Single image super-resolution via global aware external attention and multi-scale residual channel attention network
International Journal of Machine Learning and Cybernetics(2023)
摘要
Recently, deep convolutional neural networks (CNNs) have shown significant advantages in improving the performance of single image super-resolution (SISR). To build an efficient network, multi-scale convolution is commonly incorporated into CNN-based SISR methods via scale features with different perceptive fields. However, the feature correlations of the same sample are not fully utilized by the existing multi-scale SISR approaches, impeding the further improvement of reconstruction performance. In addition, the correlations between different samples are still left unexplored. To address these problems, this paper proposes a deep-connected multi-scale residual attention network (DMRAN) by virtue of the feature correlations of the same sample and the correlations between different samples. Specifically, we propose a deep-connected multi-scale residual attention block (DMRAB) to take fully advantage of the multi-scale and hierarchical features, which can effectively learn the local interdependencies between channels by adjusting the channel features adaptively. Meanwhile, a global aware external attention (GAEA) is introduced to boost the performance of SISR by learning the correlations between all the samples. Furthermore, we develop a deep feature extraction structure (DFES), which seamlessly combines the stacked deep-connected multi-scale residual attention groups (DMRAG) with GAEA to learn deep feature representations incrementally. Extensive experimental results on the public benchmark datasets show the superiority of our DMRAN to the state-of-the-art SISR methods.
更多查看译文
关键词
Single image super-resolution,Deep feature extraction structure,Deep-connected multi-scale residual attention block,Local aware channel attention,Global aware external attention
AI 理解论文
溯源树
样例
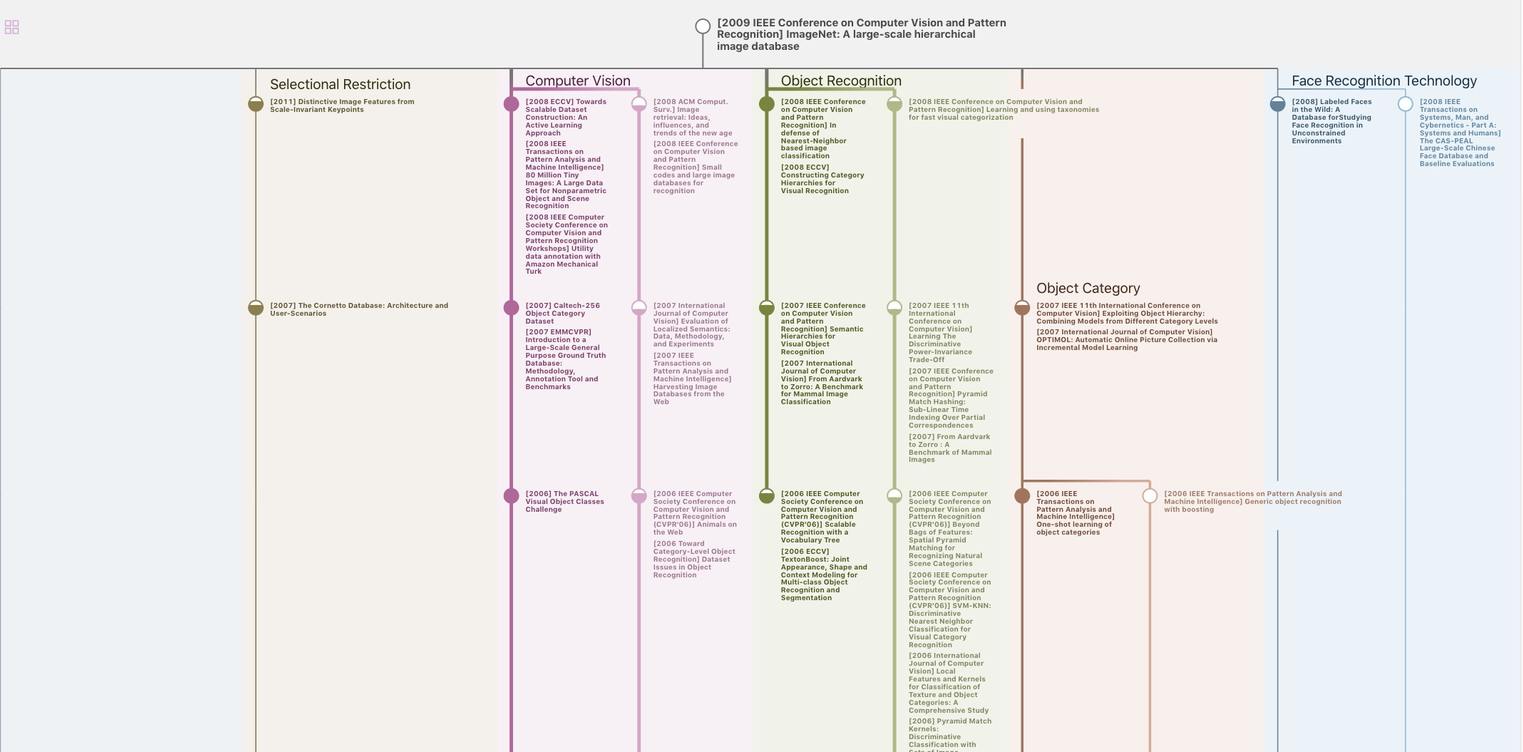
生成溯源树,研究论文发展脉络
Chat Paper
正在生成论文摘要