Unsupervised learning-based fast CU size decision for geometry videos in V-PCC
Journal of Real-Time Image Processing(2024)
摘要
In video-based point cloud compression (V-PCC), the geometry video and attribute video are generated through the projection of the 3D dynamic point clouds (DPCs), which can be further encoded with the High-Efficiency Video Coding (HEVC) standard. However, due to the huge amount of data in the DPC, the coding complexity is extremely high when encoding the geometry and attribute videos with V-PCC. In this work, we focus on compression for geometry videos which is completely different from natural videos. As a new coding structure has been adopted to encode the geometry video with V-PCC under the All Intra (AI) configuration, the existing methods to reduce the coding complexity are not suitable for geometry videos. Hence, we propose a fast Coding Unit (CU) size decision method for geometry videos based on unsupervised learning. As the geometry video contains regions of sharp edges and different scales, we design a novel hierarchical clustering method to determine the optimal CU sizes for encoding. In addition, an adaptive linkage threshold is introduced in the clustering process according to different quantization parameters and CU sizes. Experimental results show that, under the AI configuration, the savings in the coding time for the geometry videos from different DPCs achieved by the proposed approach range from 56.7% to 69.3% on average while only 0.1–0.5% of increments in Bjøntegaard delta total rate (BD-TotalRate) can be observed. Compared to various competing methods, the proposed approach achieves a better balance between coding complexity and encoding loss.
更多查看译文
关键词
3D point cloud,Dynamic point cloud compression,CU size decision,Hierarchical clustering,Unsupervised learning
AI 理解论文
溯源树
样例
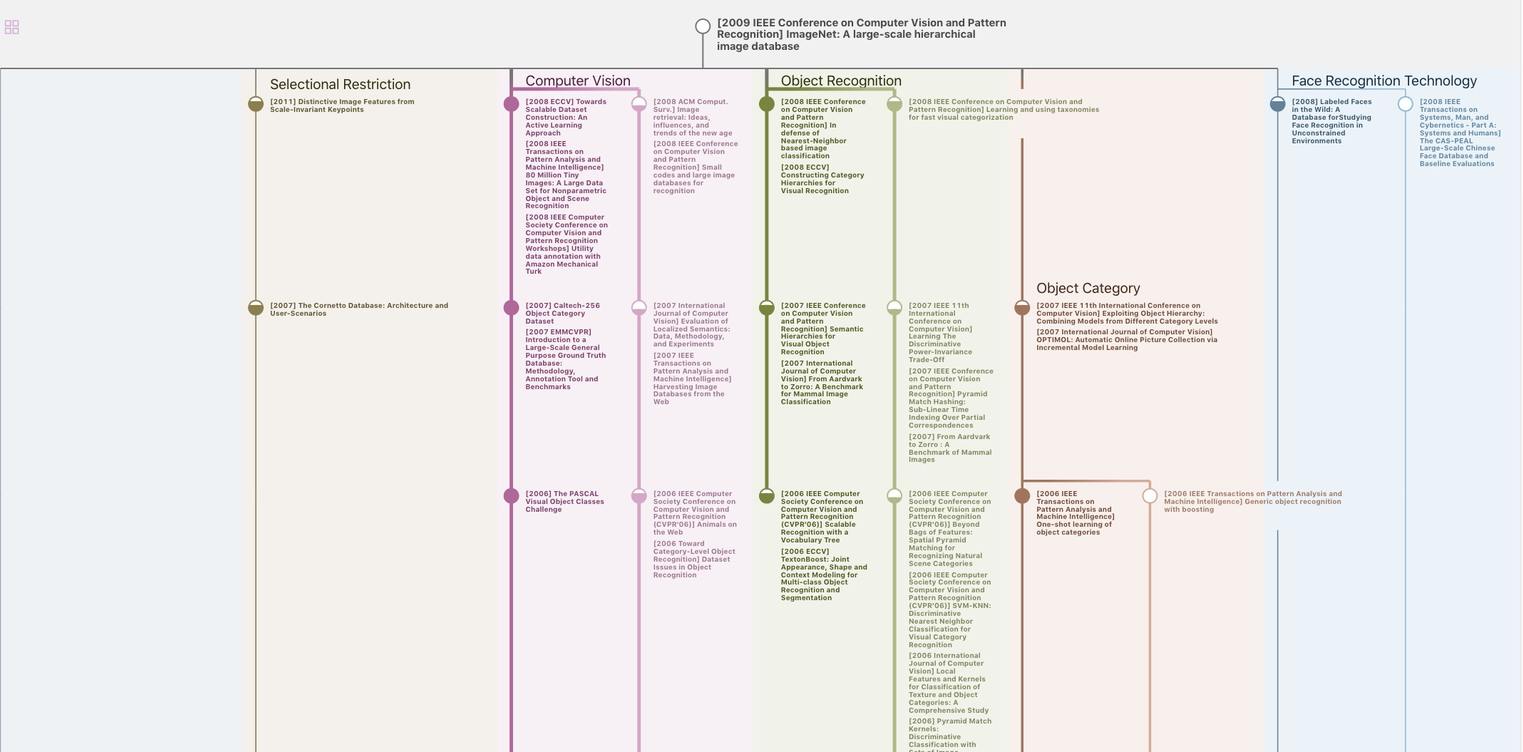
生成溯源树,研究论文发展脉络
Chat Paper
正在生成论文摘要