Large-Scale Subspace Clustering Based on Purity Kernel Tensor Learning
ELECTRONICS(2024)
摘要
In conventional subspace clustering methods, affinity matrix learning and spectral clustering algorithms are widely used for clustering tasks. However, these steps face issues, including high time consumption and spatial complexity, making large-scale subspace clustering ((LSC)-C-2) tasks challenging to execute effectively. To address these issues, we propose a large-scale subspace clustering method based on pure kernel tensor learning ((PKTLSC)-C-2). Specifically, we design a pure kernel tensor learning (PKT) method to acquire as much data feature information as possible while ensuring model robustness. Next, we extract a small sample dataset from the original data and use PKT to learn its affinity matrix while simultaneously training a deep encoder. Finally, we apply the trained deep encoder to the original large-scale dataset to quickly obtain its projection sparse coding representation and perform clustering. Through extensive experiments on large-scale real datasets, we demonstrate that the (PKTLSC)-C-2 method outperforms existing (LSC)-C-2 methods in clustering performance.
更多查看译文
关键词
cluster analysis,(LSC)-C-2,sparse coding,kernel tensor
AI 理解论文
溯源树
样例
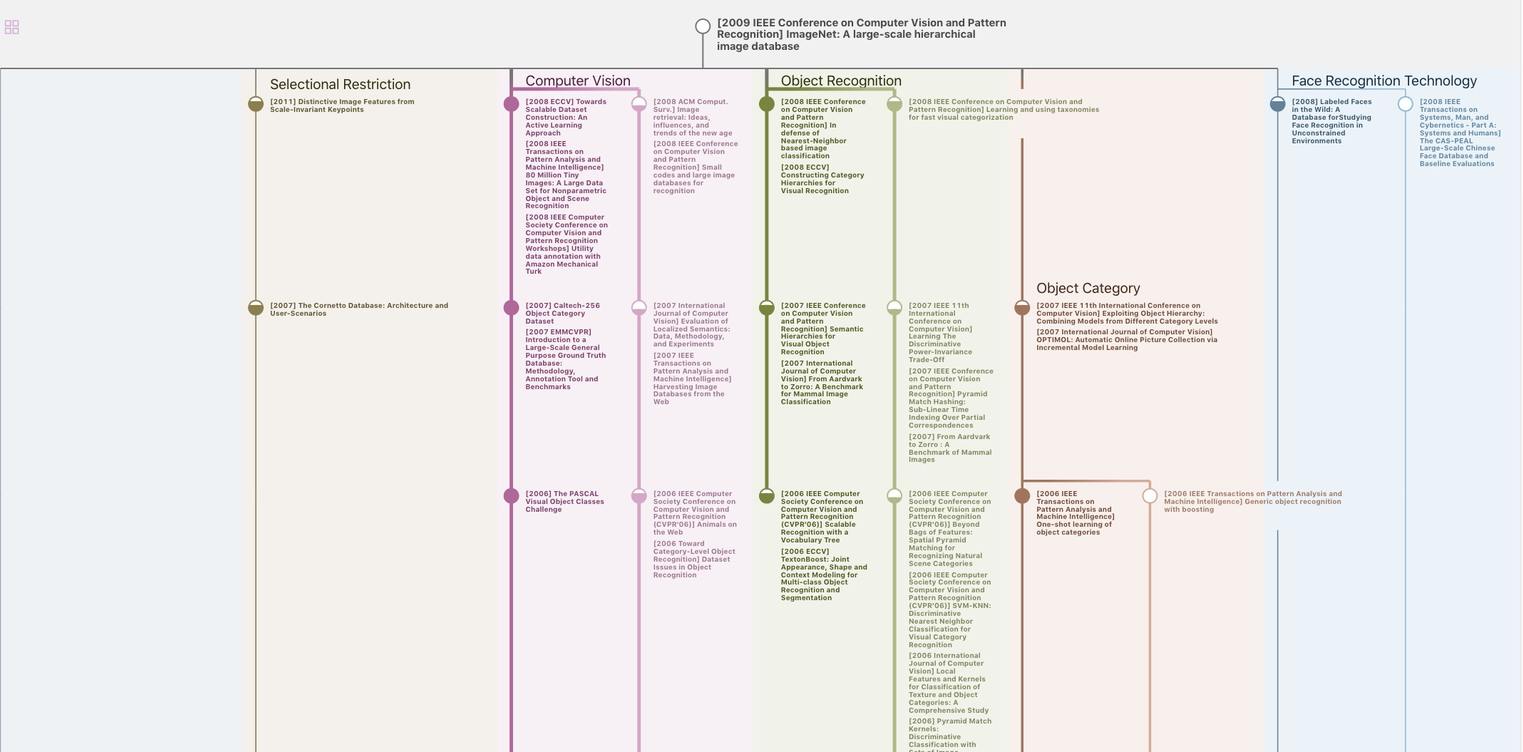
生成溯源树,研究论文发展脉络
Chat Paper
正在生成论文摘要