AI-Based Risk Score from Tumour-Infiltrating Lymphocyte Predicts Locoregional-Free Survival in Nasopharyngeal Carcinoma
CANCERS(2023)
摘要
Background: Locoregional recurrence of nasopharyngeal carcinoma (NPC) occurs in 10% to 50% of cases following primary treatment. However, the current main prognostic markers for NPC, both stage and plasma Epstein-Barr virus DNA, are not sensitive to locoregional recurrence. Methods: We gathered 385 whole-slide images (WSIs) from haematoxylin and eosin (H&E)-stained NPC sections (n = 367 cases), which were collected from Sun Yat-sen University Cancer Centre. We developed a deep learning algorithm to detect tumour nuclei and lymphocyte nuclei in WSIs, followed by density-based clustering to quantify the tumour-infiltrating lymphocytes (TILs) into 12 scores. The Random Survival Forest model was then trained on the TILs to generate risk score. Results: Based on Kaplan-Meier analysis, the proposed methods were able to stratify low- and high-risk NPC cases in a validation set of locoregional recurrence with a statically significant result (p < 0.001). This finding was also found in distant metastasis-free survival (p < 0.001), progression-free survival (p < 0.001), and regional recurrence-free survival (p < 0.05). Furthermore, in both univariate analysis (HR: 1.58, CI: 1.13-2.19, p < 0.05) and multivariate analysis (HR:1.59, CI: 1.11-2.28, p < 0.05), we also found that our methods demonstrated a strong prognostic value for locoregional recurrence. Conclusion: The proposed novel digital markers could potentially be utilised to assist treatment decisions in cases of NPC.
更多查看译文
关键词
nasopharyngeal carcinoma,tumour-infiltrating lymphocytes,locoregional recurrence,computational pathology,artificial intelligence
AI 理解论文
溯源树
样例
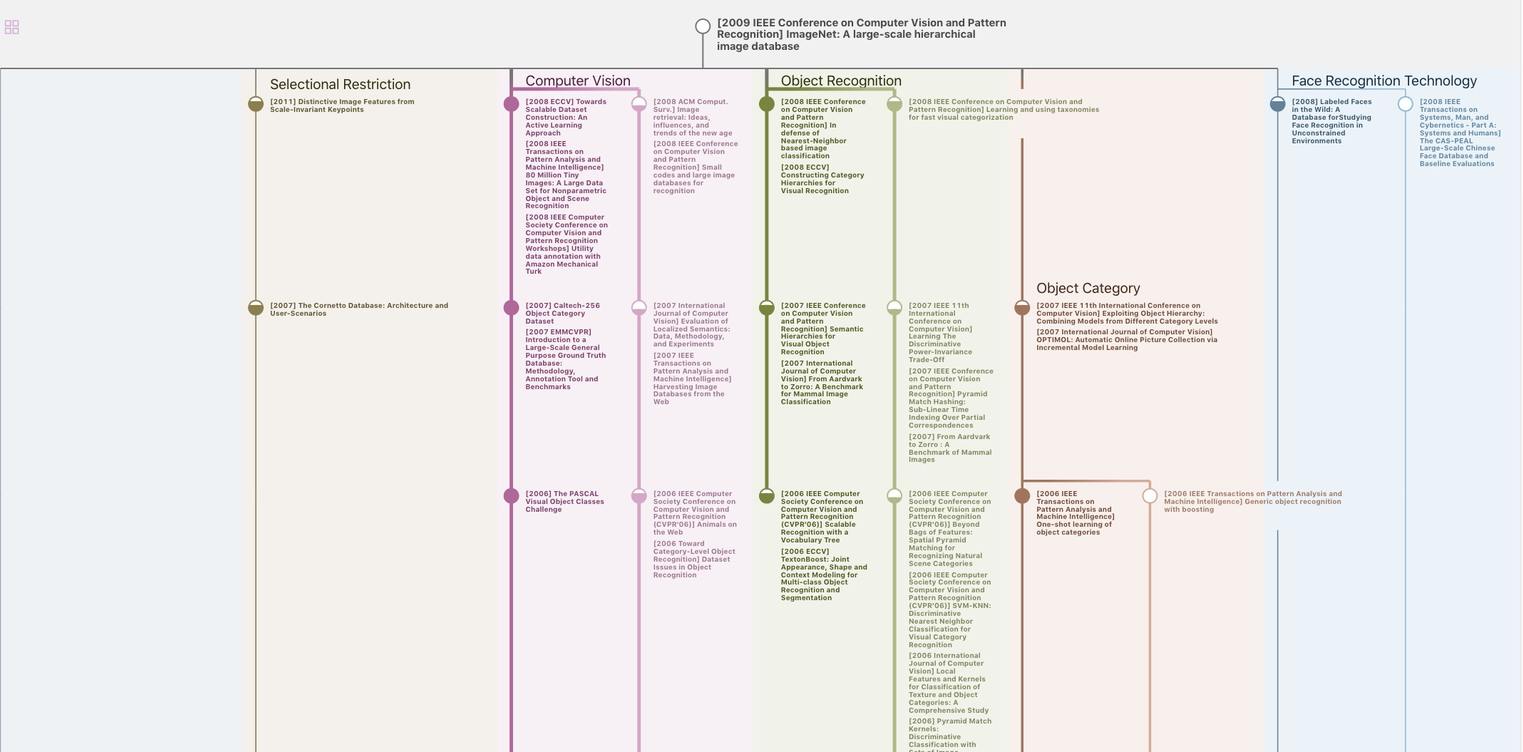
生成溯源树,研究论文发展脉络
Chat Paper
正在生成论文摘要