Performance and Domain Adaptability of Object Detection Algorithms in Processed Colonoscopy Videos.
2023 12th International Conference on Awareness Science and Technology (iCAST)(2023)
摘要
Commercial computer-aided diagnosis (CAD) systems have been approved for clinical colonoscopy. However, the detected results of these systems can only be observed visually. In this paper, we compared different object detection models to detect the indication boxes generated by CAD systems during colonoscopy. Additionally, we also investigated the domain adaptability of these models for the detection in different sources. All the models were trained by 1,362 images recorded and analyzed by CAD EYE system. In a test set A composed of 401 images from the same system, Dynamic R-CNN achieved a precision 100%, sensitivity 99.6%, F1 score 99.8% and mAP(50-95) 87.2% for detection indication boxes. In a test set B composed of 1,474 images from EndoBRAIN-EYE system, Dynamic R-CNN achieved a precision 96%, sensitivity 99.9%, F1 score 97.9% and mAP(50-95) 78.7% for detection indication boxes. These results demonstrate that Dynamic R-CNN possesses a high detection rate for detecting the indication boxes generated by the CAD system in colonoscopy, also have exceptional domain adaptability.
更多查看译文
关键词
Colonoscopy,Object Detection,Computer Aided Diagnosis System
AI 理解论文
溯源树
样例
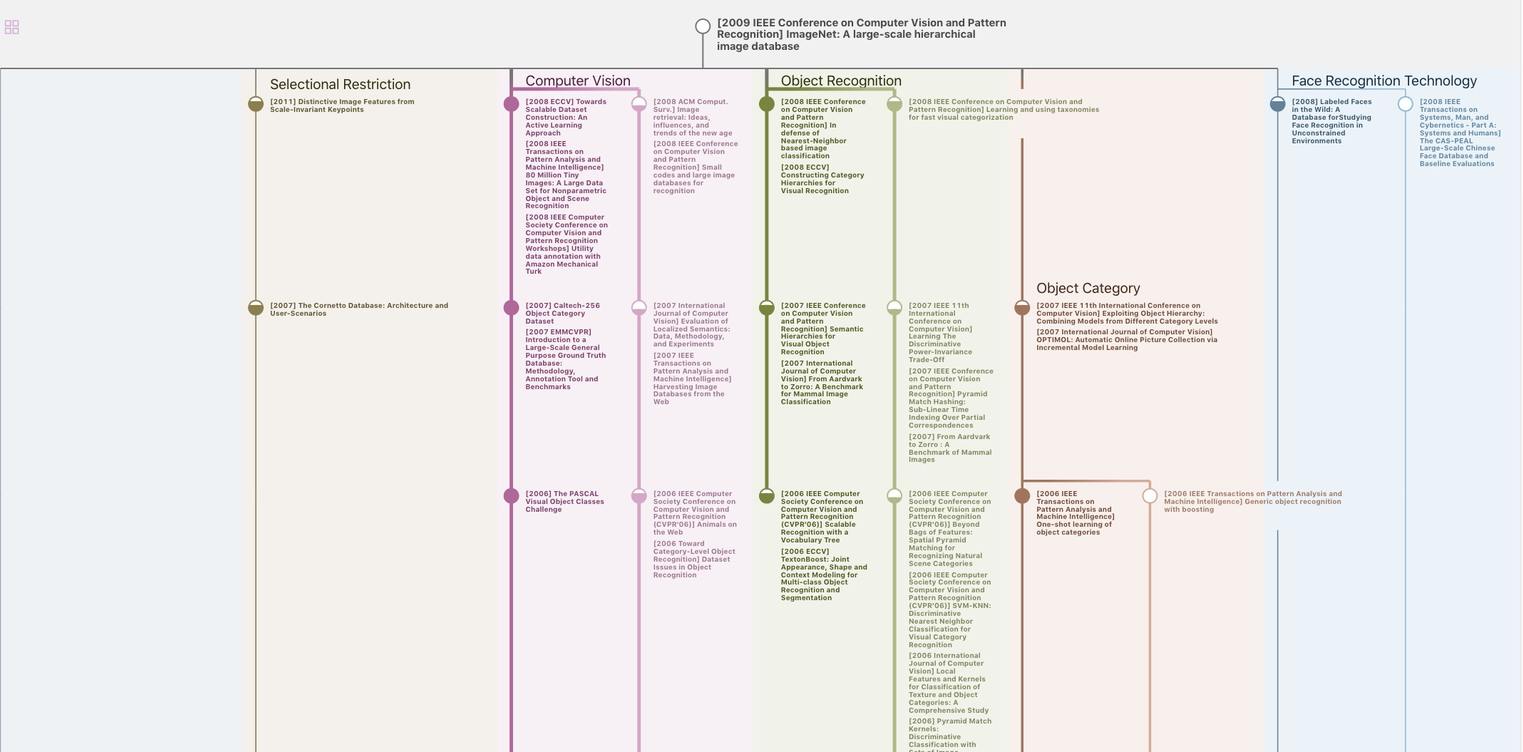
生成溯源树,研究论文发展脉络
Chat Paper
正在生成论文摘要