Predicting circRNA-miRNA interactions utilizing transformer-based RNA sequential learning and high-order proximity preserved embedding
ISCIENCE(2024)
摘要
A key regulatory mechanism involves circular RNA (circRNA) acting as a sponge to modulate microRNA (miRNA), and thus, studying their interaction has significant medical implications. In this field, there are currently two pressing issues that remain unresolved. Firstly, due to the scarcity of verified interactions, we require a minimal amount of samples for training. Secondly, the current models lack interpretability. Therefore, we propose SPBCMI, a method that combines sequence features extracted using the Bidirectional Encoder Representations from Transformer (BERT) model and structural features of biological molecule networks extracted through graph embedding to train a GBDT (Gradient-boosted decision trees) classifier for prediction. Our method yielded an AUC of 0.9143, which is currently the best for this problem. Furthermore, in the case study, SPBCMI accurately predicted 7 out of 10 circRNA-miRNA interactions. These results show that our method provides an innovative and high-performing approach to understanding the interaction between circRNA and miRNA.
更多查看译文
关键词
exhibit strong,model's performance
AI 理解论文
溯源树
样例
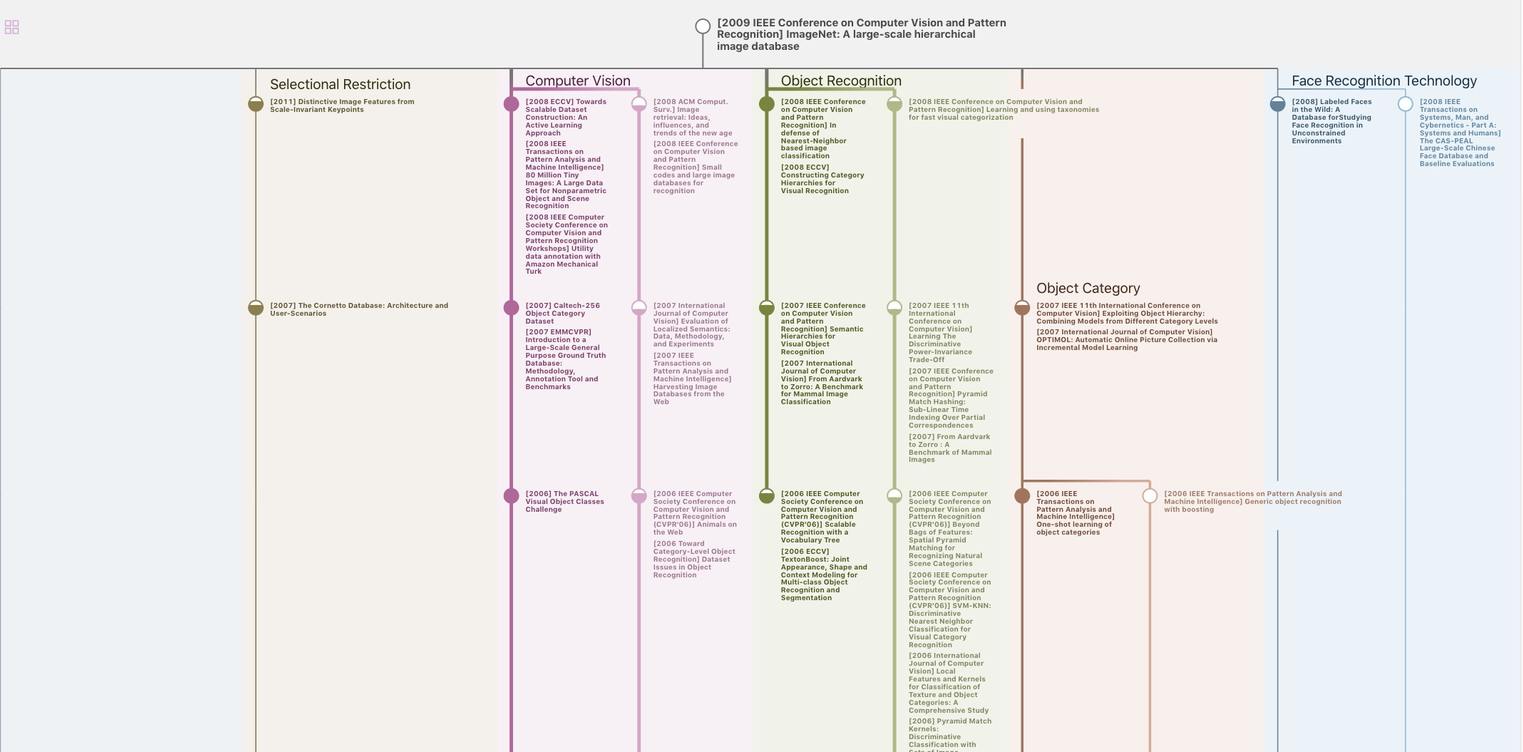
生成溯源树,研究论文发展脉络
Chat Paper
正在生成论文摘要