An adaptive robust service composition and optimal selection method for cloud manufacturing based on the enhanced multi-objective artificial hummingbird algorithm
EXPERT SYSTEMS WITH APPLICATIONS(2024)
摘要
The smooth execution of tasks in the cloud manufacturing (CMfg) process can be significantly affected by various real-world uncertainties, resulting in inefficiencies or even failures of the composite manufacturing service (CMS). Nevertheless, current research primarily concentrates on post-exception emergency handling methods, heavily dependent on the planned CMS and real-time status of manufacturing resources, often leading to suboptimal temporary adjustments or even the inability to find a viable solution. Hence, there is an urgent necessity to enhance the robustness of the planned CMS during the service composition and optimal selection (SCOS) phase. In pursuit of this goal, the paper initially presents an adaptive robust service composition and optimization selection (ARSCOS) model. This model dynamically determines whether to await exception resolution or invoke an alternative service, considering the circumstances when a service exception arises and employing task delay time to characterize the robustness of a specific CMS. Subsequently, an enhanced multi-objective artificial hummingbird algorithm (EMOAHA) is devised for this model. Finally, the efficacy of EMOAHA is demonstrated by applying it to nine different scales of civilian unmanned aerial vehicle manufacturing SCOS problems and 17 benchmark functions. The results illustrate that the proposed EMOAHA exhibits superior convergence and diversity in comparison to the other 11 classical intelligent optimization algorithms.
更多查看译文
关键词
Cloud manufacturing,Service composition,Robustness,Enhanced multi -objective artificial humming,bird algorithm
AI 理解论文
溯源树
样例
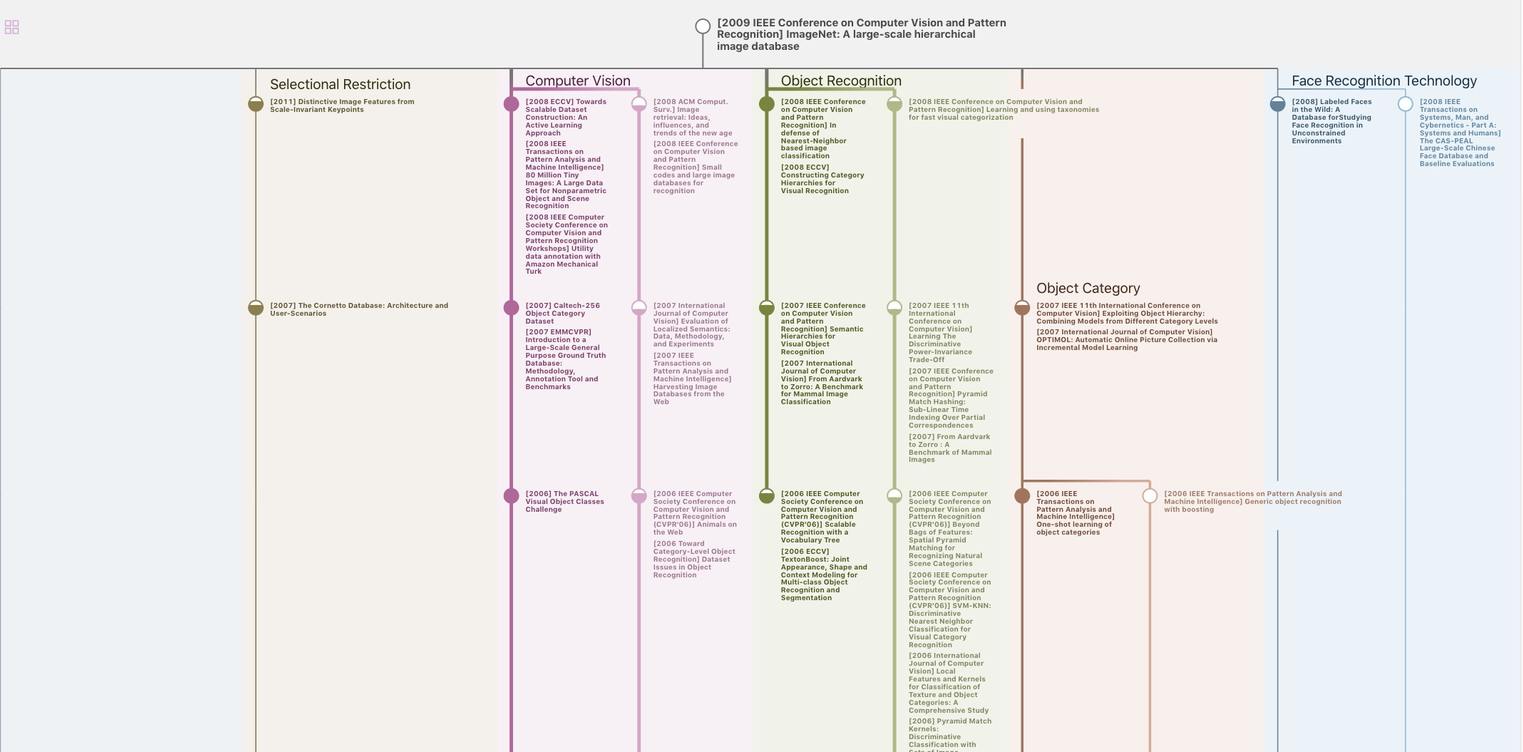
生成溯源树,研究论文发展脉络
Chat Paper
正在生成论文摘要