Fast prediction and control of air core in hydrocyclone by machine learning to stabilize operations
JOURNAL OF ENVIRONMENTAL CHEMICAL ENGINEERING(2024)
摘要
Operation stability significantly impacts hydrocyclone separation performance during wastewater treatment, sludge processing, and microplastic removal from water. The air core inside a hydrocyclone is an important indicator of operation stability. This paper presents a machine learning model designed for fast prediction and control of air core profiles. The model is built upon a modified graph neural network (GNN). It is trained by the data generated from a well-established and validated computational fluid dynamics (CFD) model. This GNNbased surrogate model has undergone two modifications to enhance its prediction accuracy. One is data smoothing, to mitigate the adverse effects of the drastic data change in spatial distributions. The other is the loss function modification to incorporate the air core information acquired by the CFD model. The predicted air cores are compared with the original GNN and random forest (RF) against the CFD results. It shows that the new surrogate model can reproduce air profiles and have higher accuracy than other models in predicting spatial distribution results among different error metrics. Furthermore, this surrogate model is combined with the genetic algorithm to optimize the air core. The proposed machine learning model framework offers a promising avenue for the prediction and control of hydrocyclones.
更多查看译文
关键词
Hydrocyclone,Air core,Graph neural network,Loss function modification,Data smoothing
AI 理解论文
溯源树
样例
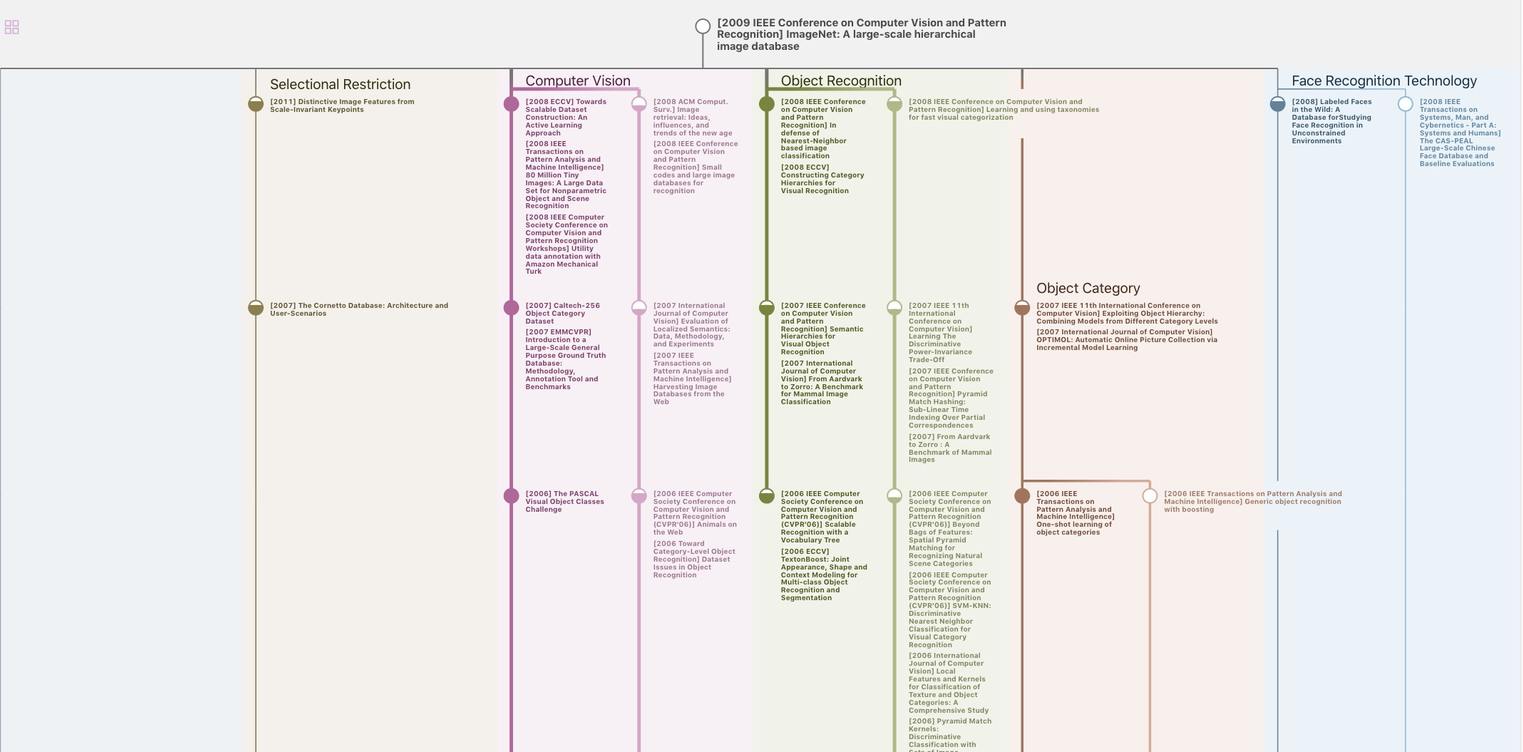
生成溯源树,研究论文发展脉络
Chat Paper
正在生成论文摘要