Global Rank Sum Test: An Efficient Rank-Based Nonparametric Test for Large Scale Online Experiment
arxiv(2023)
摘要
Online experiments are widely used for improving online services. While doing
online experiments, The student t-test is the most widely used hypothesis
testing technique. In practice, however, the normality assumption on which the
t-test depends on may fail, which resulting in untrustworthy results. In this
paper, we first discuss the question of when the t-test fails, and thus
introduce the rank-sum test. Next, in order to solve the difficulties while
implementing rank-sum test in large online experiment platforms, we proposed a
global-rank-sum test method as an improvement for the traditional one. Finally,
we demonstrate that the global-rank-sum test is not only more accurate and has
higher statistical power than the t-test, but also more time efficient than the
traditional rank-sum test, which eventually makes it possible for large online
experiment platforms to use.
更多查看译文
AI 理解论文
溯源树
样例
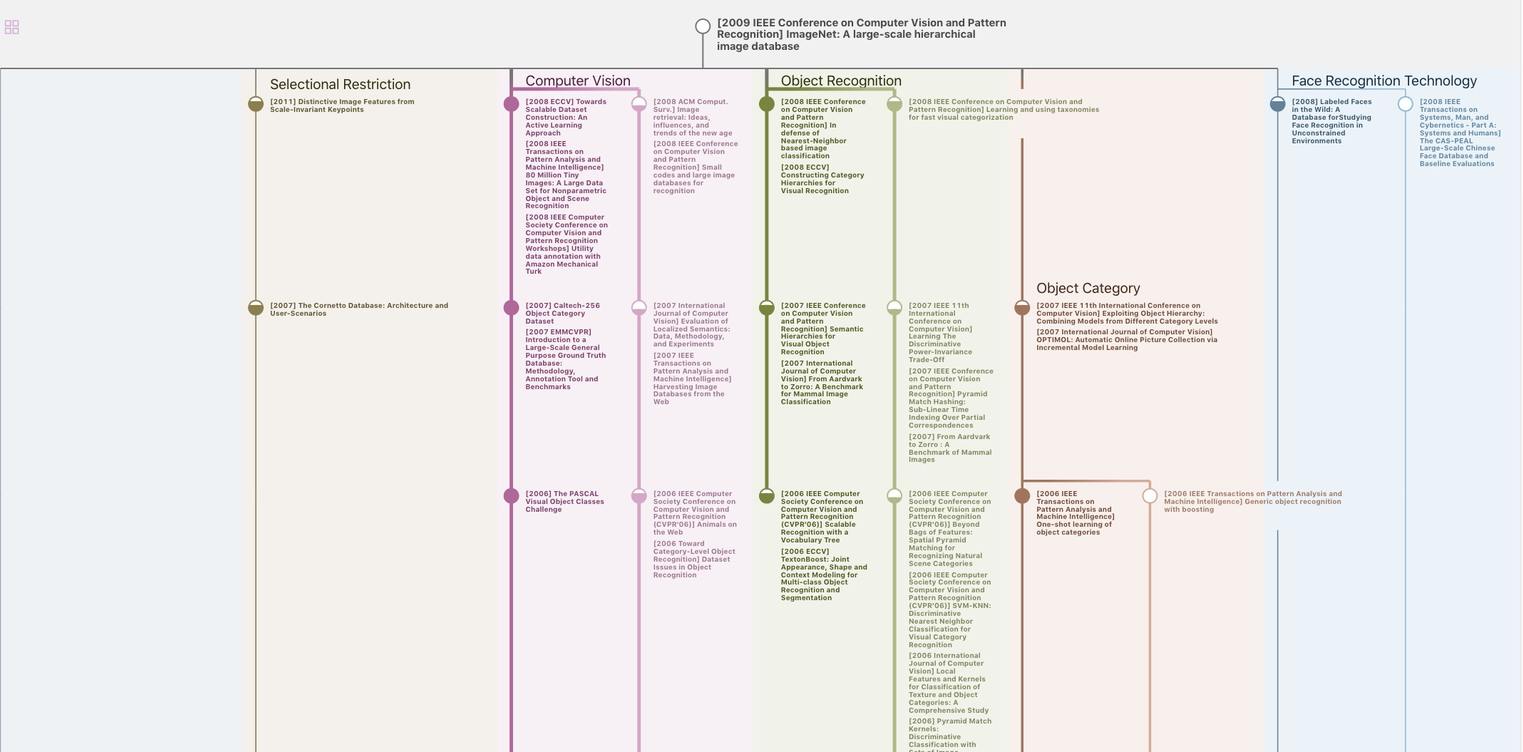
生成溯源树,研究论文发展脉络
Chat Paper
正在生成论文摘要