Self-Supervised Generative Models for Crystal Structures
arxiv(2023)
摘要
Drawing inspiration from the achievements of natural language processing, we
adopt self-supervised learning and utilize an equivariant graph neural network
to develop a unified platform designed for training generative models capable
of generating crystal structures, as well as efficiently adapting to downstream
tasks in material property prediction. To mitigate the challenge of
incorporating large-scale assessment on the reliability of generated structures
into the training process, we utilize the generative adversarial network (GAN)
with its discriminator being a cost-effective evaluator for the generated
structures, resulting in notable improvements in model performance. We
demonstrate the utility of our model in finding the optimal crystal structure
under predefined conditions. Without reliance on properties acquired
experimentally or numerically, our model further displays its capability to
comprehend the mechanism of crystal structure formation through its ability to
grouping chemically similar elements. Therefore, this paper extends an
invitation to explore deeper into the scientific understanding of material
structures through generative models, offering a fresh perspective on
broadening the scope and efficacy of machine learning in material science.
更多查看译文
AI 理解论文
溯源树
样例
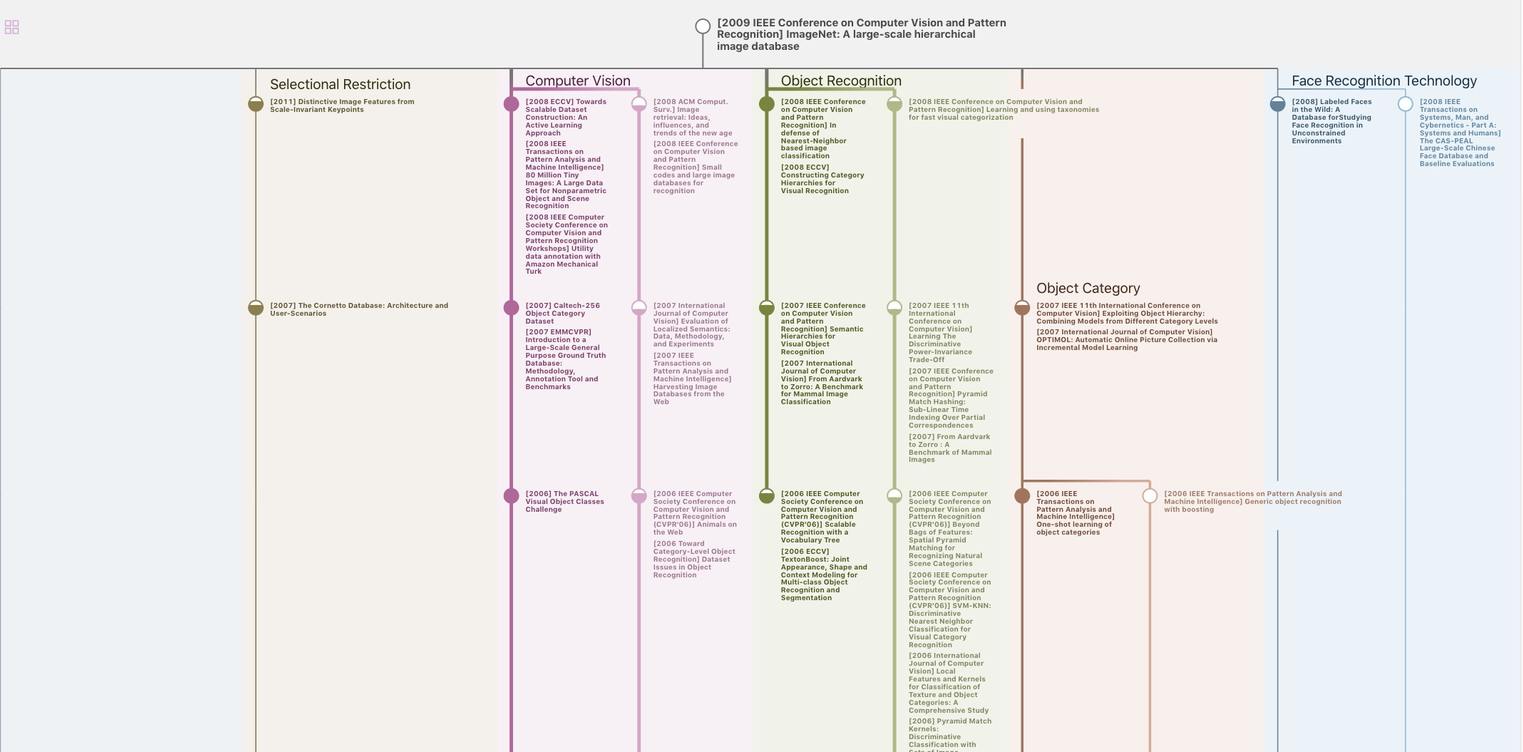
生成溯源树,研究论文发展脉络
Chat Paper
正在生成论文摘要