Enhanced ML-Based Approach for Functional Safety Improvement in Automotive AMS Circuits.
2023 IEEE International Test Conference (ITC)(2023)
摘要
The extensive adoption of safety-critical applications in high-assurance environments, such as the automotive domain, has laid emphasis on safeguarding the reliability and Functional Safety (FuSa) of the Electrical and/or Electronic (E/E) components constituting such systems. Most modern automotive Systems-on-Chips (SoCs) comprise Analog and Mixed Signal (AMS) circuits, which are more susceptible to faults than their digital equivalents. However, their attributes of operating in the continuous signal region can be leveraged to perform early anomaly detection, which could facilitate the subversion of the eventual hardware failure state, thereby improving the FuSa of the system. To this end, we had proposed a novel unsupervised learning-based early anomaly detection framework catered to automotive AMS circuits (in ITC 2022). However, existing approaches to AMS FuSa violation detection are limited by pre-specified feature inputs, and lack rationale for identifying signals to be monitored to perform anomaly detection. To address these issues as well as further augment our original solution, in this paper, we propose a novel anomaly detection strategy that involves: (1) a genetic algorithm-based feature selection approach, (2) a novel signal selection algorithm that ascertains the best intermediate circuit signal, for furnishing enhanced anomaly detection accuracy, while reducing the associated detection latency, and (3) an explainable AI (XAI)-based framework that boosts user interpretability and transparency of the anomaly detection framework. This XAI approach, in turn, can be provided as feedback to the designer during circuit design and validation. The proposed approach is evaluated using case studies of two representative AMS circuits, which are prevalent in automotive SoCs. Our experimental analyses demonstrate that the proposed approach furnishes up to 100% detection accuracy and 2.3× reduction in detection time compared to our existing framework, in addition to providing insights by improving transparency of the anomaly detection framework, thereby exhibiting the efficacy of our solution.
更多查看译文
关键词
Functional Safety,AMS Circuits,Feature Selection,Signal Selection,Explainable AI
AI 理解论文
溯源树
样例
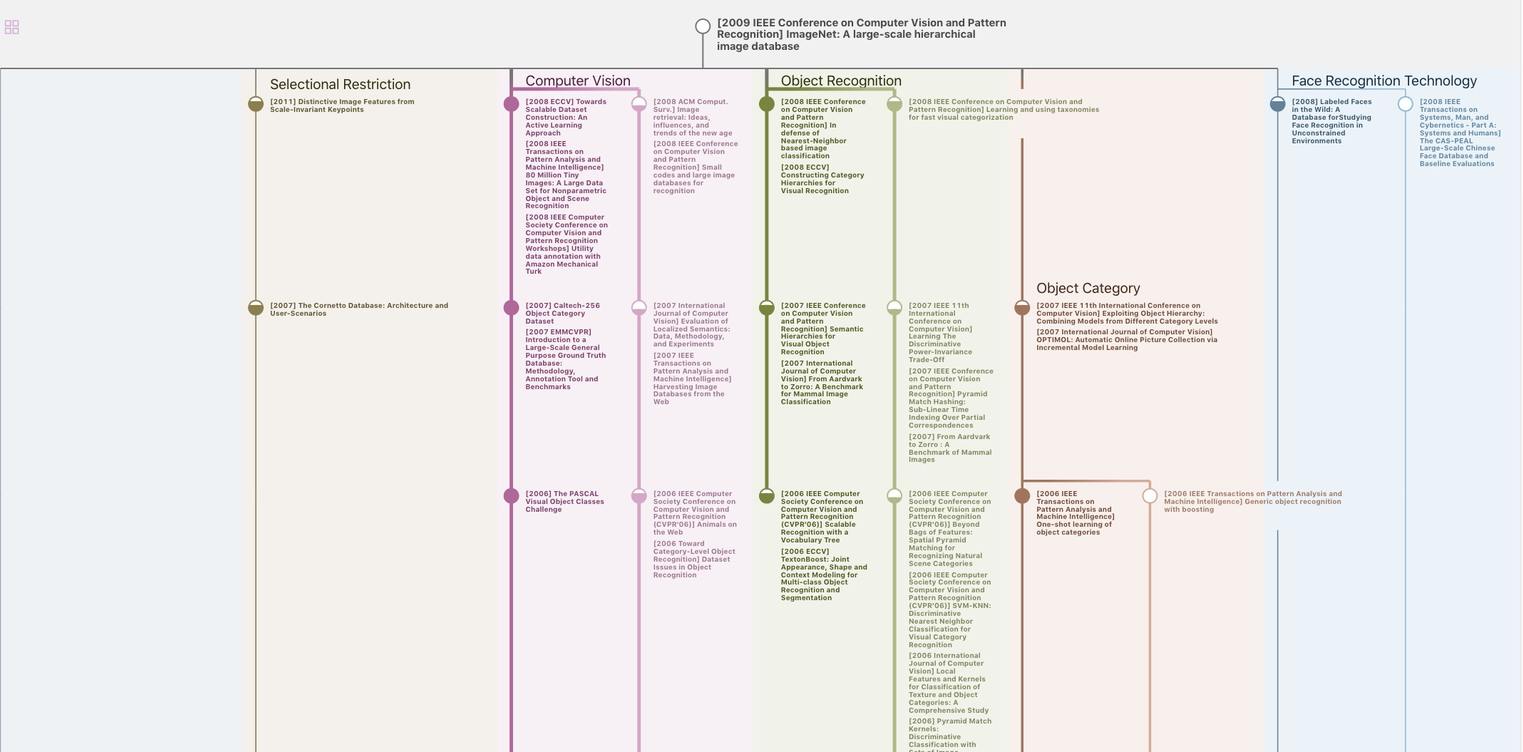
生成溯源树,研究论文发展脉络
Chat Paper
正在生成论文摘要