Subsampling and space-filling metrics to test ensemble size for robustness analysis with a demonstration in the Colorado River Basin
ENVIRONMENTAL MODELLING & SOFTWARE(2024)
摘要
Decision Making Under Deep Uncertainty often uses prohibitively large scenario ensembles to calculate robustness and rank policies' performance. This paper contributes a framework using subsampling algorithms and space-filling metrics to determine how smaller ensemble sizes impact the accuracy of robustness rankings. Subsampling methods create smaller scenario ensembles of varying sizes. We evaluate ranking sensitivity to the ensemble size and calculate accuracy relative to a baseline ranking. Then, metrics of scenario set quality predict ranking accuracy. Notably, the metrics and subsampling methods do not require additional model simulations. We demonstrate the framework with a case study of shortage policies for Lake Mead in the Colorado River Basin (CRB). Results suggest that fewer scenarios than previous studies can accurately rank Lake Mead policies, and results depend on the type of objective and robustness metric. Smaller ensembles could reduce the computational burden of robustness analyses in the ongoing CRB policy renegotiation.
更多查看译文
关键词
Scenarios,Deep uncertainty,States of the world,Robustness,Design of experiments,Colorado river
AI 理解论文
溯源树
样例
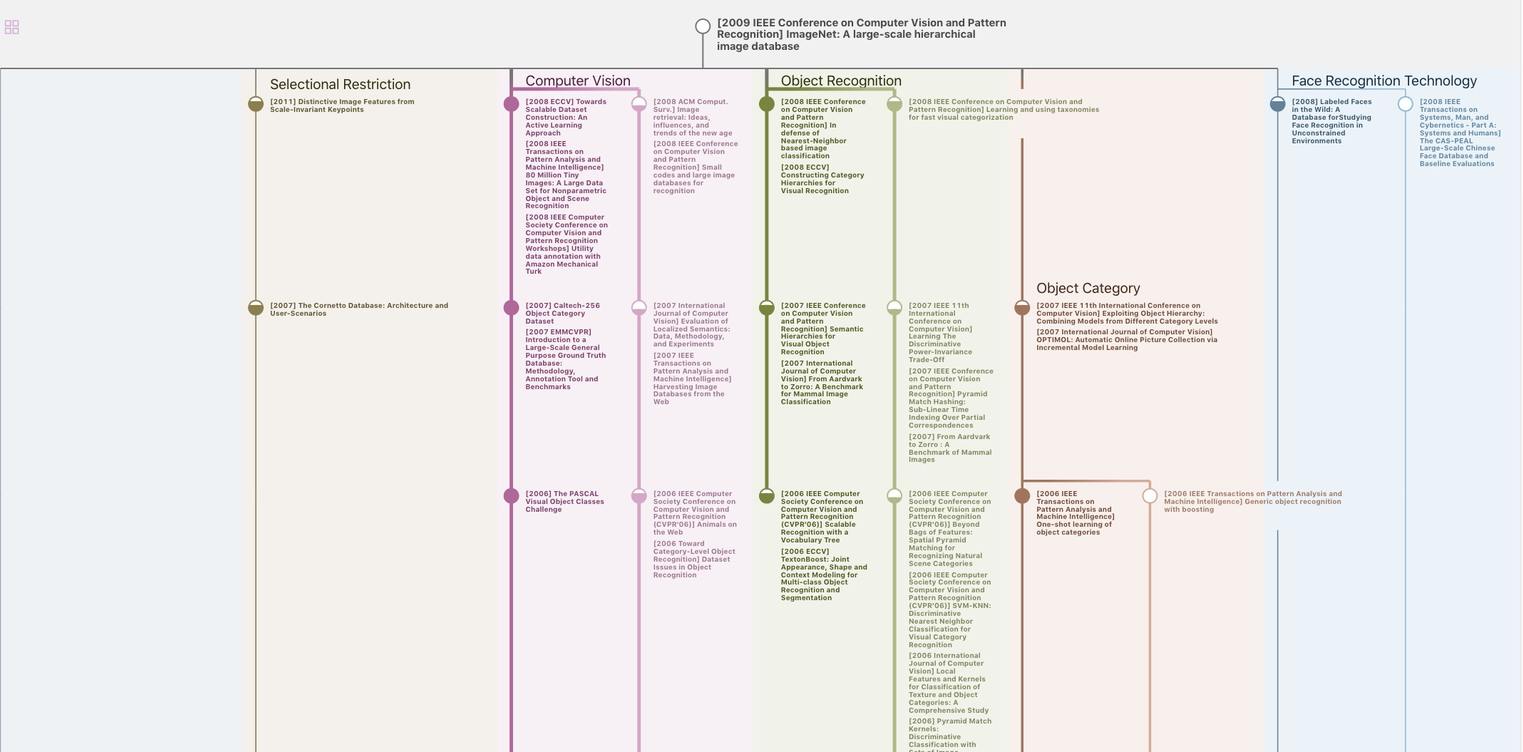
生成溯源树,研究论文发展脉络
Chat Paper
正在生成论文摘要