Inductive and adaptive graph convolution networks equipped with constraint task for spatial-temporal traffic data kriging
KNOWLEDGE-BASED SYSTEMS(2024)
摘要
In intelligent transportation systems (ITS), deploying fine-grained sensors to continuously collect spatial- temporal traffic data is important but impractical due to the expensive cost. Fortunately, spatial-temporal kriging methods bring advanced solutions for interpolating traffic data for locations without sensors, but they still have the following two drawbacks: (1) The widely adopted predefined and adaptive graphs are either inflexible or limited to transductive learning. (2) The sampling strategies to support inductive learning on new graphs result in losing important partial information. To overcome the above issues, in this paper, we propose an Inductive and Adaptive Graph Convolution Networks (IAGCN) for spatial-temporal traffic data kriging in an inductive manner. Specifically, we propose an adaptive graph constructor to model the hidden spatial relation of nodes and learn the spatial dependency that the predefined graph structure cannot capture. It can work inductively and does not require retraining when introducing new nodes. Additionally, we design a framework that integrates inductive graph convolution and temporal convolution to simultaneously capture the complex spatial-temporal dependencies of traffic data. Finally, to address the information loss issue caused by random sampling, we design a predicted-based constraint task that perceives and utilizes the history information of all observed sensors to predict the current data, as well as approach the result of the interpolation and prediction. Experiments on four real-world datasets show that IAGCN outperforms the state-of-the-art baselines.
更多查看译文
关键词
Kriging,Spatial-temporal data mining,Graph neural networks
AI 理解论文
溯源树
样例
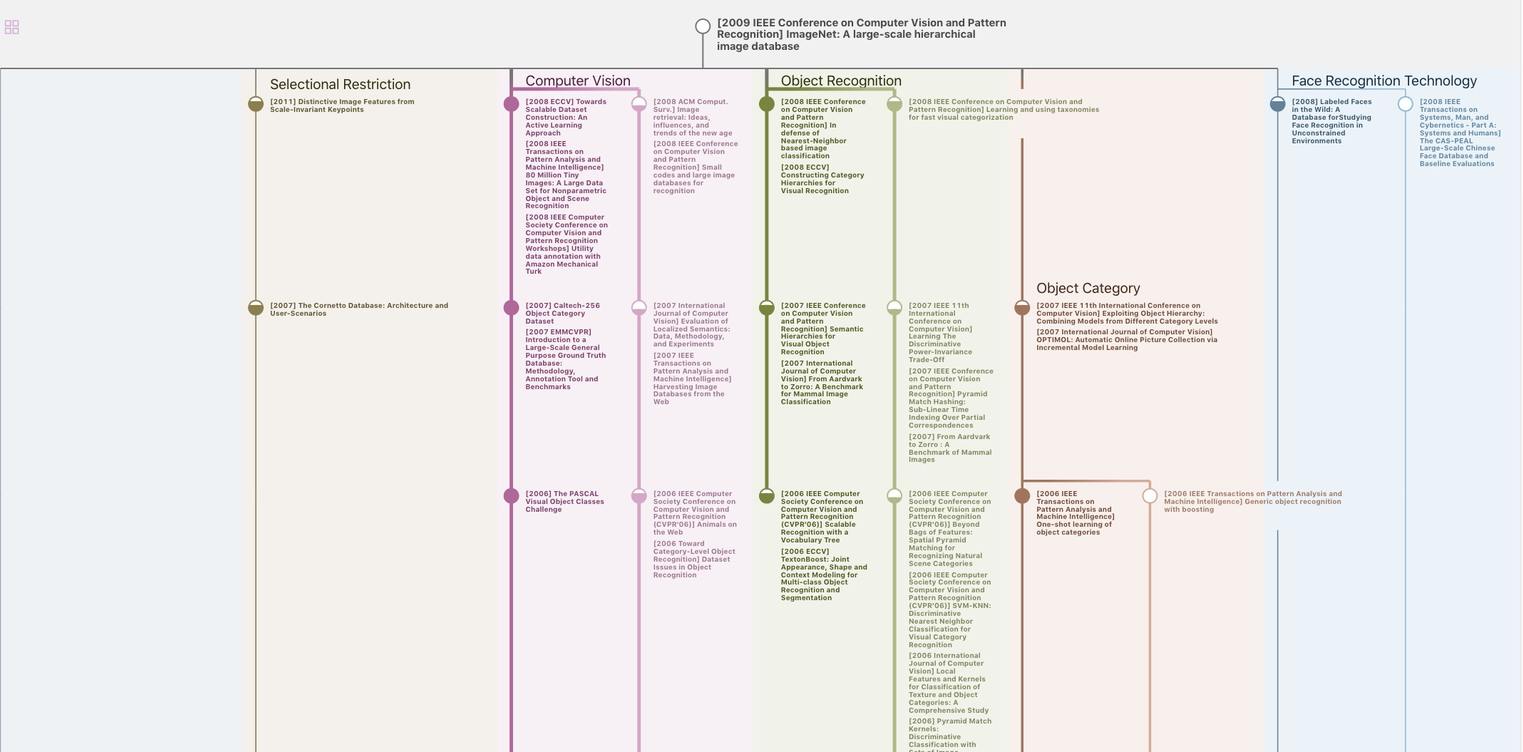
生成溯源树,研究论文发展脉络
Chat Paper
正在生成论文摘要