Unsupervised Harmonic Parameter Estimation Using Differentiable DSP and Spectral Optimal Transport
CoRR(2023)
摘要
In neural audio signal processing, pitch conditioning has been used to
enhance the performance of synthesizers. However, jointly training pitch
estimators and synthesizers is a challenge when using standard audio-to-audio
reconstruction loss, leading to reliance on external pitch trackers. To address
this issue, we propose using a spectral loss function inspired by optimal
transportation theory that minimizes the displacement of spectral energy. We
validate this approach through an unsupervised autoencoding task that fits a
harmonic template to harmonic signals. We jointly estimate the fundamental
frequency and amplitudes of harmonics using a lightweight encoder and
reconstruct the signals using a differentiable harmonic synthesizer. The
proposed approach offers a promising direction for improving unsupervised
parameter estimation in neural audio applications.
更多查看译文
关键词
differentiable signal processing,machine learning,optimal transport,frequency estimation
AI 理解论文
溯源树
样例
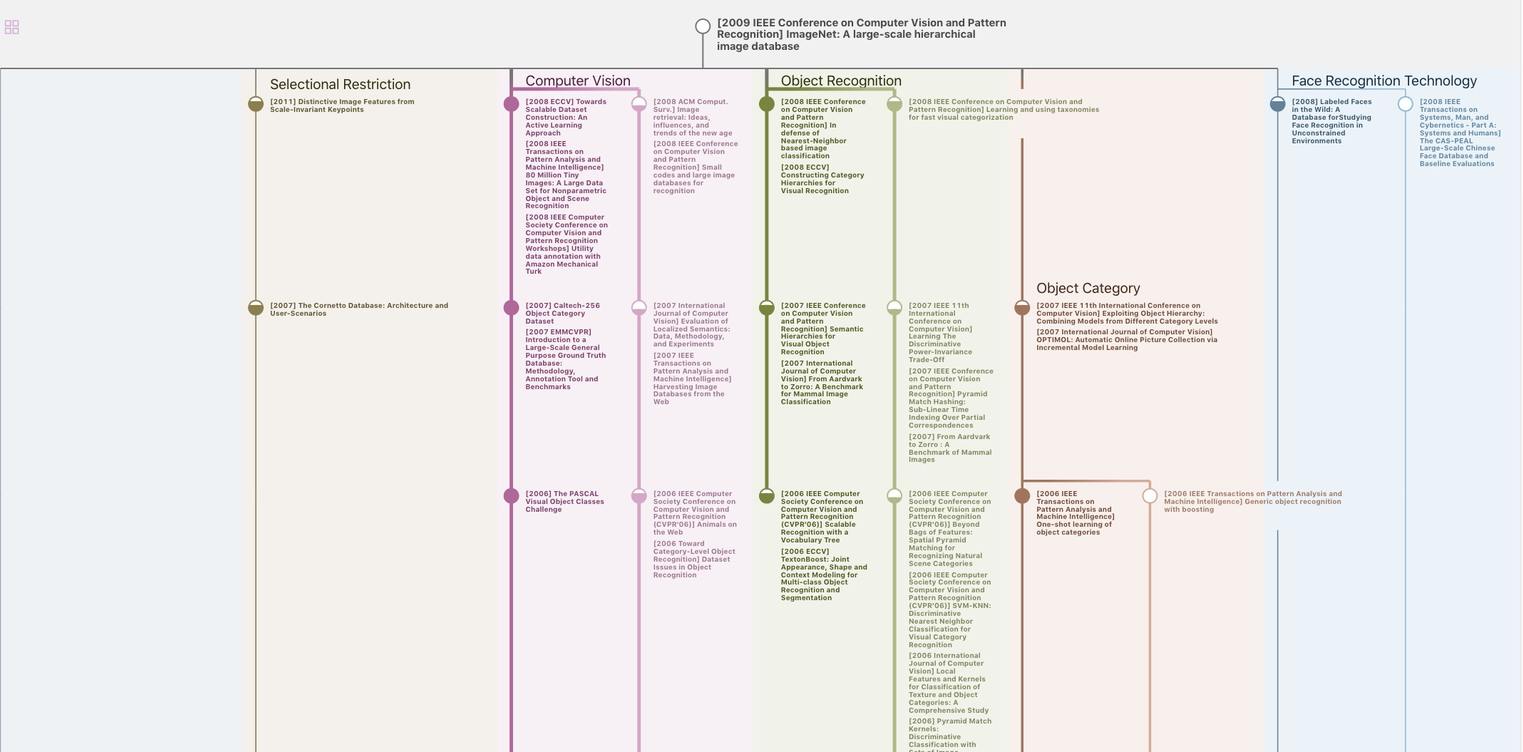
生成溯源树,研究论文发展脉络
Chat Paper
正在生成论文摘要