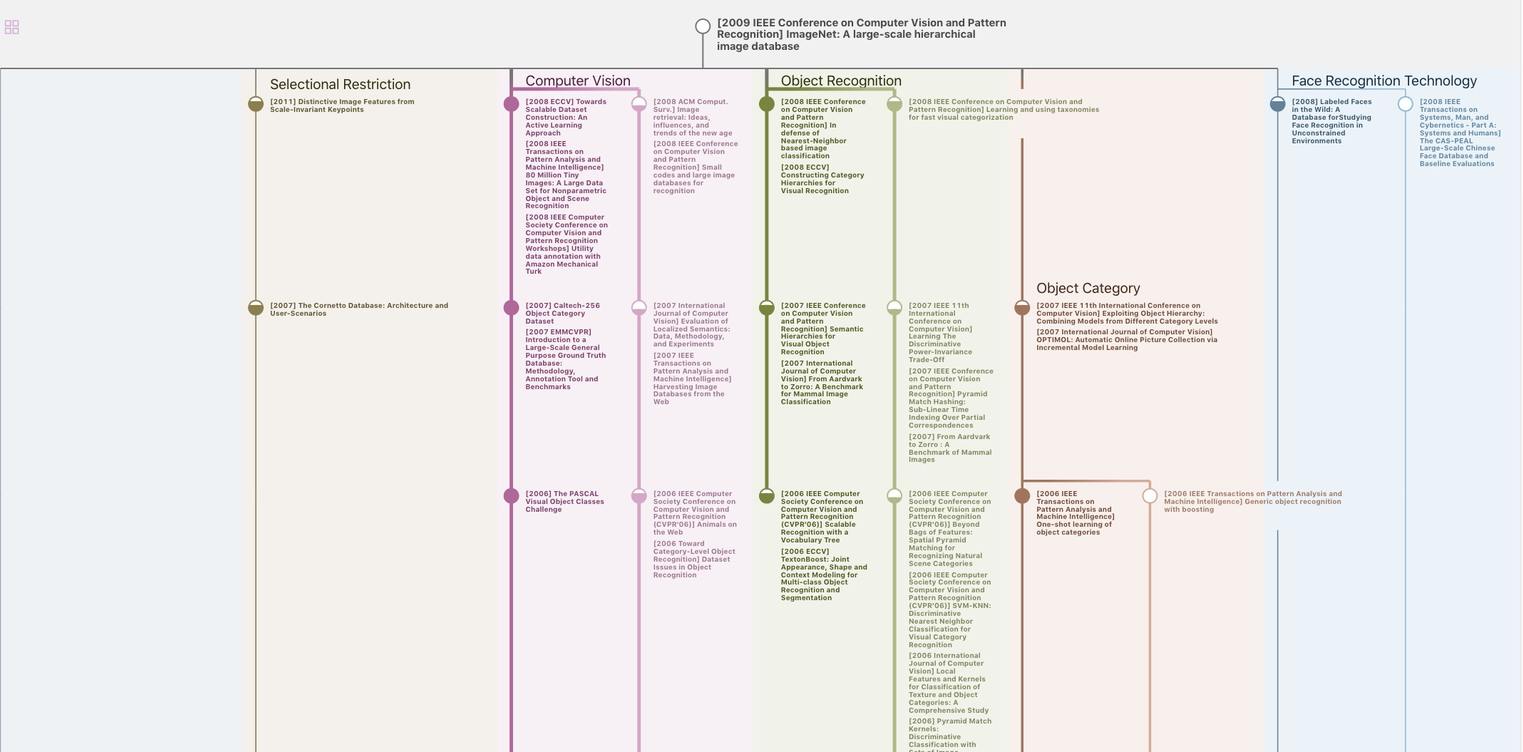
生成溯源树,研究论文发展脉络
Chat Paper
正在生成论文摘要