Two-stage feature distribution rectification for few-shot point cloud semantic segmentation
PATTERN RECOGNITION LETTERS(2024)
摘要
Few-shot point cloud semantic segmentation segments new classes given few labeled examples and has attracted much attention recently. However, due to the scarcity of labeled data, there are biases between the ideal and the actual feature distributions. Addressing the above issues, we propose a two-stage feature distribution rectification method (TFDR) to reduce these biases. We define the biases in two aspects: interclass and intraclass distribution biases. Interclass distribution bias refers to the distribution shifting introduced by the difference between support data and query data. To reduce this bias, we design a novel feature alignment module (FAM). Intraclass distribution bias is defined as the bias between the ideal and the actual feature distribution of a class, which is introduced by the difference in local structures such as the seats and the legs of chairs. To mitigate the effects of intraclass distribution, we propose a distribution canonicalization module (DCM) rectifying the feature distributions of query data. The experimental results show that the proposed method outperforms several state-of-the-art methods with great significance on the S3DIS and ScanNet datasets, thus demonstrating the effectiveness of our model.
更多查看译文
关键词
Few-shot learning,Point cloud semantic segmentation,Feature distribution rectification
AI 理解论文
溯源树
样例
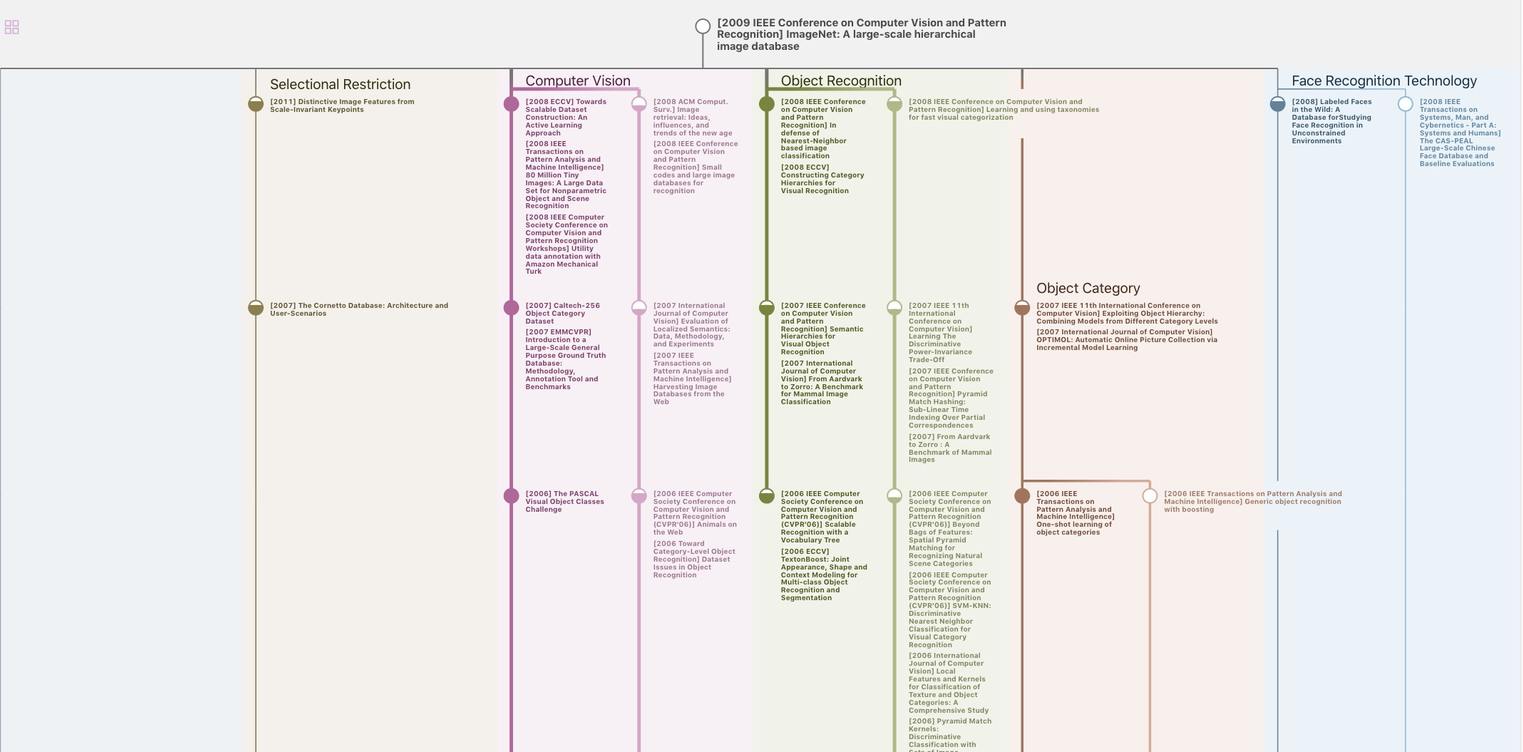
生成溯源树,研究论文发展脉络
Chat Paper
正在生成论文摘要