Active-set based block coordinate descent algorithm in group LASSO for self-exciting threshold autoregressive model
Statistical Papers(2023)
摘要
Group LASSO (gLASSO) estimator has been recently proposed to estimate thresholds for the self-exciting threshold autoregressive model, and a group least angle regression (gLAR) algorithm has been applied to obtain an approximate solution to the optimization problem. Although gLAR algorithm is computationally fast, it has been reported that the algorithm tends to estimate too many irrelevant thresholds along with the relevant ones. This paper develops an active-set based block coordinate descent (aBCD) algorithm as an exact optimization method for gLASSO to improve the performance of estimating relevant thresholds. Methods and strategy for choosing the appropriate values of shrinkage parameter for gLASSO are also discussed. To consistently estimate relevant thresholds from the threshold set obtained by the gLASSO, the backward elimination algorithm (BEA) is utilized. We evaluate numerical efficiency of the proposed algorithms, along with the Single-Line-Search (SLS) and the gLAR algorithms through simulated data and real data sets. Simulation studies show that the SLS and aBCD algorithms have similar performance in estimating thresholds although the latter method is much faster. In addition, the aBCD-BEA can sometimes outperform gLAR-BEA in terms of estimating the correct number of thresholds under certain conditions. The results from case studies have also shown that aBCD-BEA performs better in identifying important thresholds.
更多查看译文
关键词
Karush–Kuhn–Tucker,Group LASSO,SETAR,aBCD algorithm,BEA,Sparsity conditions
AI 理解论文
溯源树
样例
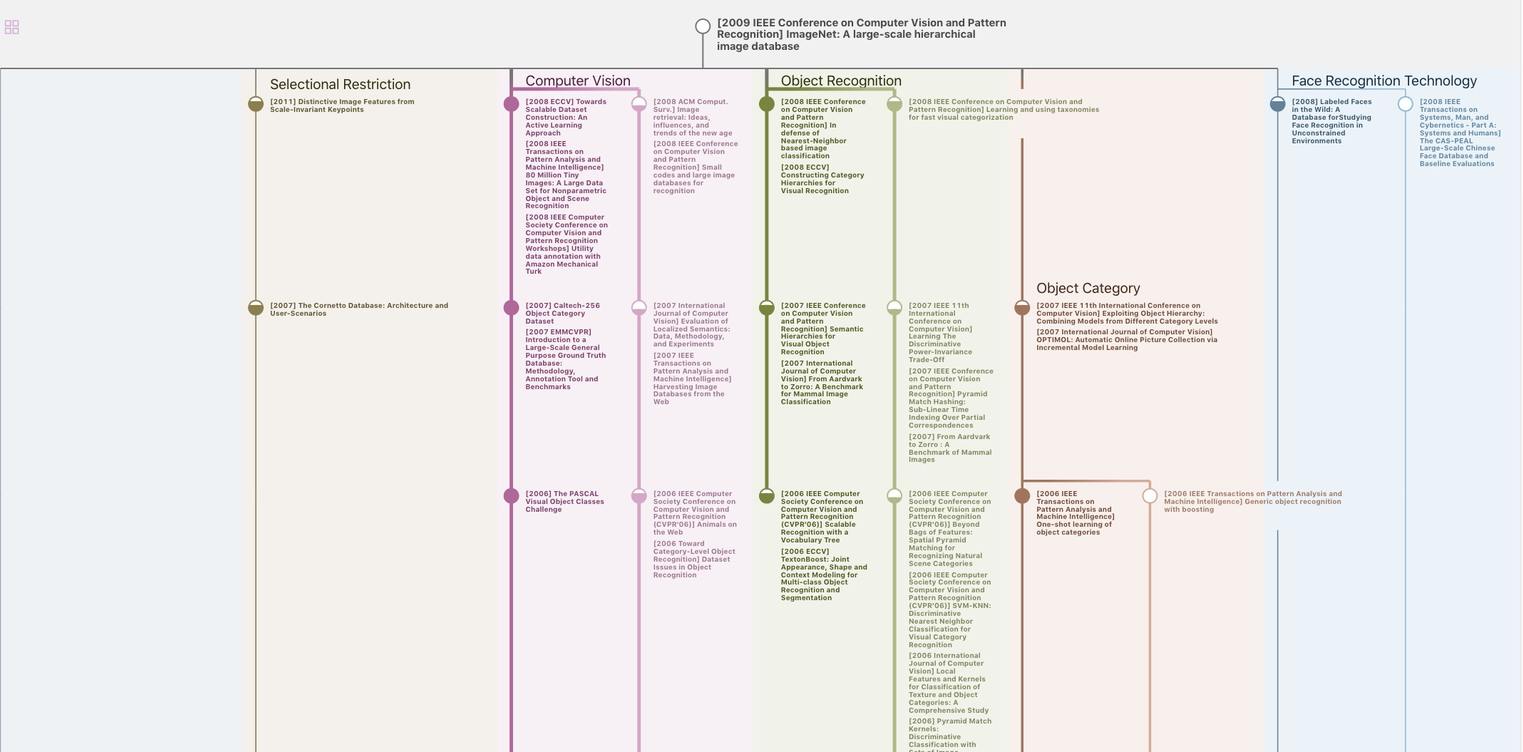
生成溯源树,研究论文发展脉络
Chat Paper
正在生成论文摘要