A Node Deployment-Aided Intelligent Optimization Estimation for WSNs Positioning Refinement
IEEE TRANSACTIONS ON INSTRUMENTATION AND MEASUREMENT(2024)
摘要
The popularity of next-generation networks has brought ubiquitous location-based demands. This article proposes a node deployment-aided intelligent optimization estimation (NDIOE) for target positioning in terms of node deployment, wireless ranging, calibration errors, and the algorithm itself. Since the optimized deployment of anchor nodes is the basis of high-accuracy positioning, we derive the characteristic matrix closely related to the upper bound of positioning error and map the signal domain to the location domain for a preliminary result solved by unconstrained optimization estimation. On this basis, the preliminary locations as the initial search values can be further refined for more accurate locations with the use of improved local particle swarm optimization in a narrowed searching area, whose adaptive fitness function, inertia weight factor, and mutation operation are designed to avoid falling into the local optimal. Aside from that, considering the high computational cost of solving extreme values through node traversal, we theoretically derive the theoretical minimum expression of any point based on Cramer-Rao lower bound (CRLB) as a benchmark, whose theoretical minimum value is 0.22 in the fully connected network. Compared with the existing related algorithms, the proposed NDIOE algorithm is effectively validated under simulation and experimental platforms, as well as in complex environments with obstacles.
更多查看译文
关键词
Accuracy evaluation,Cramer-Rao lower bound (CRLB),distributed positioning,intelligent optimization,node deployment,target localization,uncertainties,wireless sensor networks (WSNs)
AI 理解论文
溯源树
样例
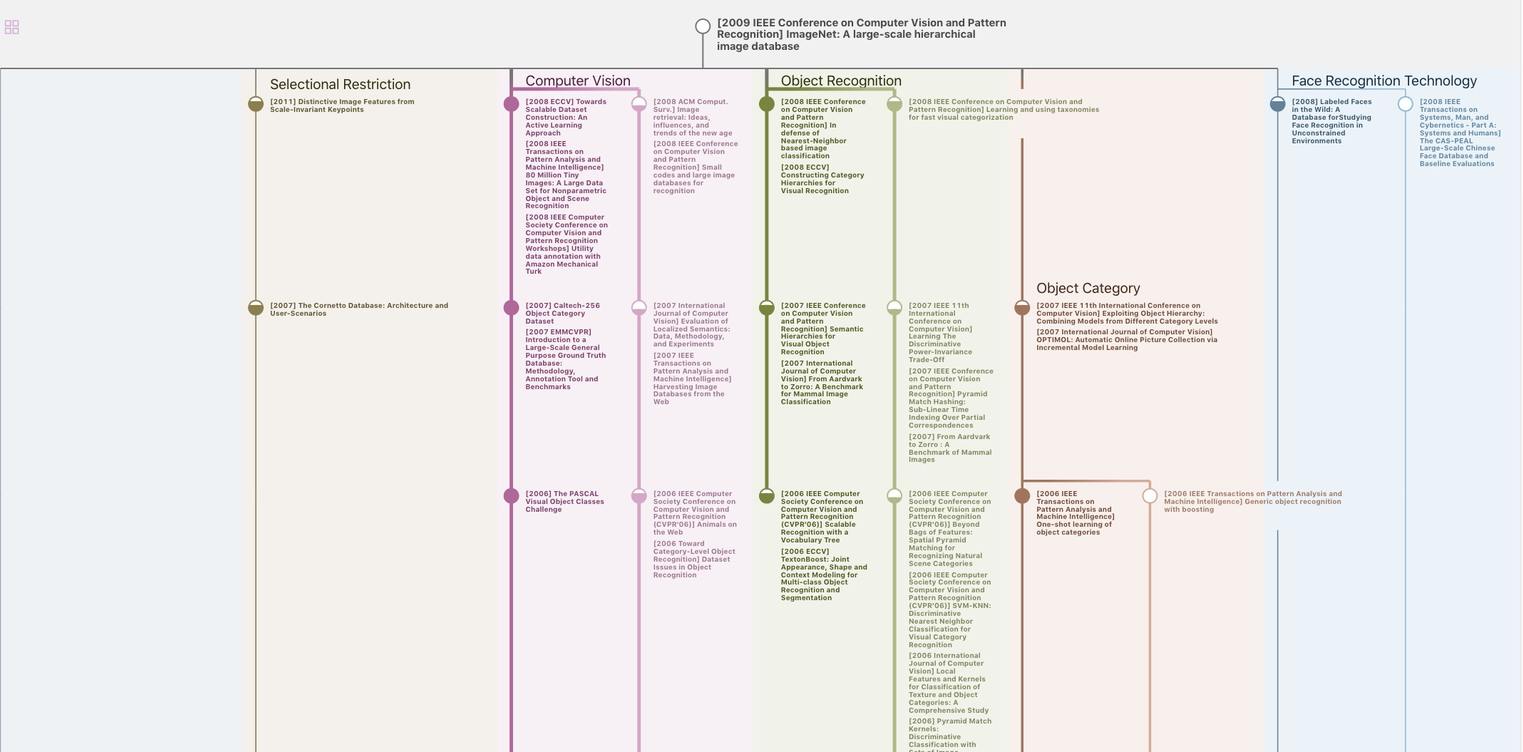
生成溯源树,研究论文发展脉络
Chat Paper
正在生成论文摘要