Achieving the Defect Transfer Detection of Semiconductor Wafer by a Novel Prototype Learning-Based Semantic Segmentation Network
IEEE TRANSACTIONS ON INSTRUMENTATION AND MEASUREMENT(2024)
摘要
Recently, deep-learning-based methods have achieved excellent performance in semiconductor wafer defect inspection. However, these trained networks are only effective for a certain background pattern and fail in defect transfer detection between multiple background patterns. Once the background pattern has changed, images are needed to be recollected and relabeled, which is very time-consuming. To achieve defect transfer detection in semiconductor wafers, a novel prototype learning (PL)-based network is proposed in this article. First, a multiscale residual fusion module (MSRFM) is elaborately designed to generate feature maps that minimize the interference of background patterns. Second, some labeled defect images are chosen as a defect library, which is further extracted into a defect prototype. Then, a distance map will be calculated between feature maps and the defect prototype, resulting in a precise prediction. Finally, an extra self-constraint loss (L-sc) is designed to regulate the defect prototype calculation process. Different from previous methods, only defect-free images are required for the proposed method to achieve defect transfer detection. Experiments on real-world semiconductor wafer production lines show that the proposed method achieves mean intersection over union (mIoU) of 83.49% and 80.12% in defect transfer detection between two background pattern wafers. Furthermore, the excellent performance on other classical industrial datasets demonstrates that the proposed network has great robustness to various defects and industrial scenarios.
更多查看译文
关键词
Defect transfer defection,multiscale residual fusion,prototype learning (PL),semantic segmentation,wafer surface defect detection
AI 理解论文
溯源树
样例
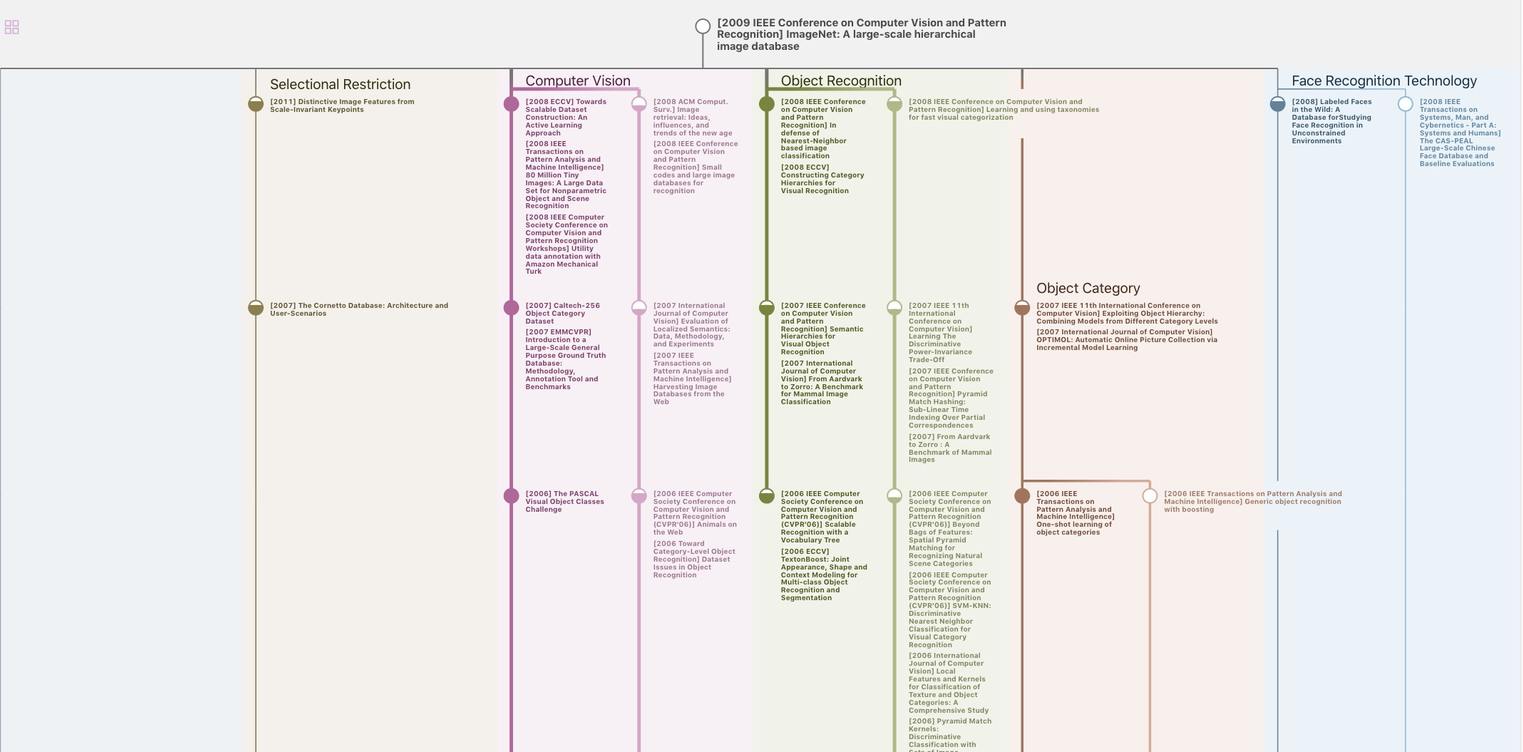
生成溯源树,研究论文发展脉络
Chat Paper
正在生成论文摘要