A lightweight early forest fire and smoke detection method
The Journal of Supercomputing(2023)
摘要
Forest fire is natural disasters that are sudden, destructive and difficult to handle and rescue, with millions of hectares of forests burned every year all over the world, causing serious ecological damage, loss of life and property. Therefore, timely detection and treatment of early fire is of positive and important significance for forest fire early control. The fire detection method based on image processing is one of the most important means of preventing the occurrence of large-scale forest fires at present by extracting the flame and smoke features in the image and quickly determining the location of the fire. The current deep learning-based forest fire early detection methods have problems such as high false alarm rate due to small detection targets and complex environmental background, and large number of detection model parameters. In view of this, this paper proposes a lightweight early forest fire and smoke detection method based on GS-YOLOv5. Firstly, this paper proposes a novel Super-SPPF structure to replace the SPPF structure in YOLOv5, by which the output of the feature extraction network is used as input, and the input is divided into two branches to retain more semantic information, and the GhostConv operation is performed separately to reduce the number of model parameters. The Super-SPPF structure performs the serial MaxPooling operation on one of the branches, which improves the computation speed by choosing a smaller pooling kernel, and then fuses the outputs of the two branches to reduce the false alarm rate of the detection model. Secondly, C3Ghost is utilized instead of the C3 module in YOLOv5 to further reduce the number of detection model parameters. Finally, the coordinate attention (CA) module is introduced in backbone of YOLOv5 to obtain the relationship between channels and space, which enables the network to obtain the location information of interest more accurately and further improves the detection accuracy of early fires. In this paper, a self-constructed DL-Fire dataset is used to verify the performance of the GS-YOLOv5 detection model by collecting environmental interference samples and combining them with the D-Fire dataset. The experimental results show that the detection accuracy of GS-YOLOv5 is 95.9
更多查看译文
关键词
Forest fire detection,YOLOv5,Super-SPPF,Ghost module
AI 理解论文
溯源树
样例
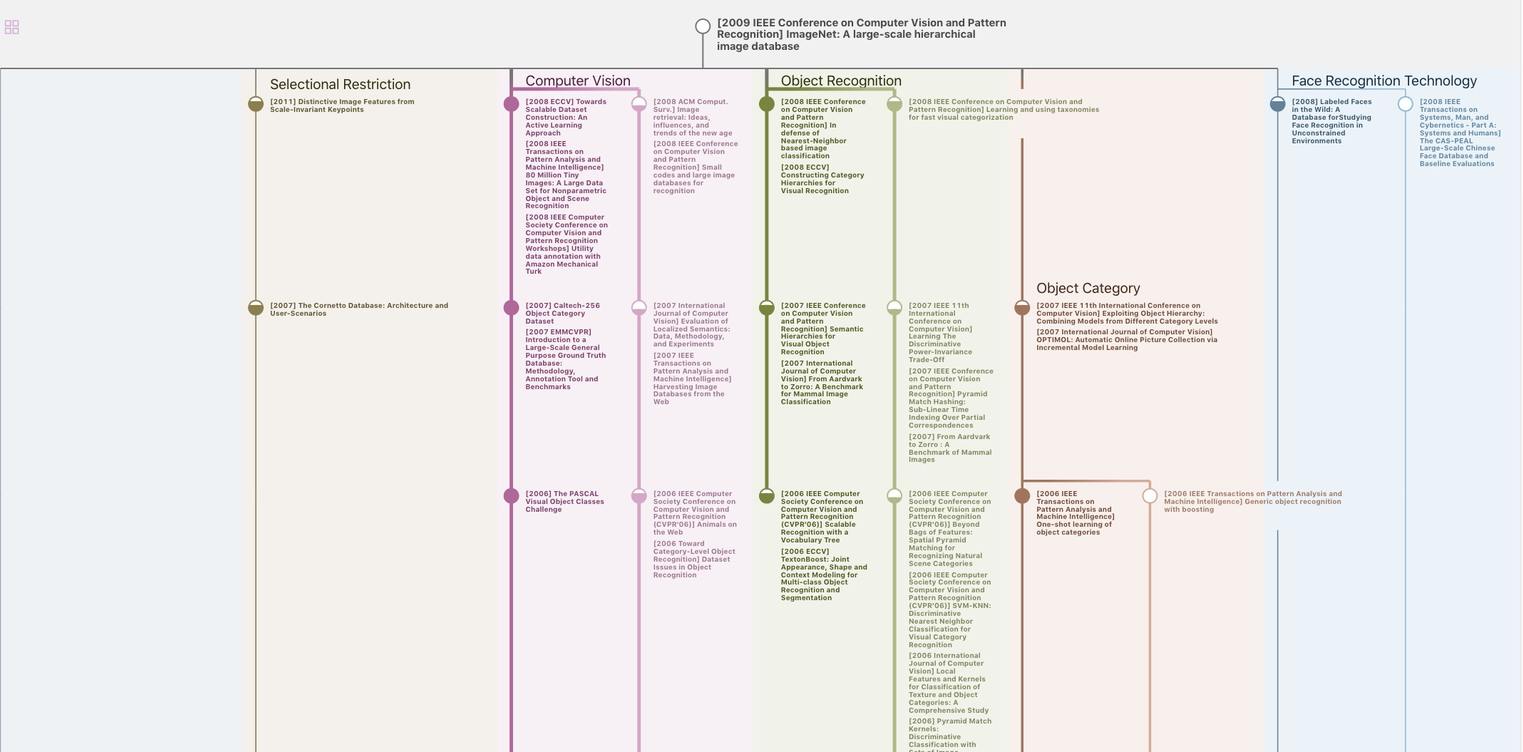
生成溯源树,研究论文发展脉络
Chat Paper
正在生成论文摘要