Estimating Fine Fuel Load Using Sentinel-2A Imagery and Machine Learning: A Case Study in the Mountainous Forests of Changsha, China
REMOTE SENSING(2023)
摘要
Fine fuel load (FFL) is a crucial variable influencing the occurrence of wildfire. Accurate knowledge of the distribution of FFL in mountainous forests is essential for ongoing wildfire risk management and the stability of mountain ecosystems. Traditional methods of estimating forest fuel load typically involve ground surveys combined with remote sensing, which can be costly and inefficient. Therefore, low-cost, large-scale FFL estimation remains challenging. In this study, Sentinel-2A satellite imagery from the Changsha forest region was used as the data source. Firstly, different feature variables were constructed based on false-color (B843), true-color (B432), four-band (B8432) combinations, and the Normalized Difference Water Index (NDWI). Subsequently, a machine learning approach based on random convolution was employed to estimate FFL. This study also included accuracy assessments of the estimation results and the creation of FFL maps for the study area. The results showed that the FFL estimation based on the B8432 band combination achieved the highest accuracy, with RMSE and R2 values of 5.847 t center dot hm-2 and 0.656, respectively. FFL estimation results based on false-color imagery followed, with true-color imagery and NDWI index-based estimation results exhibiting lower accuracy. This study offers critical FFL insights using random convolution techniques applied to Sentinel-2A imagery, enhancing the ability to monitor and manage forest fuel conditions effectively, thereby facilitating more informed regional wildfire risk management strategies.
更多查看译文
关键词
fine fuel load,wildfire,mountain ecosystem,Sentinel-2A,random convolution,machine learning
AI 理解论文
溯源树
样例
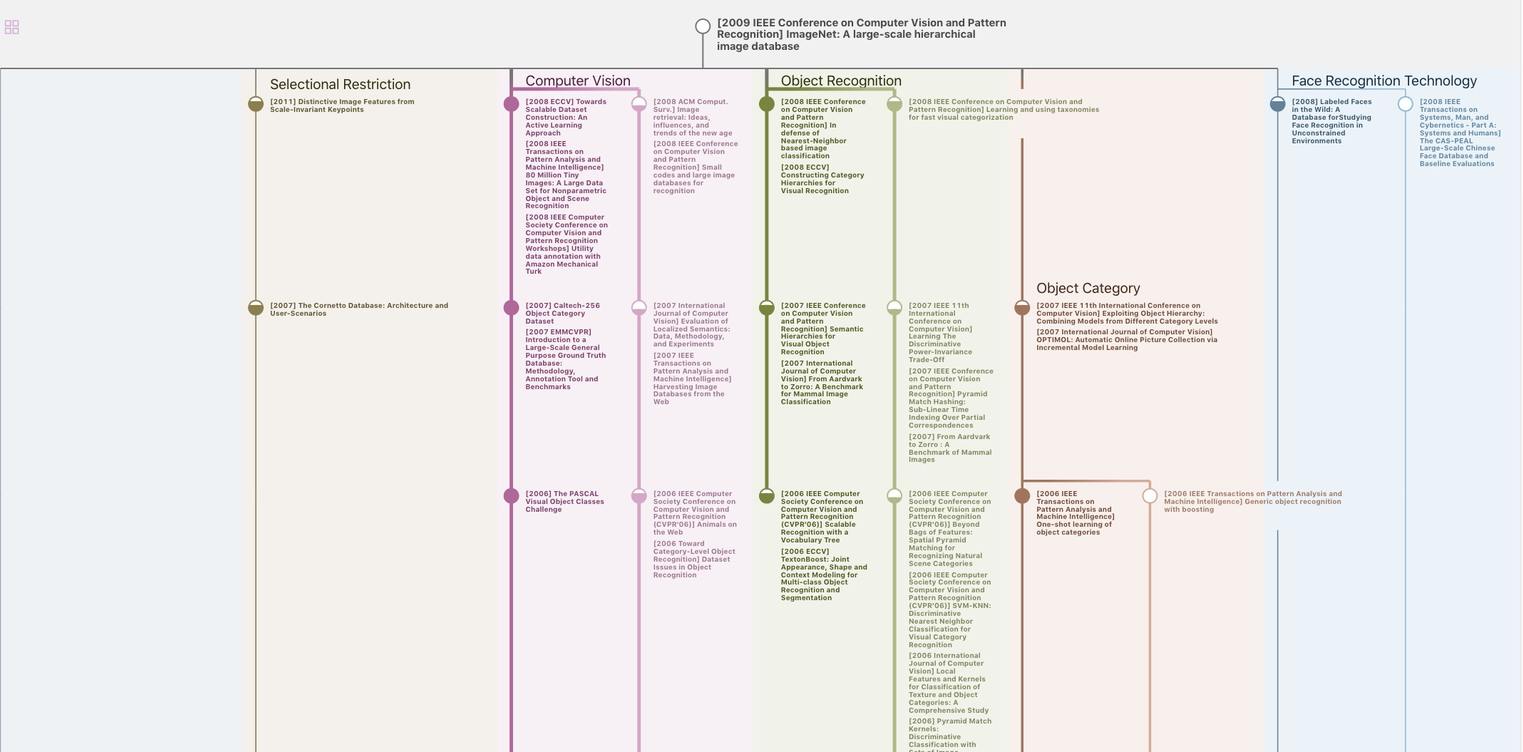
生成溯源树,研究论文发展脉络
Chat Paper
正在生成论文摘要