Model-Driven Optimization for Quantum Program Synthesis with MOMoT
2023 ACM/IEEE INTERNATIONAL CONFERENCE ON MODEL DRIVEN ENGINEERING LANGUAGES AND SYSTEMS COMPANION, MODELS-C(2023)
摘要
In the realm of classical software engineering, model-driven optimization has been widely used for different problems such as (re)modularization of software systems. In this paper, we investigate how techniques from model-driven optimization can be applied in the context of quantum software engineering. In quantum computing, creating executable quantum programs is a highly non-trivial task which requires significant expert knowledge in quantum information theory and linear algebra. Although different approaches for automated quantum program synthesis exist-e.g., based on reinforcement learning and genetic programming-these approaches represent tailor-made solutions requiring dedicated encodings for quantum programs. This paper applies the existing model-driven optimization approach MOMoT to the problem of quantum program synthesis. We present the resulting platform for experimenting with quantum program synthesis and present a concrete demonstration for a well-known quantum algorithm.
更多查看译文
关键词
Quantum Circuit Synthesis,Model-Driven Optimization,Quantum Software Engineering
AI 理解论文
溯源树
样例
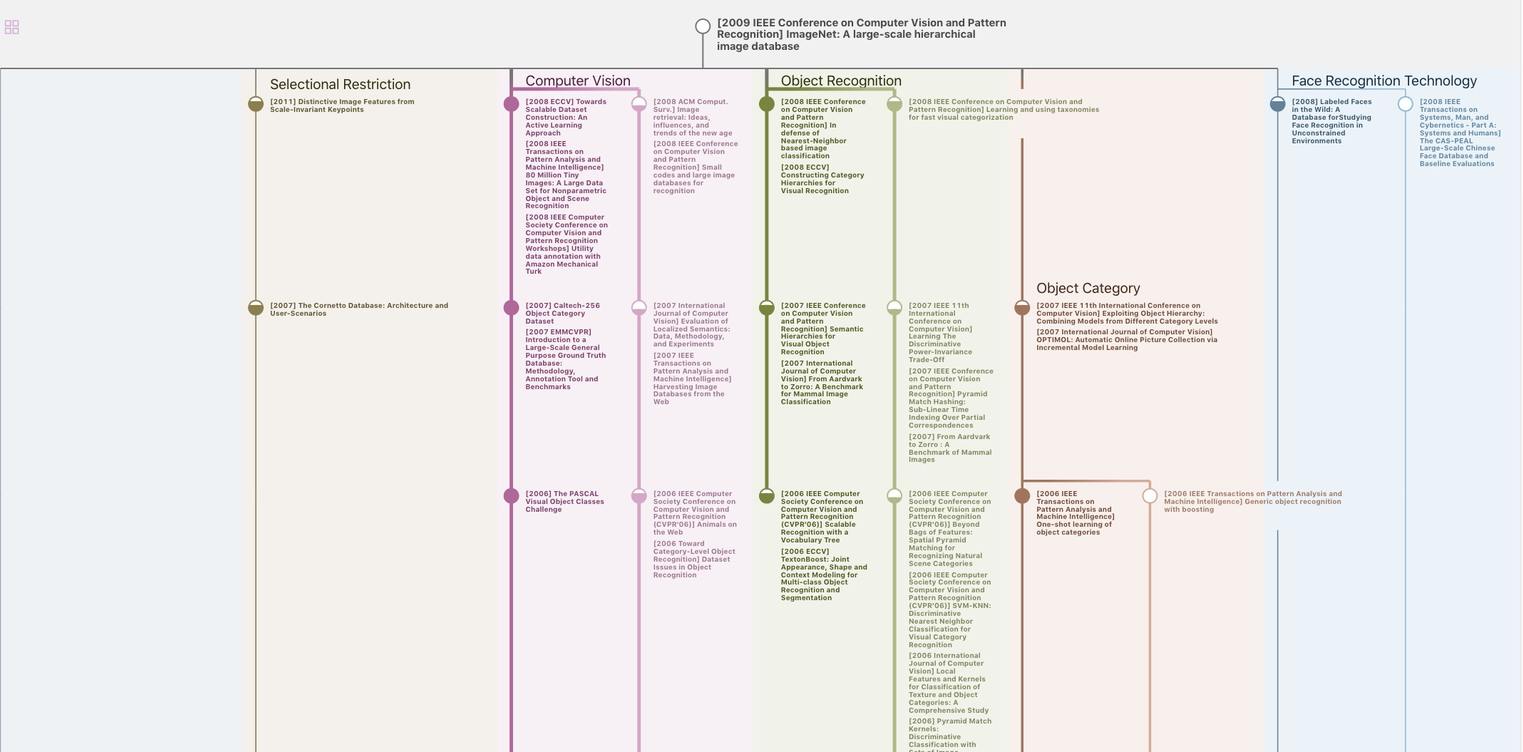
生成溯源树,研究论文发展脉络
Chat Paper
正在生成论文摘要