Equivariant neural network for Green's functions of molecules and materials
PHYSICAL REVIEW B(2024)
摘要
The many -body Green's function provides access to electronic properties beyond density -functional theory level in ab inito calculations. In this manuscript, we propose a deep learning framework for predicting the finite -temperature Green's function in atomic orbital space, aiming to achieve a balance between accuracy and efficiency. By predicting the self -energy matrices in Lehmann representation using an equivariant messagepassing neural network, our method respects its analytical property and the E (3) equivariance. The Green's function is obtained from the predicted self -energy through the Dyson equation with target total number of electrons. We present proof -of -concept benchmark results for both molecules and simple periodic systems, showing that our method is able to provide an accurate estimate of physical observables such as energy and density of states based on the predicted Green's function.
更多查看译文
AI 理解论文
溯源树
样例
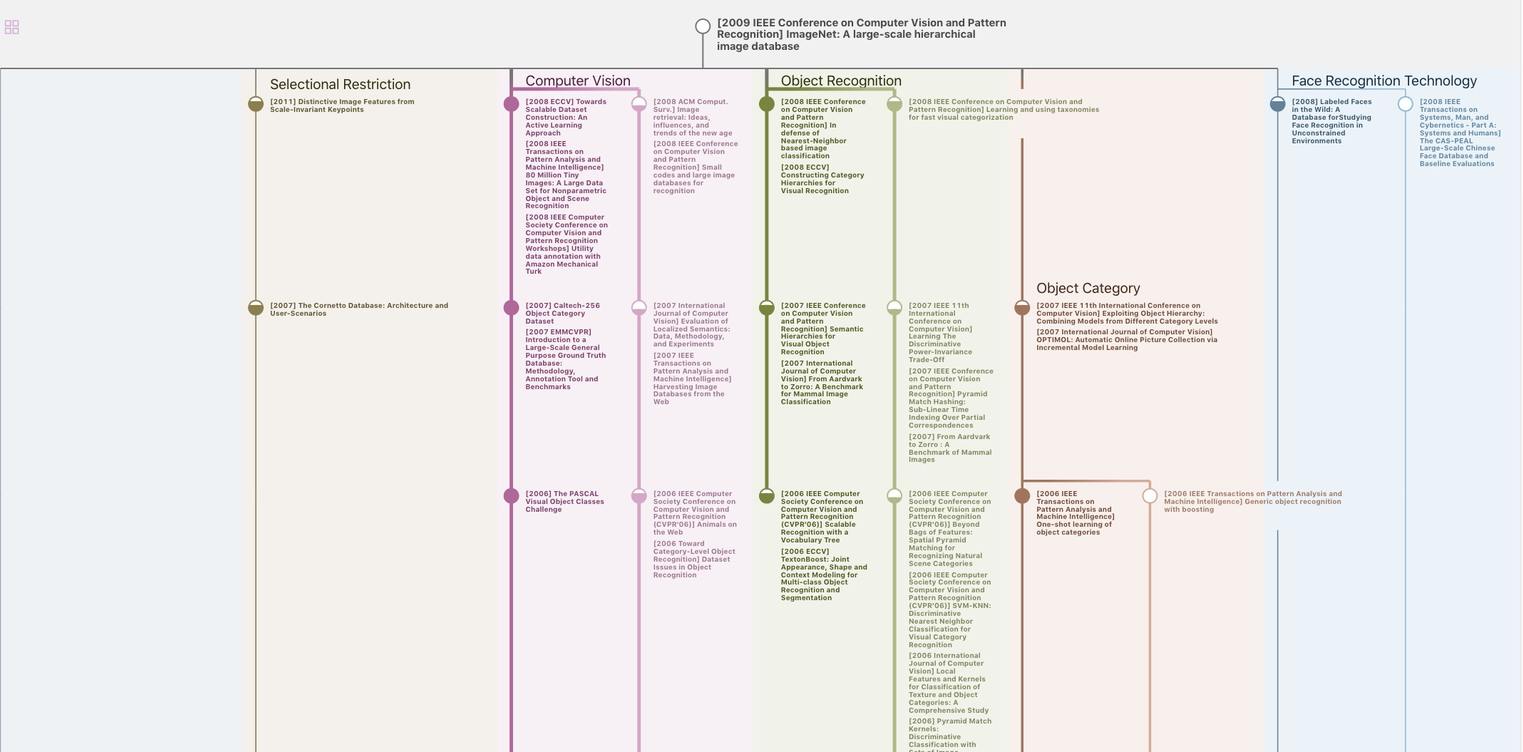
生成溯源树,研究论文发展脉络
Chat Paper
正在生成论文摘要