Explainable recommendation based on fusion representation of multi-type feature embedding
The Journal of Supercomputing(2023)
摘要
In e-commerce recommender systems, the sparsity of user-item rating data limits the quality of semantic embedding representation of users and items, which affects the accuracy of rating prediction. Previous studies have focused on learning attribute features or interaction features of nodes for recommendation and ignore the preference interest of rating tags in terms of attributes. In this paper, we propose a novel fusion representation method of multi-type node feature embedding for recommendation (MultiFEFR). The framework introduces rating preferences in terms of attributes and interactions and develops three kinds of feature presentations including rating-aware interaction feature embedding, rating-aware attribute feature embedding and heterogeneous attribute matched embedding. We design a multi-task joint learning strategy to optimize the accuracy of rating prediction. Experimental results show that the MultiFEFR outperforms the state-of-the-art models in MAE and RMSE metrics. Our findings are that the fusion of multi-type feature embedding can improve the accuracy of recommendation and the preference of attribute and interaction features are very explanatory for score grades.
更多查看译文
关键词
Recommender systems,Feature fusion,Score-aware embedding,Collaborative filtering
AI 理解论文
溯源树
样例
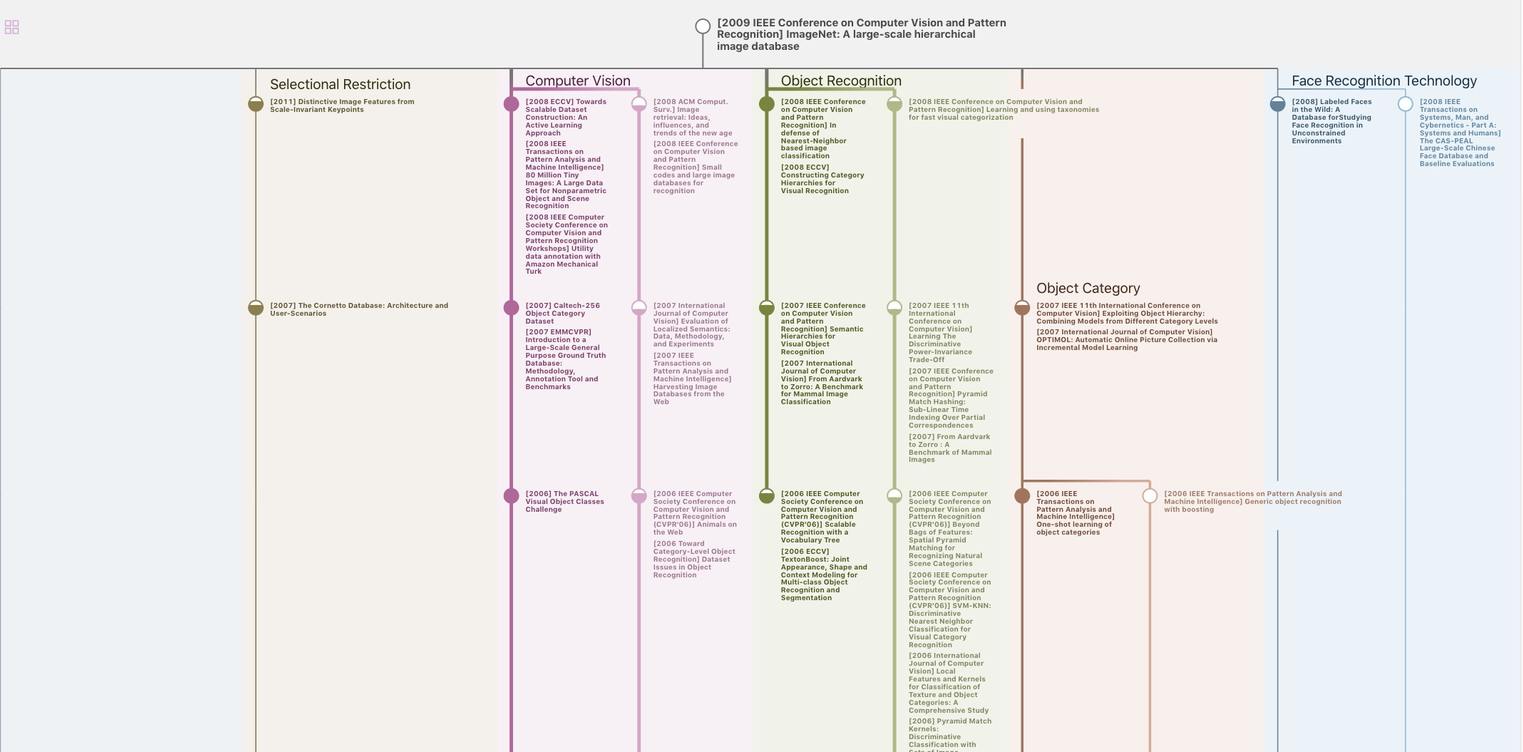
生成溯源树,研究论文发展脉络
Chat Paper
正在生成论文摘要