Spectral-Spatial Feature Extraction for Hyperspectral Image Classification Using Enhanced Transformer with Large-Kernel Attention
REMOTE SENSING(2024)
摘要
In the hyperspectral image (HSI) classification task, every HSI pixel is labeled as a specific land cover category. Although convolutional neural network (CNN)-based HSI classification methods have made significant progress in enhancing classification performance in recent years, they still have limitations in acquiring deep semantic features and face the challenges of escalating computational costs with increasing network depth. In contrast, the Transformer framework excels in expressing high-level semantic features. This study introduces a novel classification network by extracting spectral-spatial features with an enhanced Transformer with Large-Kernel Attention (ETLKA). Specifically, it utilizes distinct branches of three-dimensional and two-dimensional convolutional layers to extract more diverse shallow spectral-spatial features. Additionally, a Large-Kernel Attention mechanism is incorporated and applied before the Transformer encoder to enhance feature extraction, augment comprehension of input data, reduce the impact of redundant information, and enhance the model's robustness. Subsequently, the obtained features are input to the Transformer encoder module for feature representation and learning. Finally, a linear layer is employed to identify the first learnable token for sample label acquisition. Empirical validation confirms the outstanding classification performance of ETLKA, surpassing several advanced techniques currently in use. This research provides a robust and academically rigorous solution for HSI classification tasks, promising significant contributions in practical applications.
更多查看译文
关键词
CNN,Transformer,spectral-spatial feature,HSI
AI 理解论文
溯源树
样例
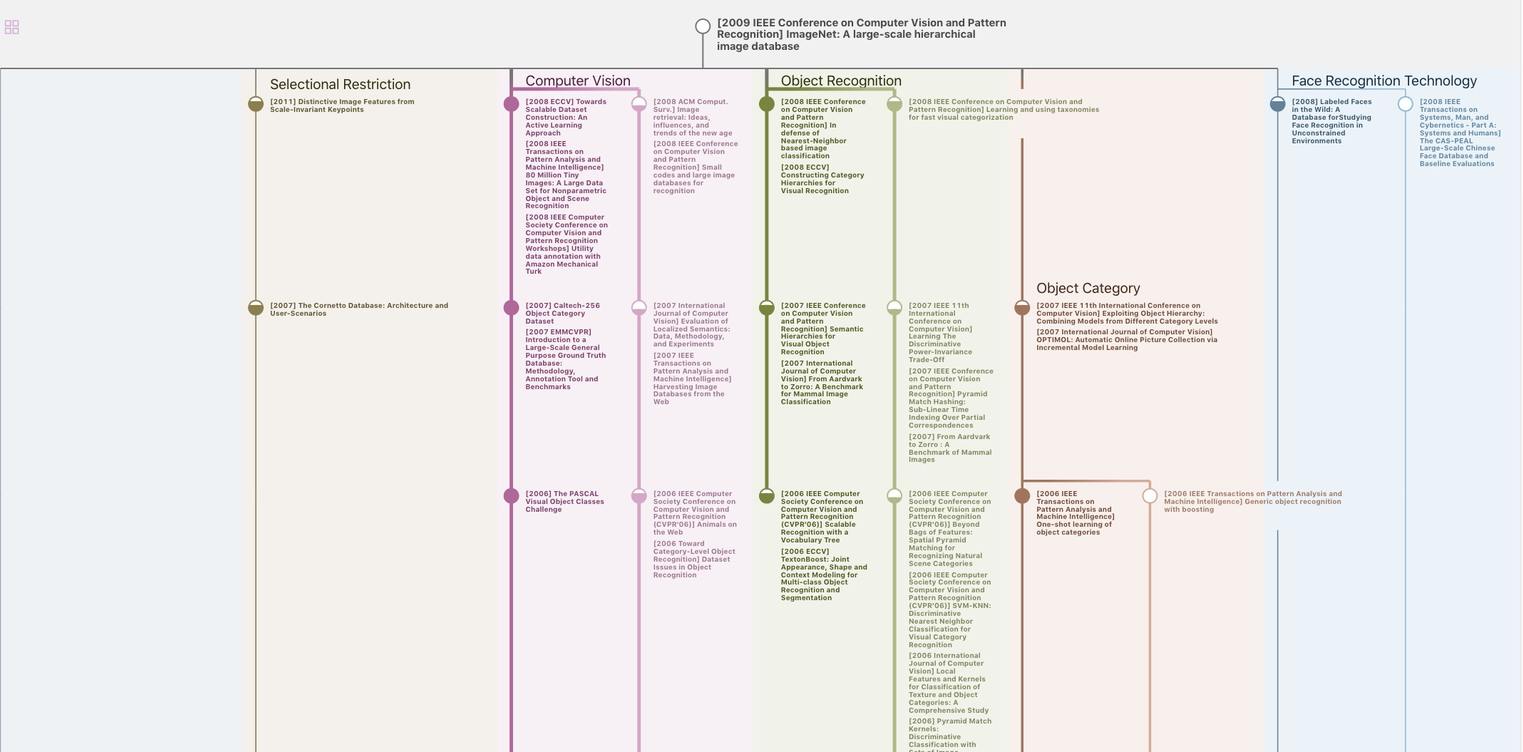
生成溯源树,研究论文发展脉络
Chat Paper
正在生成论文摘要