GAN Inversion Based Point Clouds Denoising in Foggy Scenarios for Autonomous Driving
2023 IEEE INTERNATIONAL CONFERENCE ON DEVELOPMENT AND LEARNING, ICDL(2023)
摘要
Currently, point clouds captured in adverse weather conditions are often subjected to noise, which significantly impacts the reliability of autonomous driving perception systems. To address this issue, we propose a method for denoising point clouds under adverse weather conditions by utilizing a pre-trained generative model to establish a GAN inversion network. To simulate foggy driving scenarios, we construct the Foggy KITTI dataset and pre-train the GAN to capture rich semantic information. The experimental results demonstrate that the proposed method outperforms other denoising methods, indicating its effectiveness in enhancing the quality of point clouds. Additionally, our method exhibits good generalization ability on real-world datasets, indicating its potential as a preprocessing component for other point cloud processing tasks.
更多查看译文
关键词
autonomous driving,denoising,GAN,Foggy KITTI dataset
AI 理解论文
溯源树
样例
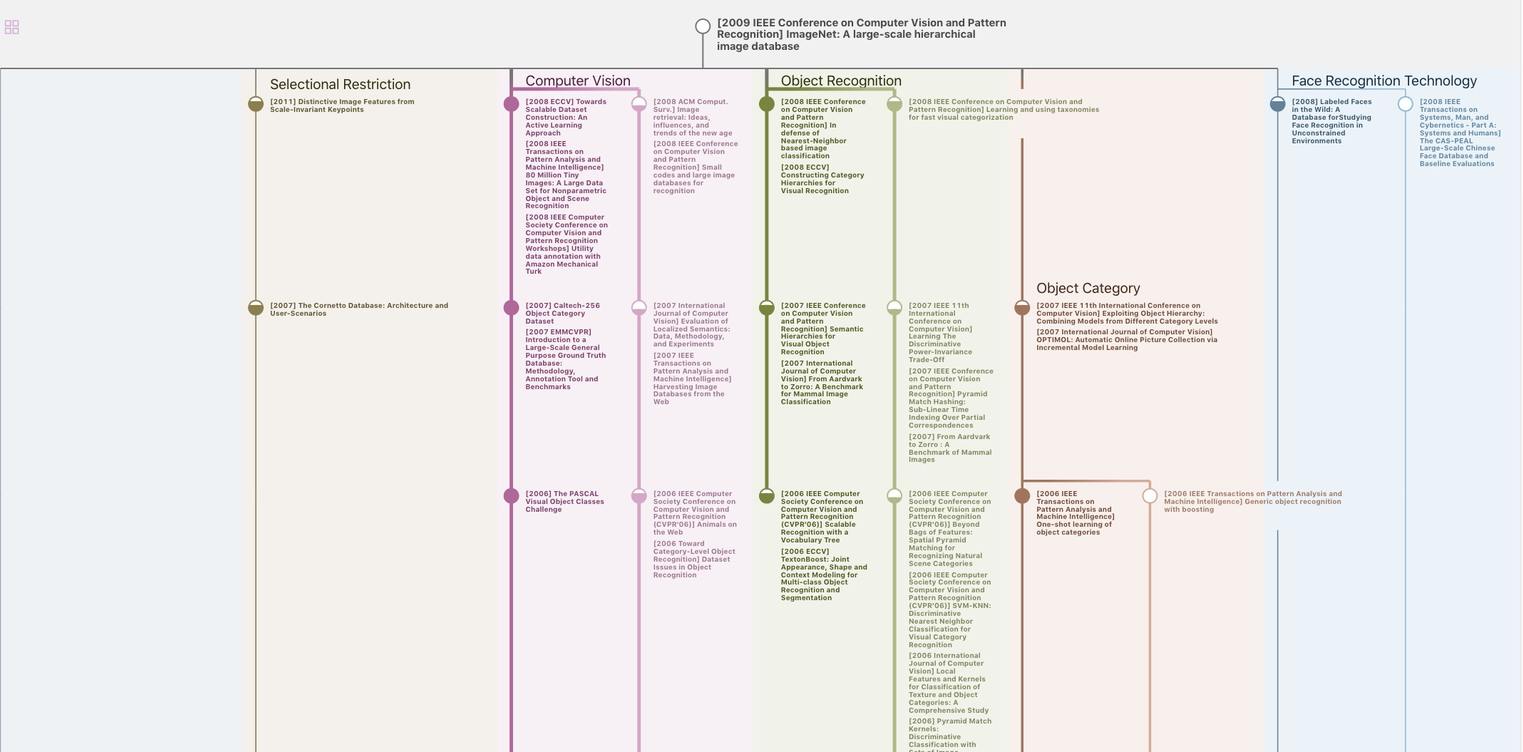
生成溯源树,研究论文发展脉络
Chat Paper
正在生成论文摘要