BioDynGrap: Biomedical event prediction via interpretable learning framework for heterogeneous dynamic graphs
EXPERT SYSTEMS WITH APPLICATIONS(2024)
摘要
Model dynamic graphs with entity-specific heterogeneity has achieved remarkable success across various domains. By utilizing a novel dynamic graph encoding mechanism that employs rich temporal reasoning, we introduce BioDynGraph - a versatile and innovative framework for biomedical event prediction that bridges the gap between historical search and future reasoning. Existing studies tend to overlook clinical relationships between diseases during a patient's visit by treating diagnoses as independent illnesses. Many machine learning techniques assume that disease progression within each time period is static and stationary, attenuating the information of all previous time steps using a homogeneous approach. However, in practice, disease progression is non-stationary, necessitating better use of combinational disease information to learn more about the dynamics of diseases. To address this problem, we use event clue searching and event temporal reasoning infuse entity and relation information into dynamic subgraphs efficiently. BioDynGraph learns a global disease co-occurrence graph through event clue searching and acquires dynamic subgraphs for each patient's visit using event temporal reasoning. Experimental results demonstrate that BioDynGraph exhibits superior performance compared to state-of-the-art (SOTA) baseline methods on biomedical event prediction using three electronic medical records datasets. Additionally, the clues found by BioDynGraph provide interpretability of the event prediction, showcasing its potential as an effective general-purpose interpretability algorithm for biomedical graph data.
更多查看译文
关键词
Biomedical event prediction,Heterogeneous dynamic graphs,Interpretable learning framework,Biomedical knowledge-aware,Knowledge graph
AI 理解论文
溯源树
样例
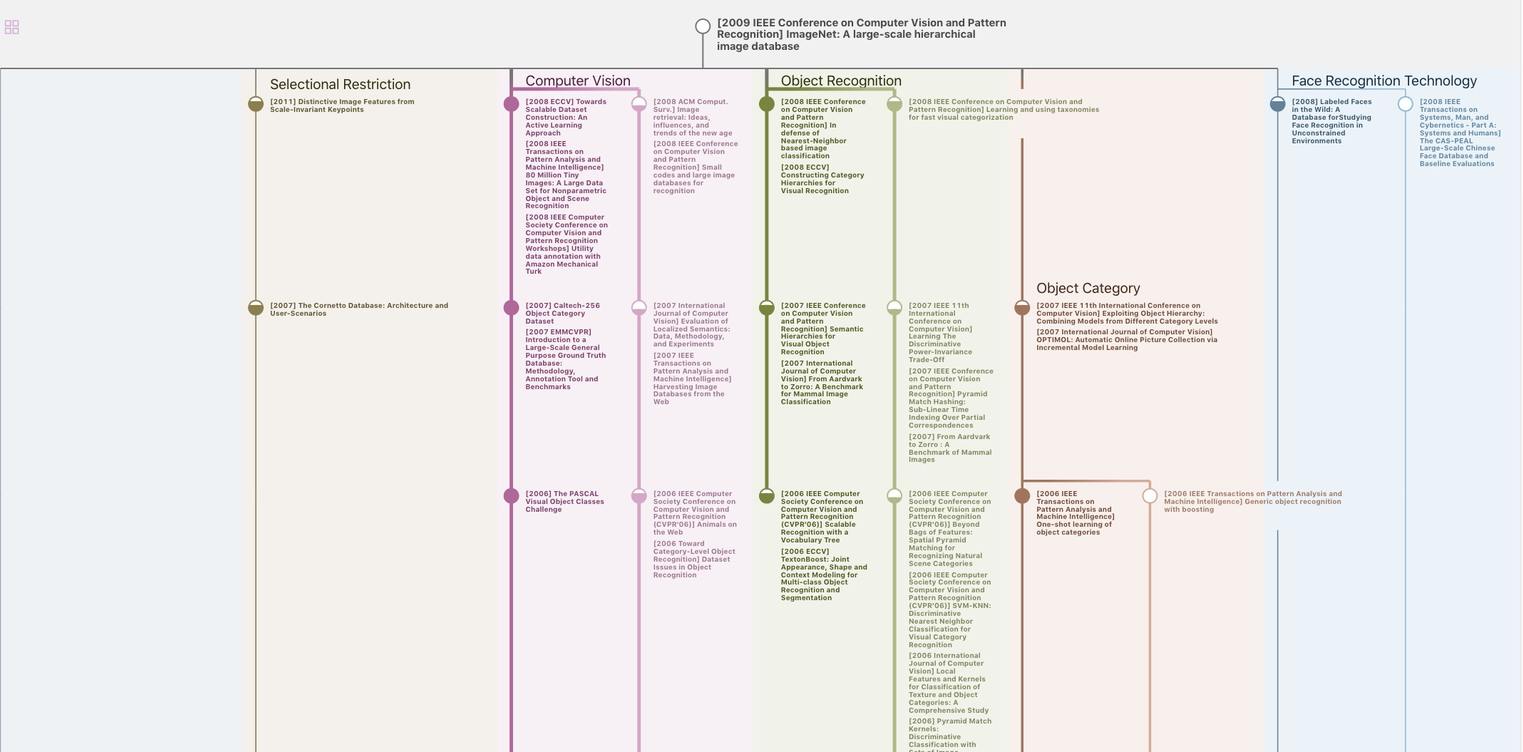
生成溯源树,研究论文发展脉络
Chat Paper
正在生成论文摘要