Robust Multiobjective Particle Swarm Optimization With Feedback Compensation Strategy
IEEE TRANSACTIONS ON CYBERNETICS(2024)
摘要
Multiobjective particle swarm optimization (MOPSO) has been proven effective in solving multiobjective problems (MOPs), in which the evolutionary parameters and leaders are selected randomly to develop the diversity. However, the randomness would cause the evolutionary process uncertainty, which deteriorates the optimization performance. To address this issue, a robust MOPSO with feedback compensation (RMOPSO-FC) is proposed. RMOPSO-FC provides a novel closed-loop optimization framework to reduce the negative influence of uncertainty. First, Gaussian process (GP) models are established by dynamically updated archives to obtain the posterior distribution of particles. Then, the feedback information of particle evolution can be collected. Second, an intergenerational binary metric is designed based on the feedback information to evaluate the evolutionary potential of particles. Then, the particles with negative evolutionary directions can be identified. Third, a compensation mechanism is presented to correct the negative evolution of particles by modifying the particle update paradigm. Then, the compensated particles can maintain the positive exploration toward the true PF. Finally, the comparative simulation results illustrate that the proposed RMOPSO-FC can provide superior search capability of PFs and algorithmic robustness over multiple runs.
更多查看译文
关键词
Uncertainty,Optimization,Particle swarm optimization,Convergence,Statistics,Sociology,History,Evolutionary process uncertainty,feedback compensation (FC),Gaussian processes (GPs),multiobjective particle swarm optimization (MOPSO)
AI 理解论文
溯源树
样例
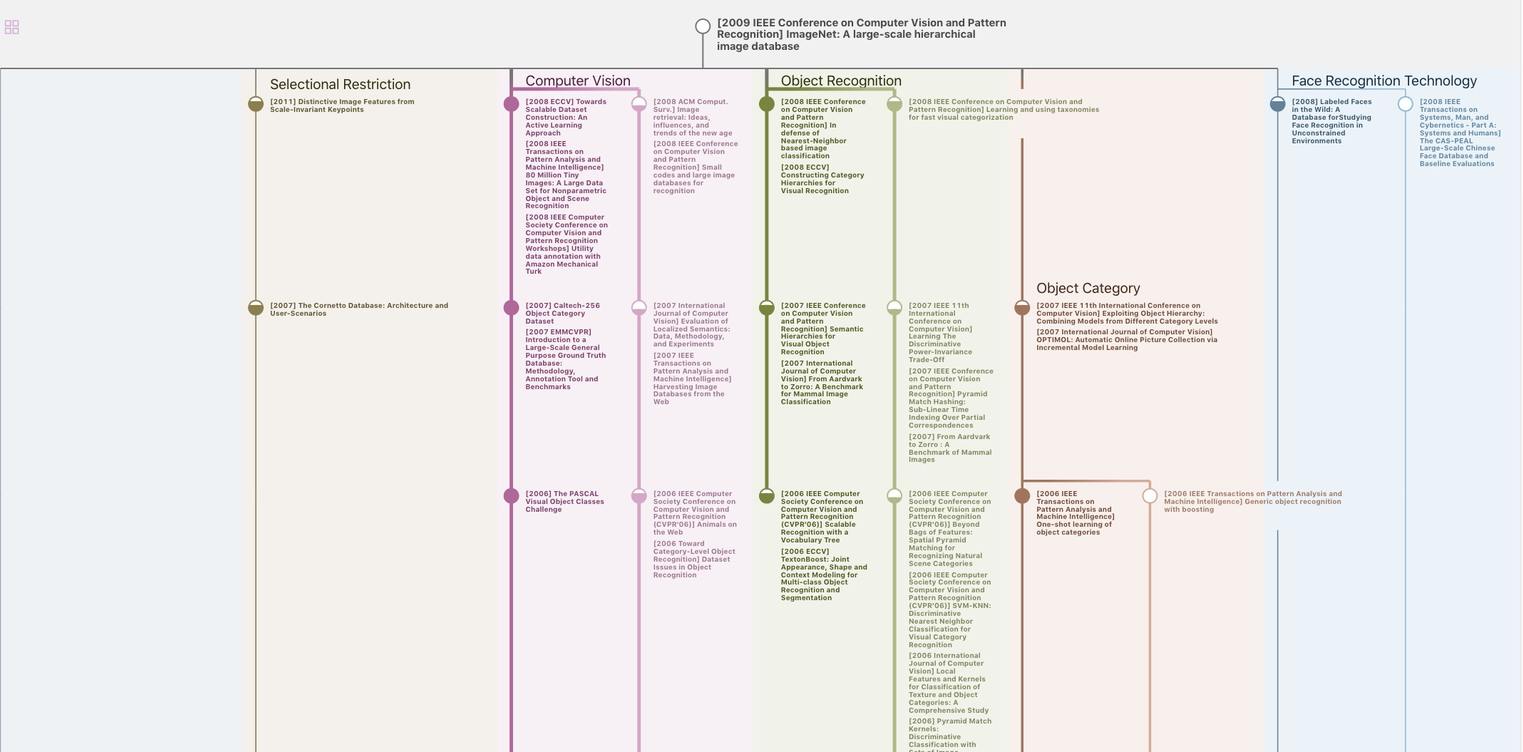
生成溯源树,研究论文发展脉络
Chat Paper
正在生成论文摘要