Sample Point Classification of Abdominal ECG Through CNN-Transformer Model Enables Efficient Fetal Heart Rate Detection
IEEE TRANSACTIONS ON INSTRUMENTATION AND MEASUREMENT(2024)
摘要
Monitoring fetal heart rate (FHR) is essential for the early detection of fetal distress and ensuring safe delivery. Direct invasive fetal electrocardiography (FECG) provides reliable FHR signals but is limited to use during labor. Noninvasive fetal heart monitoring can be achieved through abdominal electrocardiography (AECG), where fetal heartbeat waveforms are captured from electrodes placed on maternal abdomen. However, accurately locating fetal R-peaks and obtaining FHRs remain challenging due to interference from uterine contractions and maternal heartbeats. To address this challenge, we proposed an end-to-end fetal R-peak detection method based on sample point classification. Inspired by semantic segmentation in image analysis, we utilized an encoder-decoder model to classify each point in the 1-D AECG signal. The model captures the global contextual information of fetal heartbeat waveforms by combining convolutional neural networks (CNNs) and transformer, resulting in a high-precision classification of each sample point in the AECG signal. Moreover, to mitigate the impact of misclassified points on fetal R-peak detection, we proposed a postprocessing method based on the periodicity of heartbeats. The effectiveness of the proposed method is validated on two AECG databases, achieving satisfactory results with an average F1-score of 98.91%. These findings demonstrate the potential application of long-term fetal monitoring using portable devices. The code is publicly available at https://github.com/ZJUT-CBS/FR-Net.
更多查看译文
关键词
Abdominal ECG (AECG),fetal heart rate (FHR),fetal R-peak detection,sample point classification
AI 理解论文
溯源树
样例
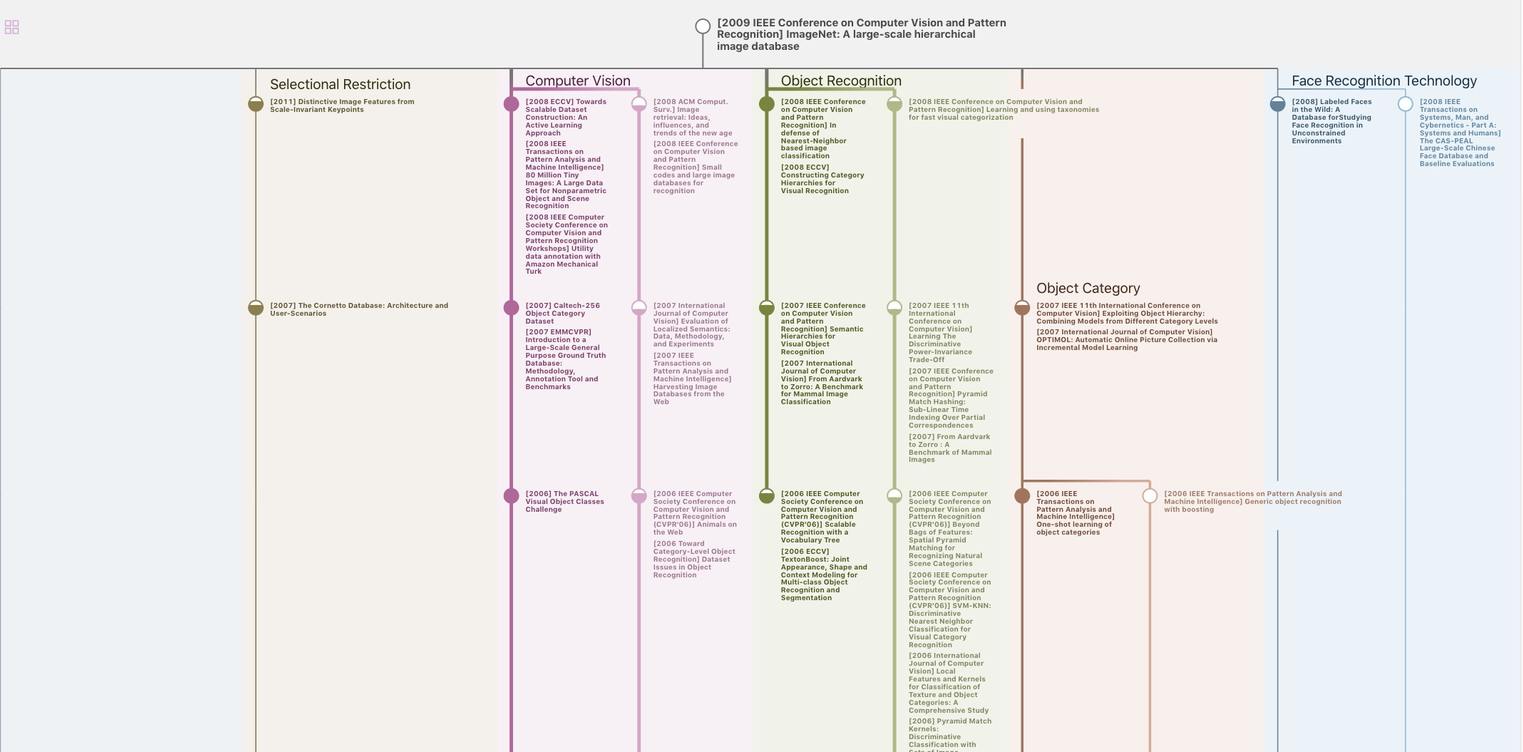
生成溯源树,研究论文发展脉络
Chat Paper
正在生成论文摘要