Reliability and Performance Evaluation of Two-input Machine Learning Systems.
2023 IEEE 28th Pacific Rim International Symposium on Dependable Computing (PRDC)(2023)
摘要
The multiple-input machine learning system (MLS) is a system architecture exploiting data diversity to improve the output reliability of the system by comparing prediction results on multiple input data. While the output reliability is enhanced by redundancy, the architecture imposes additional costs and non-negligible processing overheads. The performance of multiple-input MLSs has been theoretically investigated in the previous study using queueing analysis. However, it is little known how real MLSs are impacted by the multiple predictions and comparison processes needed in the architecture. In this paper, we implement two-input MLSs in two different configurations, a parallel type architecture and a shared type architecture, and evaluate the reliability, performance, and energy consumption of the system by experiments. Our empirical results unveil several advantages of the shared type architecture that can suppress the increases in response time and energy consumption by using a shared machine learning module for predictions of two inputs. We also compare the results of the performance simulation of two-input MLS with the empirical results. While we confirm the effectiveness of the simulation, we also find some gaps in the real observations. For example, we observe that the inference time distribution fits well in the log-normal distribution rather than the exponential distribution assumed in the simulation. Our findings could be useful for developing performance models for multiple-input MLSs.
更多查看译文
关键词
Energy consumption,Machine learning system,Performance,Reliability,Simulation
AI 理解论文
溯源树
样例
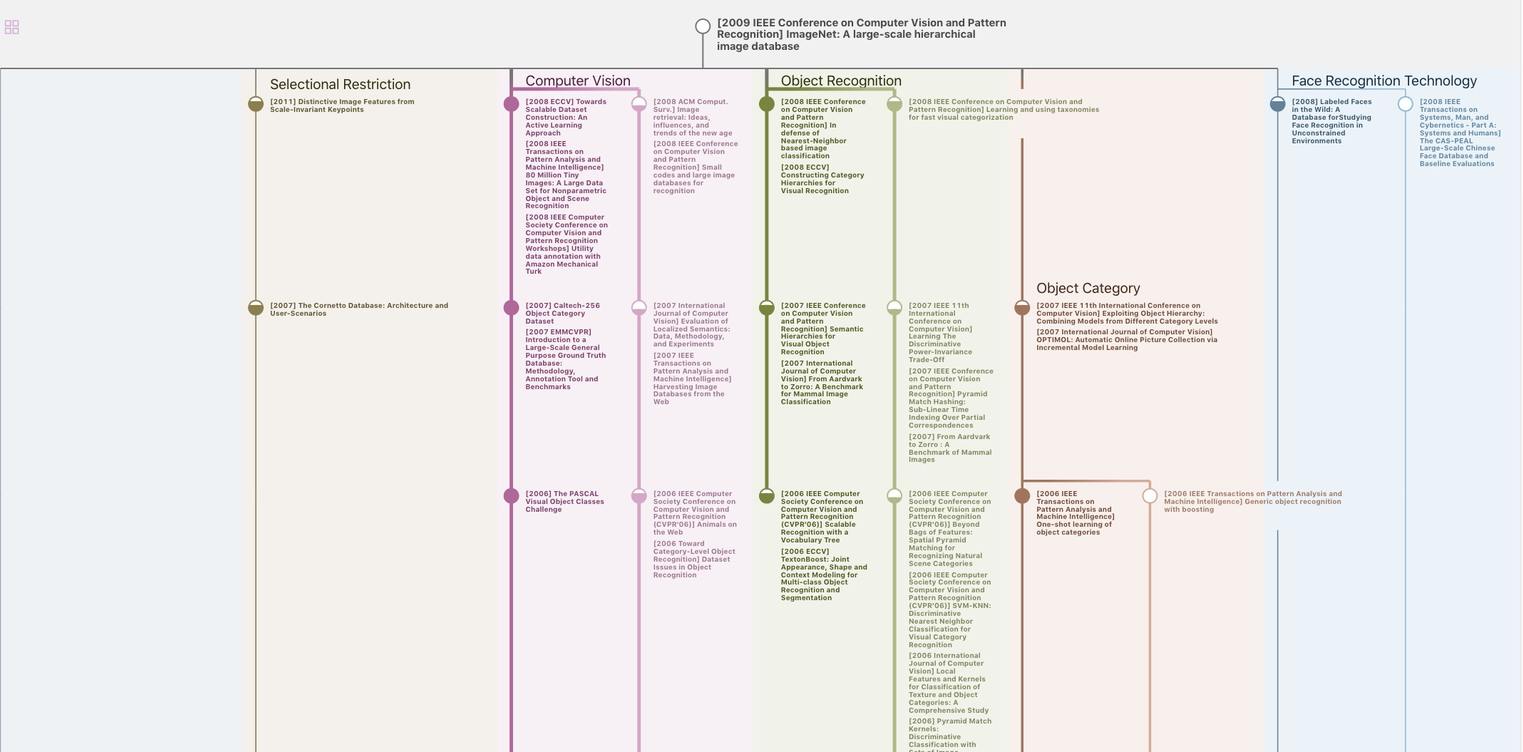
生成溯源树,研究论文发展脉络
Chat Paper
正在生成论文摘要