Doxing, Political Affiliation, and Type of Information: Effects on Suspicion, Perceived Similarity, and Hiring-Related Judgments
JOURNAL OF APPLIED PSYCHOLOGY(2023)
摘要
Researchers have begun to focus on the influence of political affiliation in organizations. In this context, we investigated how doxing (i.e., using social media to post information online with malintent) influences hiring-related decisions. Based on the integration of a political affiliation and state suspicion model, we investigated how a dox containing different types of information (affirming a political party affiliation vs. providing derogatory/negative information about an opposing party) and political party affiliation similarity influenced hiring-related perceptions of job applicants. Given doxing's characteristics, we expanded the "decision space" to include effects about expected organizational image and expected retaliation. In Study 1, we found that the type of information and party similarity influenced suspicion of the applicant and perceived similarity with the applicant, whereas doxing only influenced suspicion. In turn, suspicion and perceived similarity predicted expected task performance and organizational image, and exploratory analyses suggested an interactive effect of these variables. Suspicion also predicted expected retaliation from individuals outside the organization. In Study 2, we confirmed that doxing was related to suspicion as well as the interactive effect of information type and party similarity. We explain that interaction using the notion of symbolic threat. In both studies, the effects of type of information and party similarity were pervasive. Our results support the similarity-attraction paradigm and a model of political affiliation. Expanding relevant theories to include suspicion helps better understand politically related judgments and the additional outcomes of expected organizational image and retaliation.
更多查看译文
关键词
political affiliation,political ideology,doxing,similarity,personnel selection
AI 理解论文
溯源树
样例
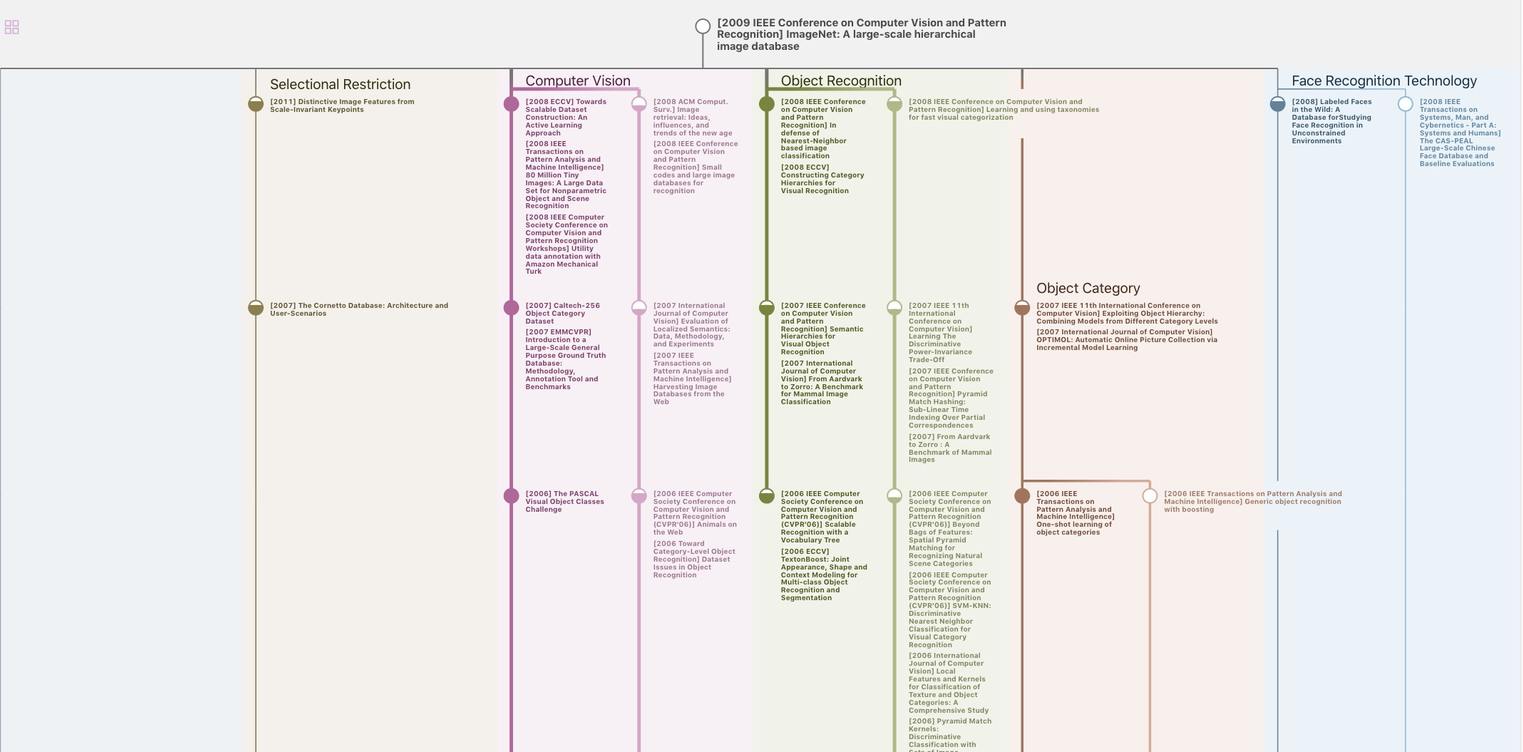
生成溯源树,研究论文发展脉络
Chat Paper
正在生成论文摘要