A Multi-scale feature modulation network for efficient underwater image enhancement
JOURNAL OF KING SAUD UNIVERSITY-COMPUTER AND INFORMATION SCIENCES(2024)
摘要
Many strategies have been proposed for underwater image enhancement. However, they are often incompatible with underwater remotely operated vehicle platforms with low memory and computational power. In this paper, we address this problem by proposing a simple yet effective multi-scale feature modulation network to achieve a better trade-off between the efficiency of the model and the performance of the reconstruction. Specifically, we develop a multi-scale modulation module on top of a Visual Transformer (VIT)-like block. Within this module, we extract the features of the input image using a Multi-scale Spatial Feature Module (MSFM) to dynamically select representative features in the feature space of the image. Since the MSFM block handles the image features from a spatial point of view of the image, we further introduce the channel mixing module to perform the channel mixing. Extensive experimental results show that the proposed method is 8.5 times smaller in terms of network parameters than the state-of-the-art underwater image enhancement methods (e.g., Semi-uir), and achieves essentially the same performance with less computational cost. The code is available at https://github.com/zhengshijian1993/MFMN.
更多查看译文
关键词
Efficient convolutional network,Underwater image enhancement,Multi-scale spatial feature modulation,Channel mixing modulation
AI 理解论文
溯源树
样例
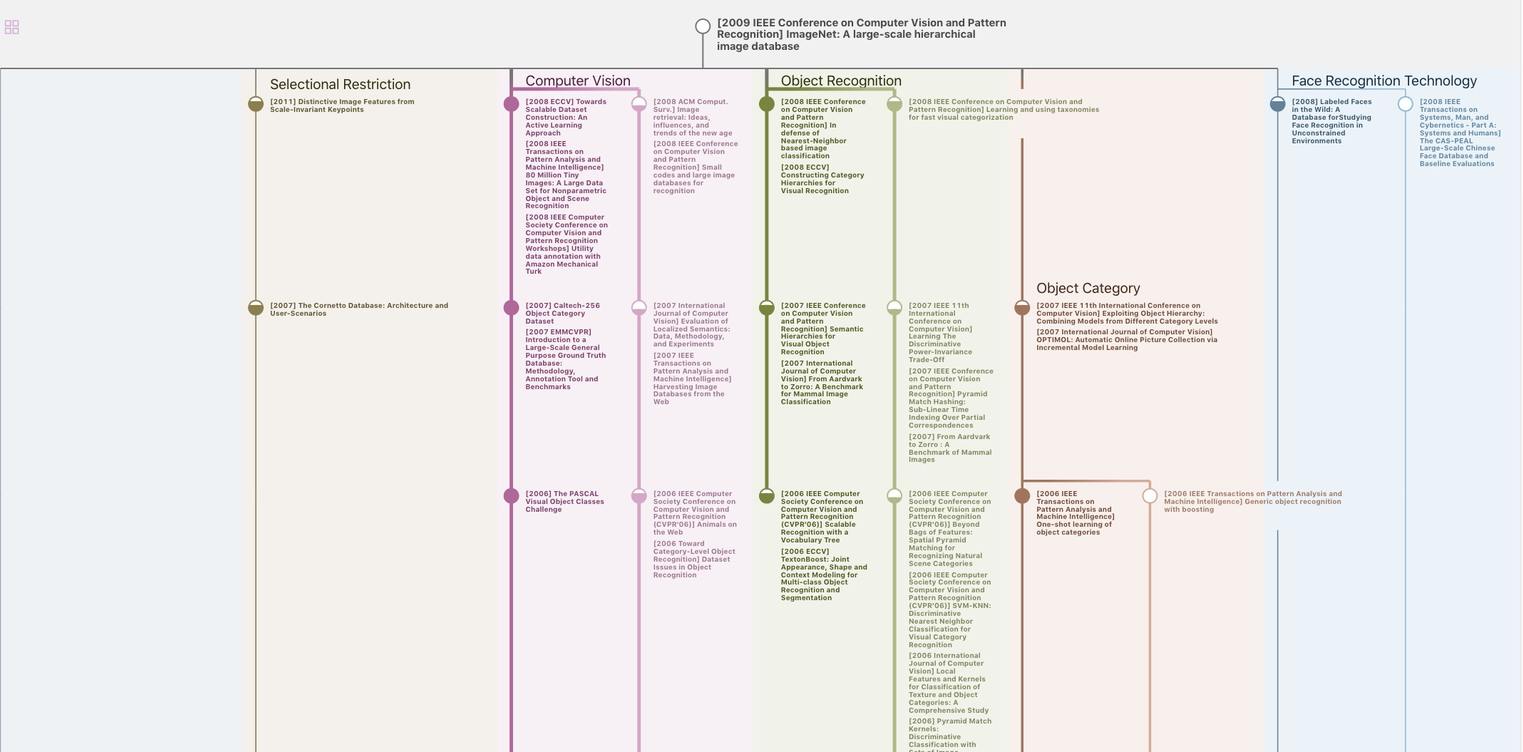
生成溯源树,研究论文发展脉络
Chat Paper
正在生成论文摘要