EndoNet: A Model for the Automatic Calculation of H-Score on Histological Slides
INFORMATICS-BASEL(2023)
摘要
H-score is a semi-quantitative method used to assess the presence and distribution of proteins in tissue samples by combining the intensity of staining and the percentage of stained nuclei. It is widely used but time-consuming and can be limited in terms of accuracy and precision. Computer-aided methods may help overcome these limitations and improve the efficiency of pathologists' workflows. In this work, we developed a model EndoNet for automatic H-score calculation on histological slides. Our proposed method uses neural networks and consists of two main parts. The first is a detection model which predicts the keypoints of centers of nuclei. The second is an H-score module that calculates the value of the H-score using mean pixel values of predicted keypoints. Our model was trained and validated on 1780 annotated tiles with a shape of 100 x 100 mu m and we achieved 0.77 mAP on a test dataset. We obtained our best results in H-score calculation; these results proved superior to QuPath predictions. Moreover, the model can be adjusted to a specific specialist or whole laboratory to reproduce the manner of calculating the H-score. Thus, EndoNet is effective and robust in the analysis of histology slides, which can improve and significantly accelerate the work of pathologists.
更多查看译文
关键词
object detection,digital pathology,deep learning,prediction model,neural network
AI 理解论文
溯源树
样例
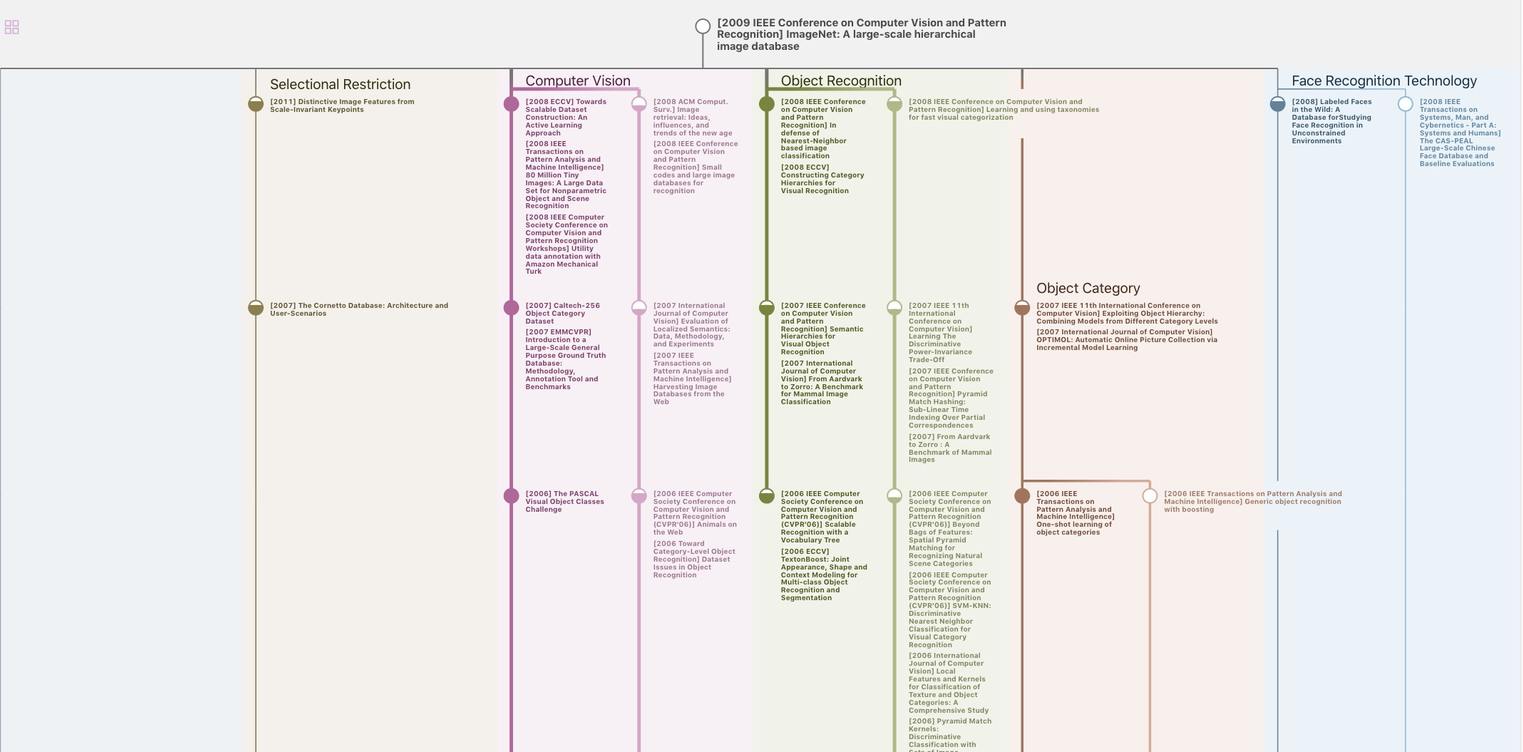
生成溯源树,研究论文发展脉络
Chat Paper
正在生成论文摘要