Dual Contrastive Training and Transferability-Aware Adaptation for Multisource Privacy-Preserving Motor Imagery Classification
IEEE TRANSACTIONS ON INSTRUMENTATION AND MEASUREMENT(2024)
摘要
Motor imagery (MI) is one of the brain-computer interface (BCI) paradigms that allows a participant to mentally imagine the movement execution without moving physically by electroencephalogram (EEG). The MI signals vary dramatically among multiple subjects, which makes the MI classification task extremely challenging, because the model trained on one subject may totally fail on another one. Furthermore, privacy concerns always arise as the EEG contains sensitive health and mental information. In this article, we propose an unsupervised multisource-free domain adaptation (DA) algorithm to reduce the discrepancy between the individual MI signals and protect individual privacy simultaneously. Specifically, in the source training phase, we fully leverage the labels of the source data in an instance-contrast fashion guided by a contrastive cross-entropy loss and learn the intrinsic structure inside the data in a category-consistent way by a categorical contrastive loss. In the target adaptation phase, our model only accesses the parameters of the source models instead of the source data for privacy preservation. To achieve this, we propose to ensemble the source models by linear combination and then theoretically explain why the target model performs better than or equal to the arbitrary source model. We further keep our model's attention to the transferability of the source models by estimating the maximum value of label evidence to prevent noise accumulation when generating pseudo-labels. Sufficient experiments on three datasets with similar properties demonstrate our model outperforms state-of-the-art methods for cross-subject MI classification tasks. Our source code is available at https://github.com/grilled-chicken-burger/bci for noncommercial use.
更多查看译文
关键词
Domain discrepancy,motor imagery (MI),multisource-free domain adaptation (DA),privacy preservation
AI 理解论文
溯源树
样例
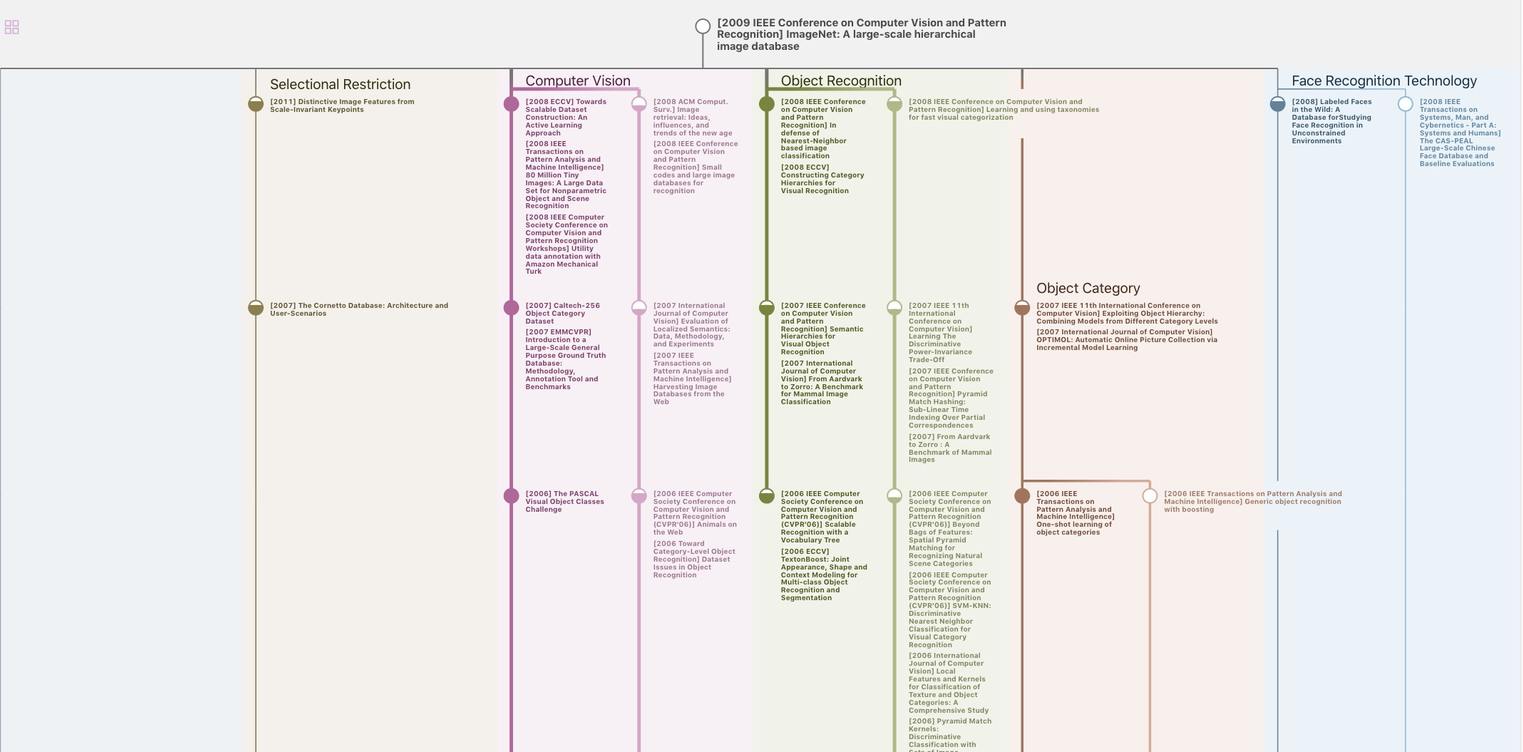
生成溯源树,研究论文发展脉络
Chat Paper
正在生成论文摘要