Few-Shot Fault Diagnosis Based on an Attention-Weighted Relation Network
ENTROPY(2024)
摘要
As energy conversion systems continue to grow in complexity, pneumatic control valves may exhibit unexpected anomalies or trigger system shutdowns, leading to a decrease in system reliability. Consequently, the analysis of time-domain signals and the utilization of artificial intelligence, including deep learning methods, have emerged as pivotal approaches for addressing these challenges. Although deep learning is widely used for pneumatic valve fault diagnosis, the success of most deep learning methods depends on a large amount of labeled training data, which is often difficult to obtain. To address this problem, a novel fault diagnosis method based on the attention-weighted relation network (AWRN) is proposed to achieve fault detection and classification with small sample data. In the proposed method, fault diagnosis is performed through the relation network in few-shot learning, and in order to enhance the representativeness of feature extraction, the attention-weighted mechanism is introduced into the relation network. Finally, in order to verify the effectiveness of the method, a DA valve fault dataset is constructed, and experimental validation is performed on this dataset and another benchmark PU rolling bearing fault dataset. The results show that the accuracy of the network on DA is 99.15%, and the average accuracy on PU is 98.37%. Compared with the state-of-the-art diagnosis methods, the proposed method achieves higher accuracy while significantly reducing the amount of training data.
更多查看译文
关键词
fault diagnosis,energy conversion systems,relation network,attention mechanism,pneumatic control valve
AI 理解论文
溯源树
样例
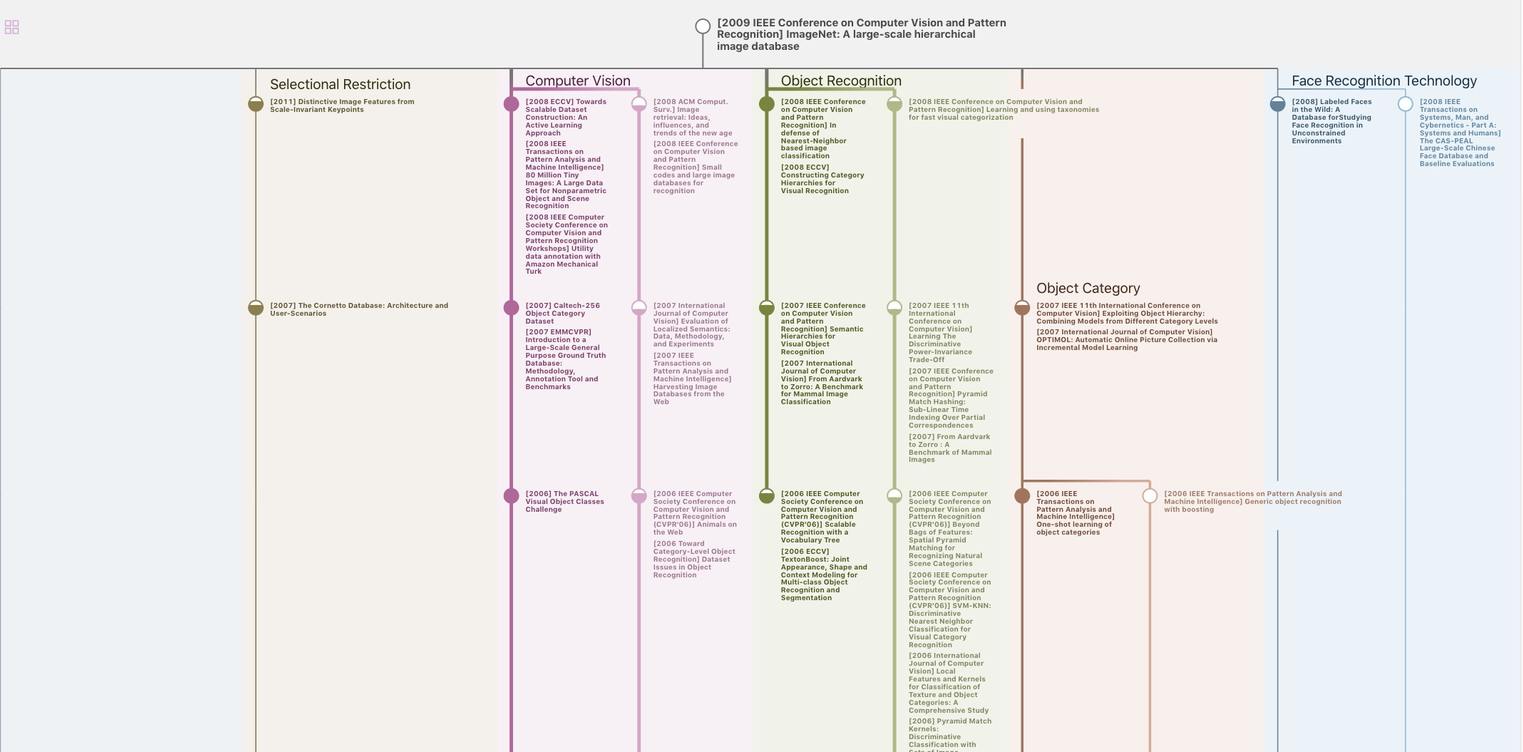
生成溯源树,研究论文发展脉络
Chat Paper
正在生成论文摘要