Application of machine learning in chemical engineering: outlook and perspectives
IAES International Journal of Artificial Intelligence (IJ-AI)(2024)
摘要
Chemical engineers' formulation, development, and stance processes all heavily rely on models. The physical and economic consequences of these decisions can have disastrous effects. Attempts to employ a hybrid form of artificial intelligence for modeling in various disciplines. However, they fell short of expectations. Due to a rise in the amount of data and computational resources during the previous five years. A lot of recent work has gone into developing new data sources, indexes, chemical interface designs, and machine learning algorithms in an effort to facilitate the adoption of these techniques in the research community. However, there are some important downsides to machine learning gains. The most promising uses for machine learning are in time-critical tasks like real-time optimization and planning that require extreme precision and can build on models that can self-learn to recognize patterns, draw conclusions from data, and become more intelligent over time. Due to their limited exposure to computer science and data analysis, the majority of chemical engineers are potentially vulnerable to the development of artificial intelligence. But in the not-too-distant future, chemical engineers' modeling toolbox will include a reliable machine learning component.
更多查看译文
AI 理解论文
溯源树
样例
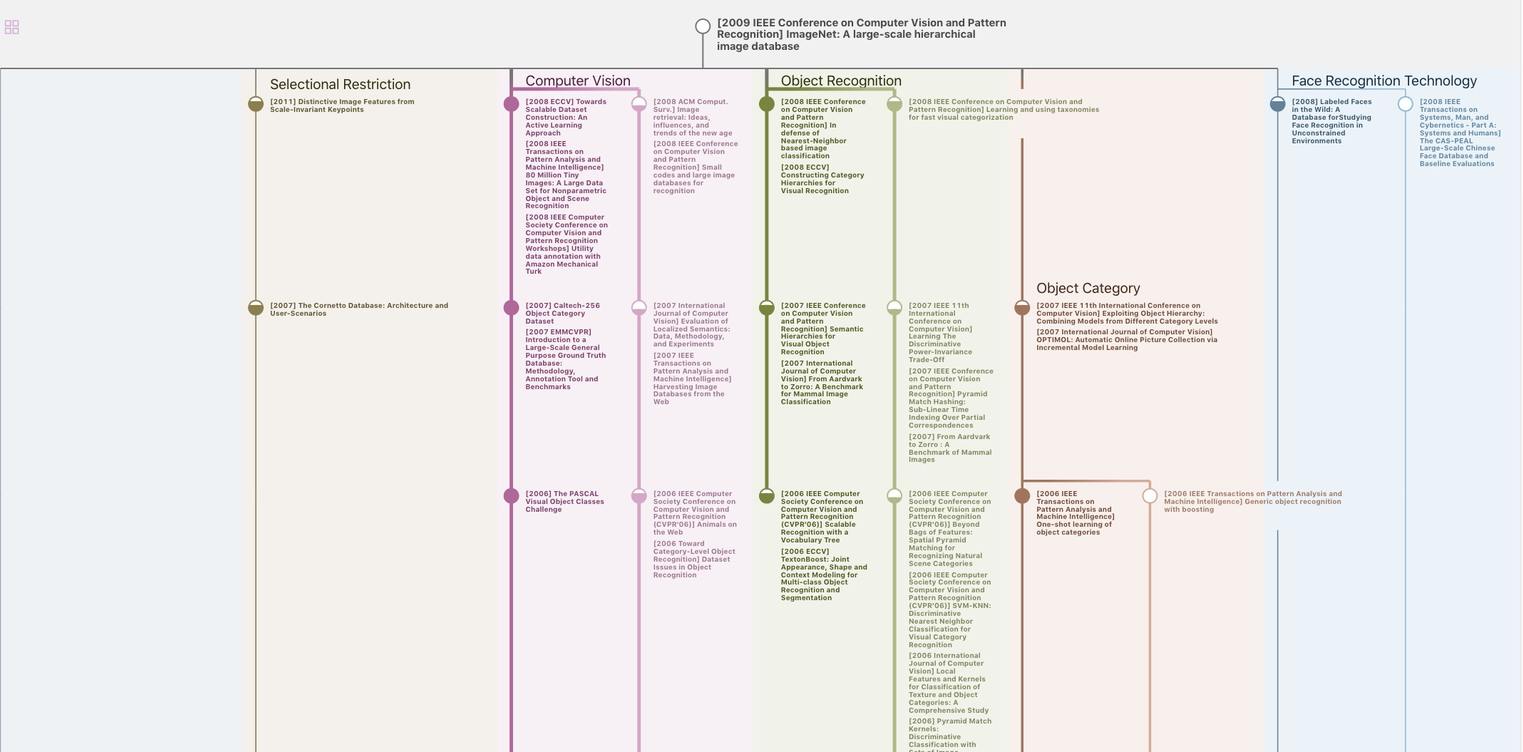
生成溯源树,研究论文发展脉络
Chat Paper
正在生成论文摘要