Elastic subspace diagnosis via graph-balanced discriminant projection
MEASUREMENT(2024)
摘要
Subspace learning of high-dimensional vibration signals is crucial for mechanical fault diagnosis. However, traditional subspace learning methods are difficult to acquire and balance the adaptive global and local intrinsic graph structures of fault data. To address this problem, we propose a novel elastic subspace diagnosis method based on graph balanced discriminant projection (GbDP). By inter-sample collaborative representation and supervision information, the method constructs adaptive global and local geometric graphs and further gives a graph balancing strategy for the optimization model of elastic subspace learning. On the basis of capturing the inherent discriminative structure of fault data, GbDP improves the intra-class aggregation and inter-class separability of the elastic subspace and achieves a relative balance of global and local structures. The results of the experimental cases on bearing and gear faults show that GbDP can extract more discriminative low-dimensional sensitive features and can effectively improve fault classification accuracy.
更多查看译文
关键词
Fault diagnosis,Subspace learning,Collaborative representation,Graph balanced
AI 理解论文
溯源树
样例
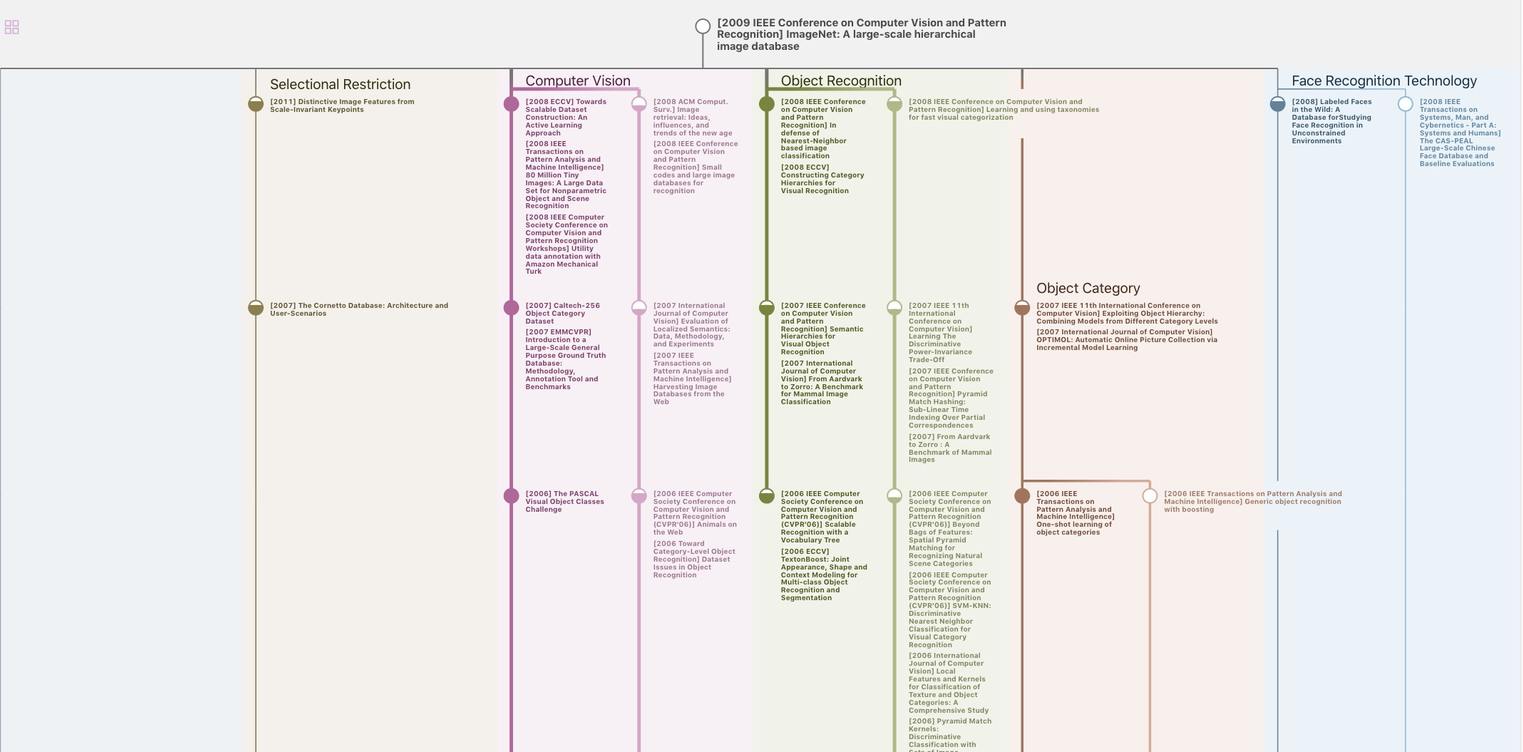
生成溯源树,研究论文发展脉络
Chat Paper
正在生成论文摘要