Development of robust fault diagnosis model for variable situations in robotic spot-welding (RSW) process based on transfer learning
Journal of Mechanical Science and Technology(2023)
摘要
A key component of vehicle production automation is an industrial robot, and the robotic spot-welding (RSW) process is crucial due to more than 5000 spot-welds per vehicle. Due to the large number of welds, the quality of each weld must be adequately monitored and controlled; therefore, an automatic diagnostic system is required. In this paper, a robust fault diagnostic system is developed and evaluated for variable RSW process situations. Among the numerous RSW defects, electrode tip wear has been selected as a significant abnormal state that can lead to undersized welds. In addition, the thickness of the welding specimen is selected as a variable situation, and three thickness values — 0.6, 0.8, and 1.0 mm — are considered as the variable situations. On the basis of 1D convolutional neural networks (1D-CNN) and transfer learning (TL) methods, a robust diagnostic model is established. The diagnostic accuracy is then used to compare and evaluate the performance of the TL-based models.
更多查看译文
关键词
Vehicle production system,Welding quality control,Data-driven approach,Artificial intelligence,Prognostics and health management
AI 理解论文
溯源树
样例
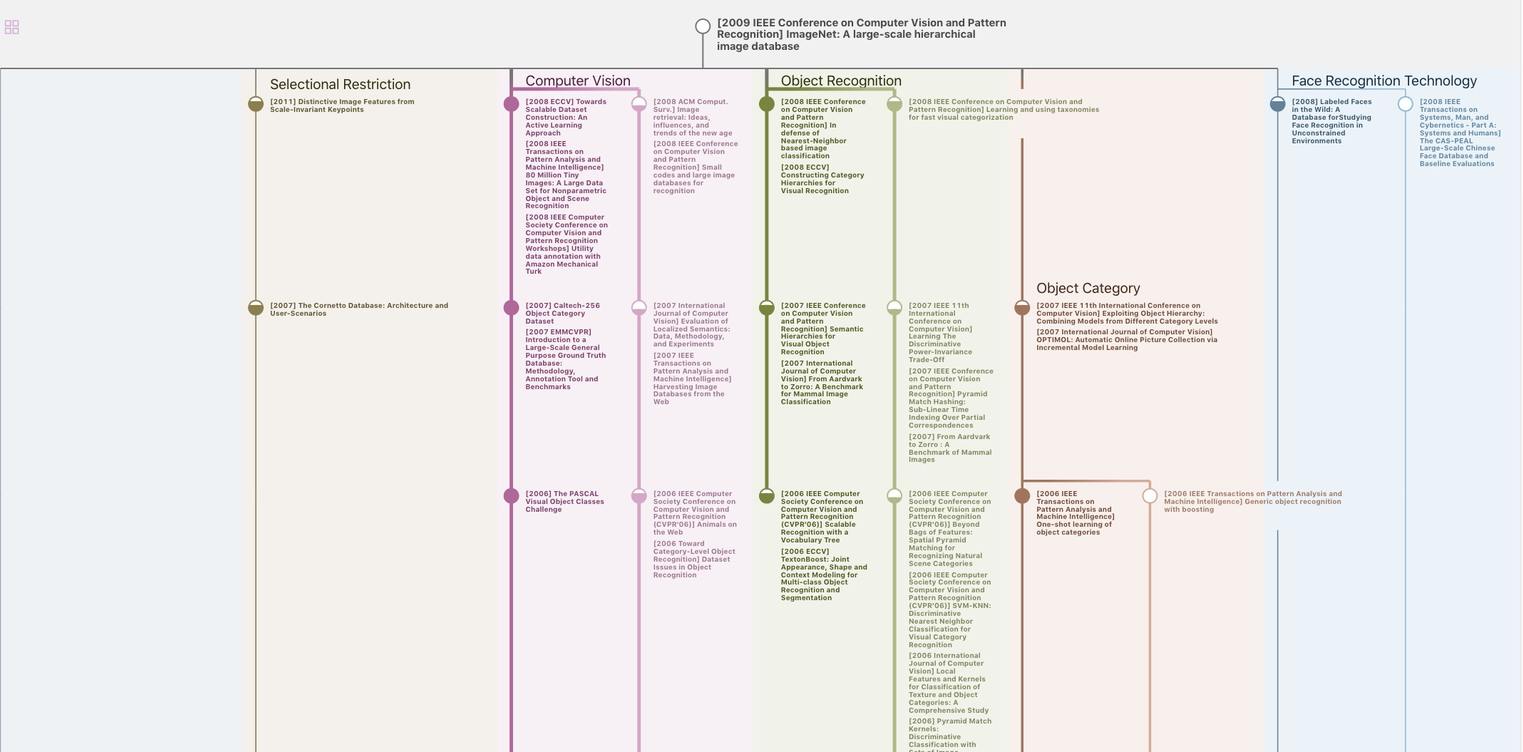
生成溯源树,研究论文发展脉络
Chat Paper
正在生成论文摘要