Beyond Weisfeiler-Lehman with Local Ego-Network Encodings
MACHINE LEARNING AND KNOWLEDGE EXTRACTION(2023)
摘要
Identifying similar network structures is key to capturing graph isomorphisms and learning representations that exploit structural information encoded in graph data. This work shows that ego networks can produce a structural encoding scheme for arbitrary graphs with greater expressivity than the Weisfeiler-Lehman (1-WL) test. We introduce IGEL, a preprocessing step to produce features that augment node representations by encoding ego networks into sparse vectors that enrich message passing (MP) graph neural networks (GNNs) beyond 1-WL expressivity. We formally describe the relation between IGEL and 1-WL, and characterize its expressive power and limitations. Experiments show that IGEL matches the empirical expressivity of state-of-the-art methods on isomorphism detection while improving performance on nine GNN architectures and six graph machine learning tasks.
更多查看译文
关键词
graph neural networks,graph representation learning,Weisfeiler-Lehman,graph isomorphism,GNN expressivity,ego networks
AI 理解论文
溯源树
样例
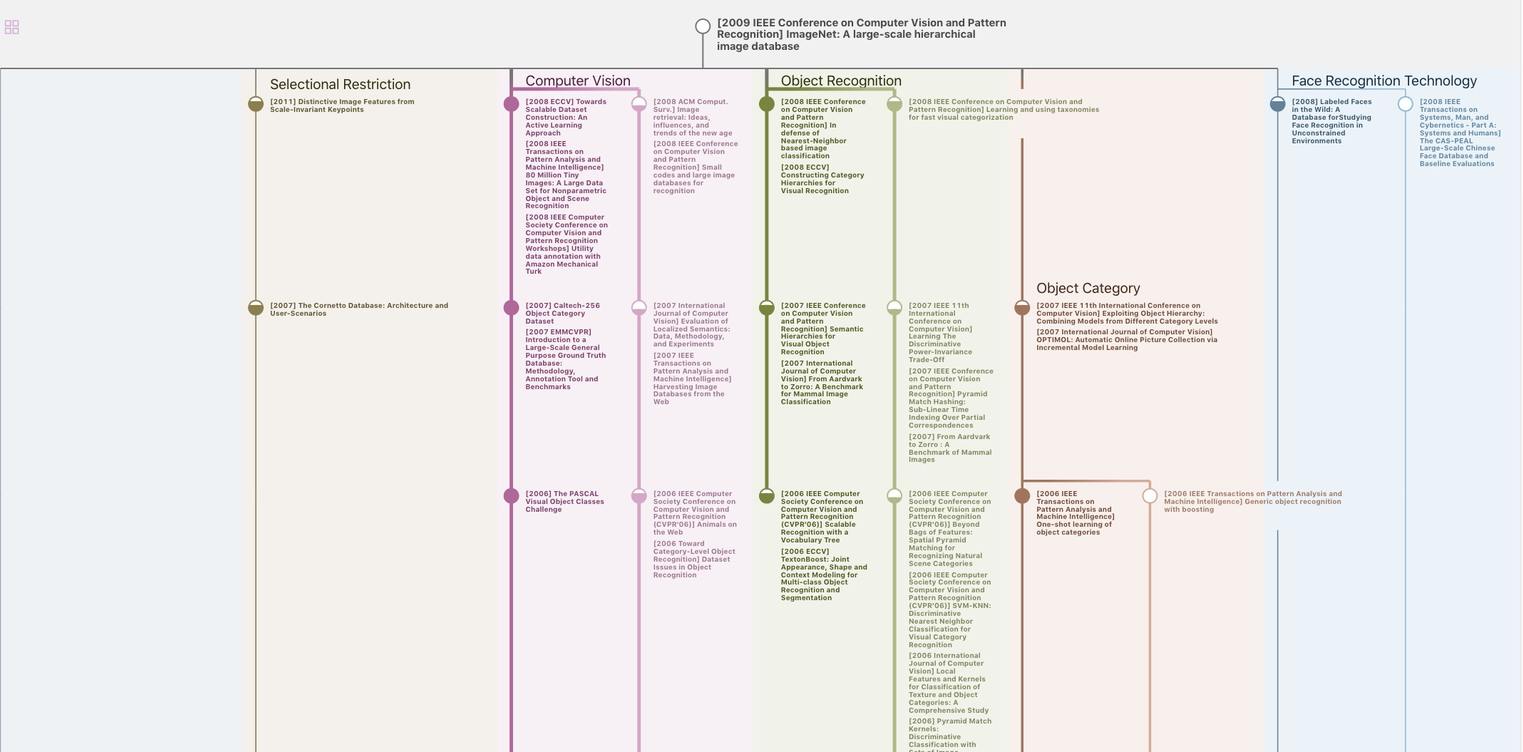
生成溯源树,研究论文发展脉络
Chat Paper
正在生成论文摘要