Fedisp: an incremental subgradient-proximal-based ring-type architecture for decentralized federated learning
Complex & Intelligent Systems(2023)
摘要
Federated learning (FL) represents a promising distributed machine learning paradigm for resolving data isolation due to data privacy concerns. Nevertheless, most vanilla FL algorithms, which depend on a server, encounter the problem of reliability and a high communication burden in real cases. Decentralized federated learning (DFL) that does not follow the star topology faces the challenges of weight divergence and inferior communication efficiency. In this paper, a novel DFL framework called federated incremental subgradient-proximal (FedISP) is proposed that utilizes the incremental method to perform model updates to alleviate weight divergence. In our setup, multiple clients are distributed in a ring topology and communicate in a cyclic manner, which significantly mitigates the communication load. A convergence guarantee is given under the convex condition to demonstrate the impact of the learning rate on our algorithms, which further improves the performance of FedISP. Extensive experiments on benchmark datasets validate the effectiveness of the proposed approach in both independent and identically distributed (IID) and non-IID settings while illustrating the advantages of the FedISP algorithm in achieving model consensus and saving communication costs.
更多查看译文
关键词
Decentralized machine learning,Federated learning (FL),Incremental methods,Non-IID data
AI 理解论文
溯源树
样例
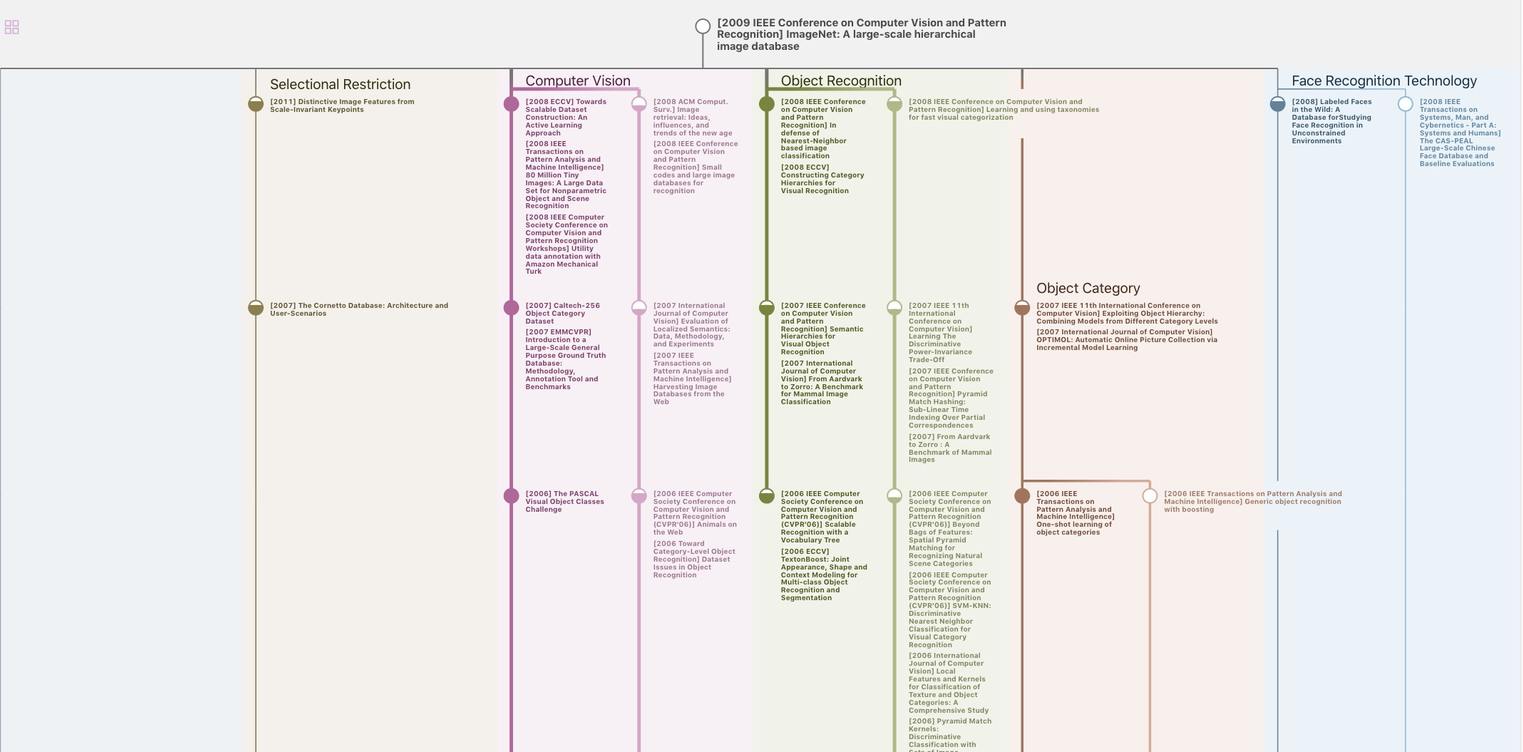
生成溯源树,研究论文发展脉络
Chat Paper
正在生成论文摘要