Adaptive Reconvergence-driven AIG Rewriting via Strategy Learning
2023 IEEE 41ST INTERNATIONAL CONFERENCE ON COMPUTER DESIGN, ICCD(2023)
摘要
Rewriting is a common procedure in logic synthesis aimed at improving the performance, power, and area (PPA) of circuits. The traditional reconvergence-driven And-Inverter Graph (AIG) rewriting method focuses solely on optimizing the reconvergence cone through Boolean algebra minimization. However, there exist opportunities to incorporate other node-rewriting algorithms that are better suited for specific cones. In this paper, we propose an adaptive reconvergence-driven AIG rewriting algorithm that combines two key techniques: multi-strategy-based AIG rewriting and strategy learning-based algorithm selection. The multi-strategy-based rewriting method expands upon the traditional approach by incorporating support for multi-node-rewriting algorithms, thus expanding the optimization space. Additionally, the strategy learning-based algorithm selection method determines the most suitable node-rewriting algorithm for a given cone. Experimental results demonstrate that our proposed method yields a significant average improvement of 5.567% in size and 5.327% in depth.
更多查看译文
关键词
Rewriting,Reconvergence,Multi-strategy,Learning
AI 理解论文
溯源树
样例
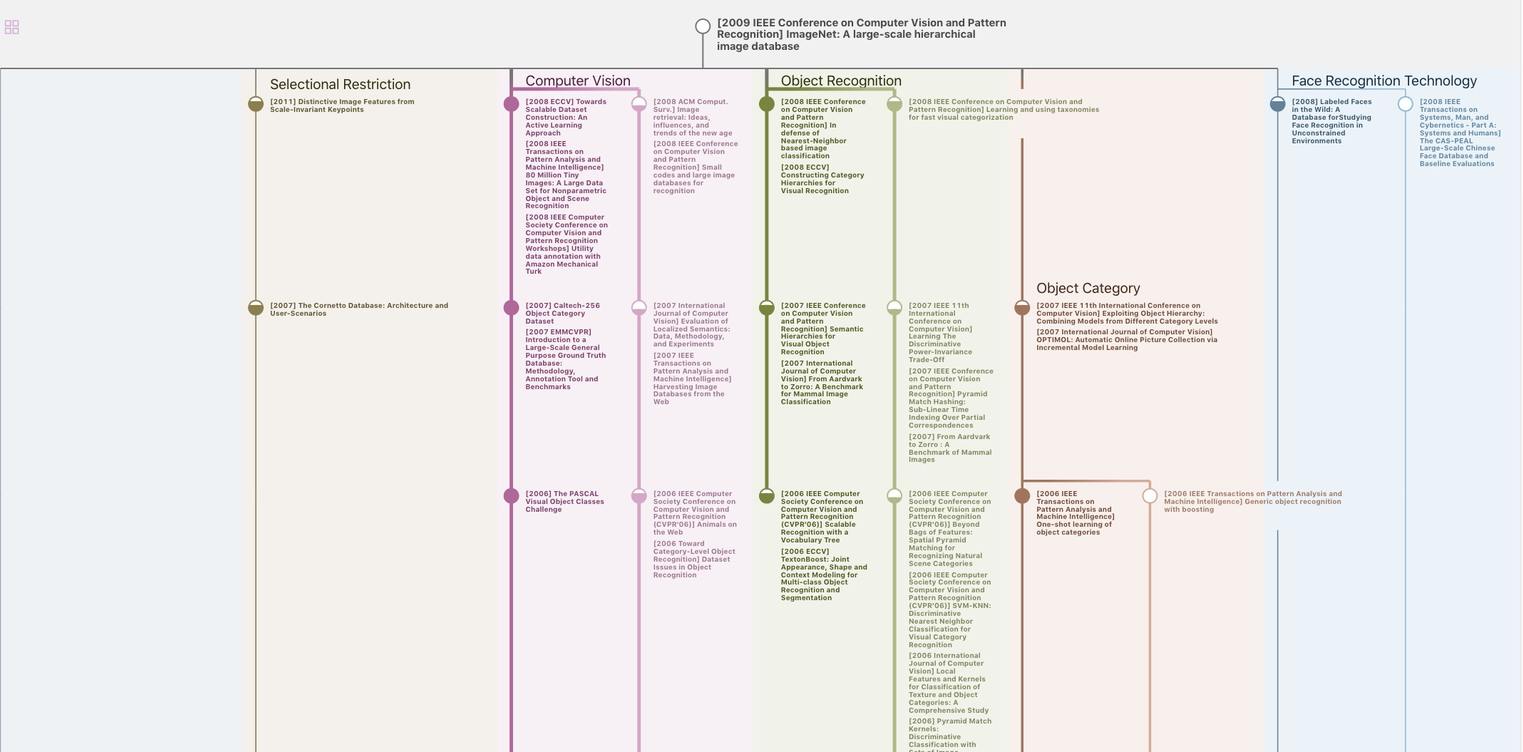
生成溯源树,研究论文发展脉络
Chat Paper
正在生成论文摘要