Deforming Garment Classification With Shallow Temporal Extraction and Tree-Based Fusion
IEEE ROBOTICS AND AUTOMATION LETTERS(2024)
摘要
A novel RGB-based continuous perception garment classification approach is proposed in this letter, with the aim of identifying the correct category of the garment from a set of categories. It has been observed that treating a video of the continuous deformation of cloth as a set of disordered static figures leads to low classification precision. On the contrary, investigating the temporal information between frames can significantly improve the quality of extracted features and increase classification performance. In this regard, we propose a hybrid temporal fusion RGB-based algorithm, including an improved image-level shallow temporal feature extraction module (STEM) and a binary-tree fusion module (BiTF) for adaptive feature fusion. STEM incorporates multi-scale optical flow and long-short-term memorised information to capture both static features in every single image and dynamic features in consecutive images. BiTF constructs a tree-shaped structure to fuse an arbitrary number of extracted features in a video. The proposed algorithm achieves 97.5% accuracy on the public dataset, surpassing the state-of-the-art RGB-based classification algorithm by 44.0% and achieving comparable performance with RGBD-based algorithms.
更多查看译文
关键词
Object detection,segmentation and categorization,deep learning for visual perception,shallow temporal feature extraction,binary-tree fusion
AI 理解论文
溯源树
样例
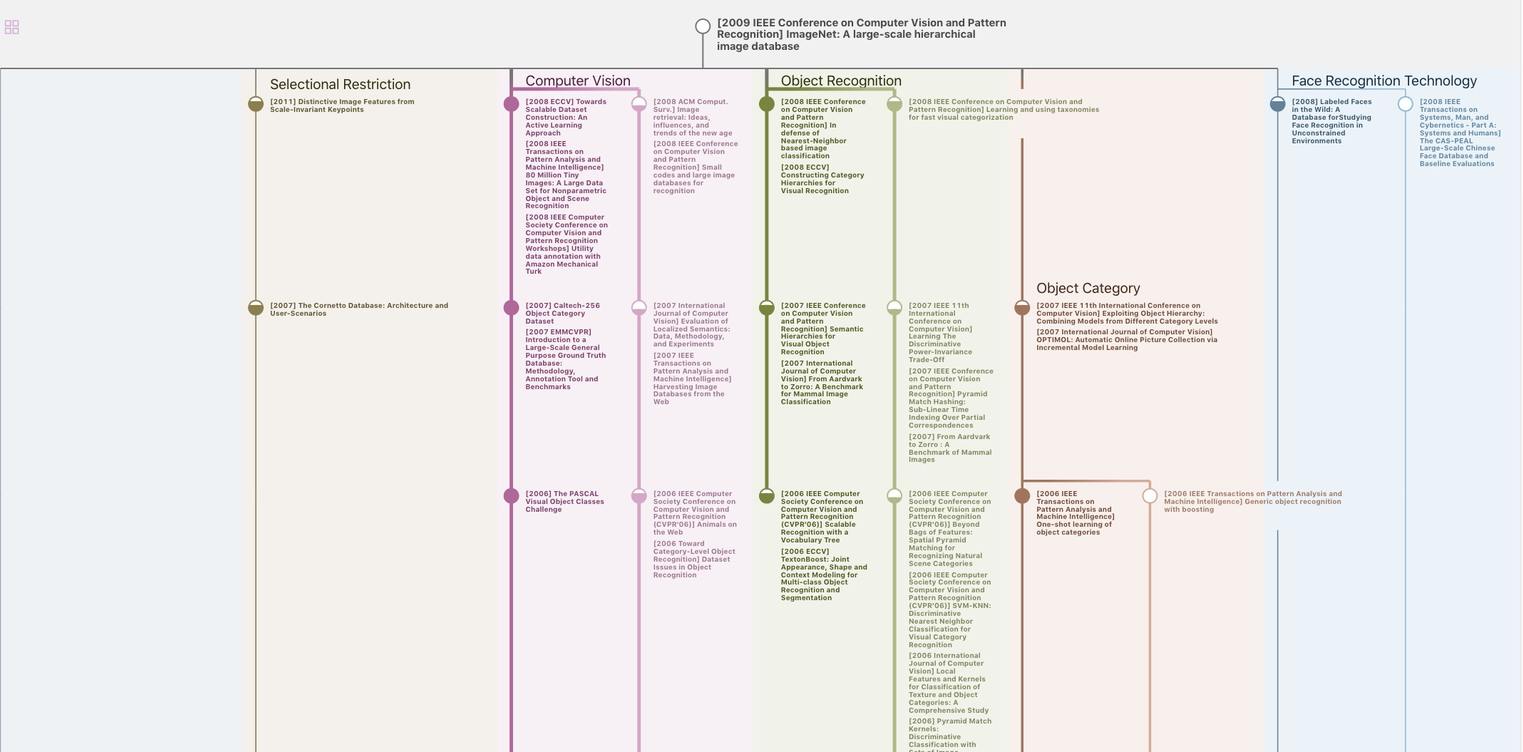
生成溯源树,研究论文发展脉络
Chat Paper
正在生成论文摘要