Industrial IoT–enabled real-time prediction of strip cross-section shape for hot-rolling steel
The International Journal of Advanced Manufacturing Technology(2023)
摘要
Because strip cross sections cannot be obtained during hot rolling in advance, traditional automatic shape control systems can only rely on the measured shape at the exit of the final mill for feedback control, which causes a significant lag and poor adjustment effect. To accurately predict the cross-sectional shape, an industrial Internet of things platform for steel plants is developed to collect real-time production data. A novel real-time prediction model that can determine the cross sections of strips is proposed to address the drawbacks of traditional data-driven methods that perform offline predictions. This model is established by adopting a dynamic mode decomposition algorithm (DMD) to optimize the sparse identification of nonlinear dynamics (SINDy). A practical dataset of 81 variables from a 2250-mm hot-rolling production line is utilized to validate the proposed method, and this method is compared with SINDy, EMD-optimized SINDy, and VMD-optimized SINDy. The experimental results show that the proposed method can achieve higher prediction accuracy and more minor errors.
更多查看译文
关键词
Cross-section shape,Hot rolling,Industrial Internet of things platform,Dynamic mode decomposition algorithm,Sparse identification of nonlinear dynamics
AI 理解论文
溯源树
样例
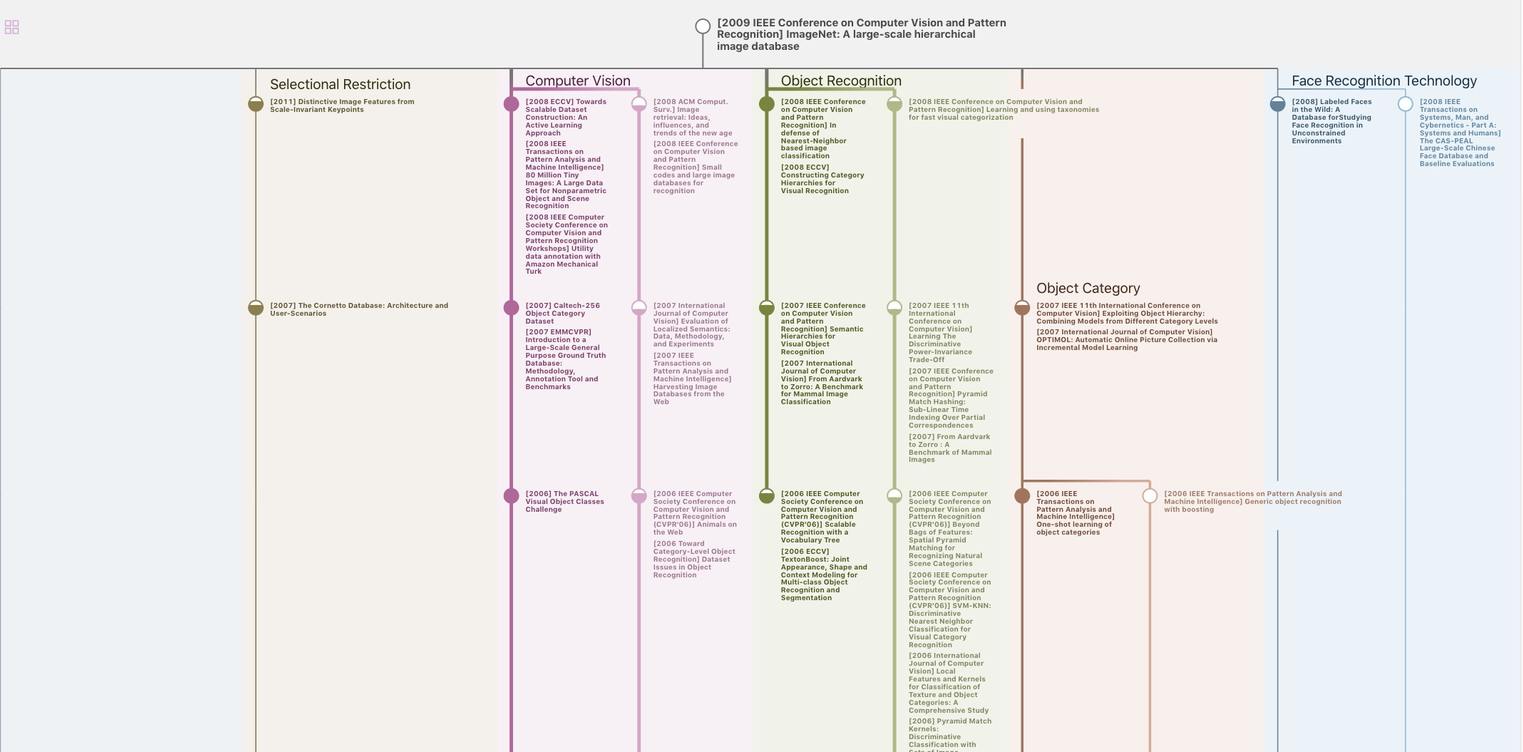
生成溯源树,研究论文发展脉络
Chat Paper
正在生成论文摘要