N-IoU: better IoU-based bounding box regression loss for object detection
Neural Computing and Applications(2023)
摘要
Object detection is one of the core tasks of computer vision, and bounding box (bbox) regression is one of the basic tasks of object detection. In recent years of related research, bbox regression is often used in the Intersection over Union (IoU) loss and its improved version. In this paper, for the first time, we introduce the Dice coefficient into the regression loss calculation and propose a new measure which is superior to and can replace the IoU. We define three properties of the new measure and prove the theory by mathematical reasoning and analysis of the existing work. This paper also proposes the N-IoU regression loss family. And the superiority of the N-IoU regression loss family is proved by designing simulation experiments and comparative experiments. The main results of this paper are: (1) The proposed new measure is better than IoU which can be used to evaluate bounding box regression, and the three properties of the new measure can be used as a broad criterion for the design of regression loss functions; and (2) we propose N-IoU loss. The parameter n of N-IOU can be debugged, which can be widely adapted to different application scenarios with higher flexibility, and the regression performance is better.
更多查看译文
关键词
Bounding box regression,IoU,N-IoU,Regression loss,Object detection,Computer vision
AI 理解论文
溯源树
样例
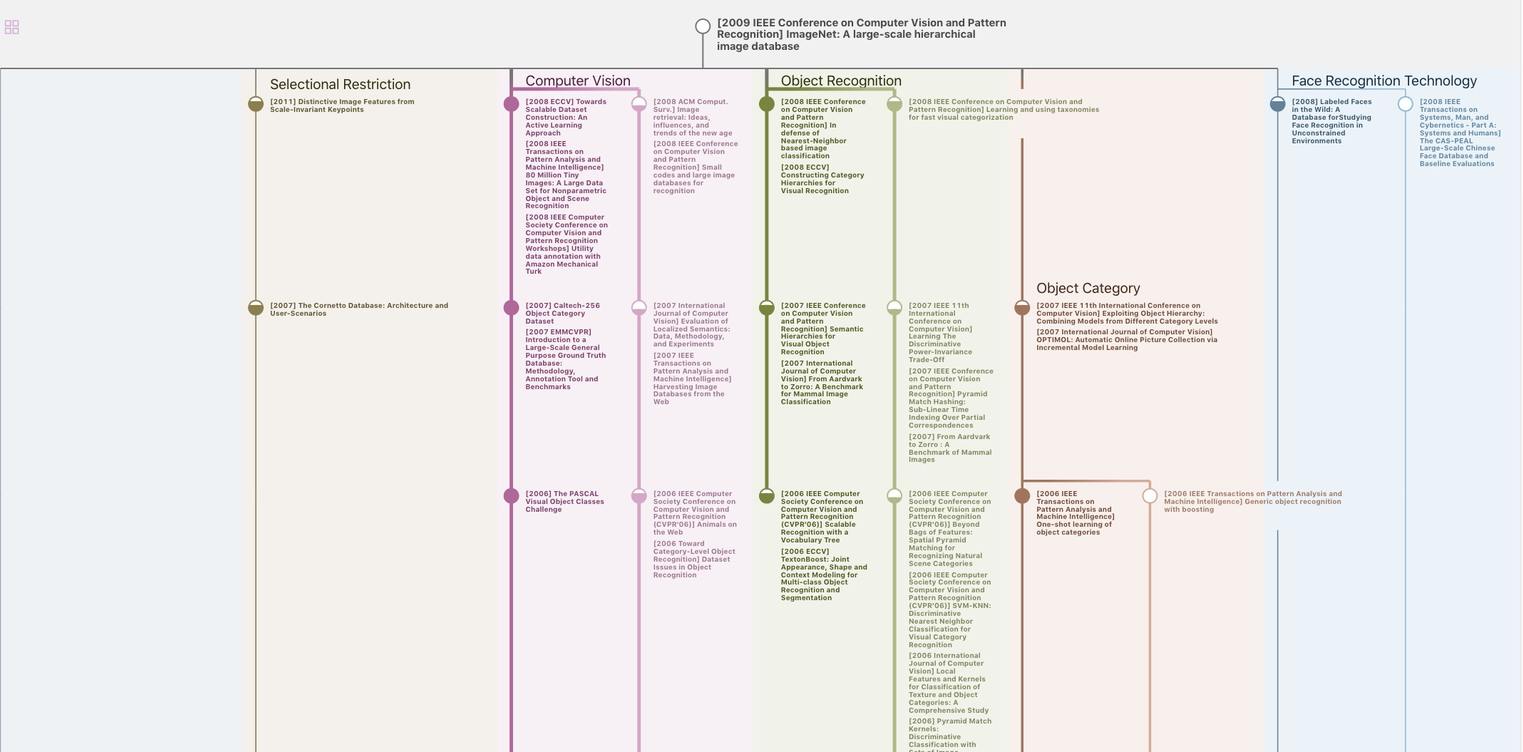
生成溯源树,研究论文发展脉络
Chat Paper
正在生成论文摘要