SGSR: style-subnets-assisted generative latent bank for large-factor super-resolution with registered medical image dataset
International Journal of Computer Assisted Radiology and Surgery(2024)
摘要
Purpose We propose a large-factor super-resolution (SR) method for performing SR on registered medical image datasets. Conventional SR approaches use low-resolution (LR) and high-resolution (HR) image pairs to train a deep convolutional neural network (DCN). However, LR–HR images in medical imaging are commonly acquired from different imaging devices, and acquiring LR–HR image pairs needs registration. Registered LR–HR images have registration errors inevitably. Using LR–HR images with registration error for training an SR DCN causes collapsed SR results. To address these challenges, we introduce a novel SR approach designed specifically for registered LR–HR medical images. Methods We propose style-subnets-assisted generative latent bank for large-factor super-resolution (SGSR) trained with registered medical image datasets. Pre-trained generative models named generative latent bank (GLB), which stores rich image priors, can be applied in SR to generate realistic and faithful images. We improve GLB by newly introducing style-subnets-assisted GLB (S-GLB). We also propose a novel inter-uncertainty loss to boost our method’s performance. Introducing more spatial information by inputting adjacent slices further improved the results. Results SGSR outperforms state-of-the-art (SOTA) supervised SR methods qualitatively and quantitatively on multiple datasets. SGSR achieved higher reconstruction accuracy than recently supervised baselines by increasing peak signal-to-noise ratio from 32.628 to 34.206 dB. Conclusion SGSR performs large-factor SR while given a registered LR–HR medical image dataset with registration error for training. SGSR’s results have both realistic textures and accurate anatomical structures due to favorable quantitative and qualitative results. Experiments on multiple datasets demonstrated SGSR’s superiority over other SOTA methods. SR medical images generated by SGSR are expected to improve the accuracy of pre-surgery diagnosis and reduce patient burden.
更多查看译文
关键词
Large-factor super-resolution,Medical image registration,Cross-modality image processing,Fine anatomy reconstruction
AI 理解论文
溯源树
样例
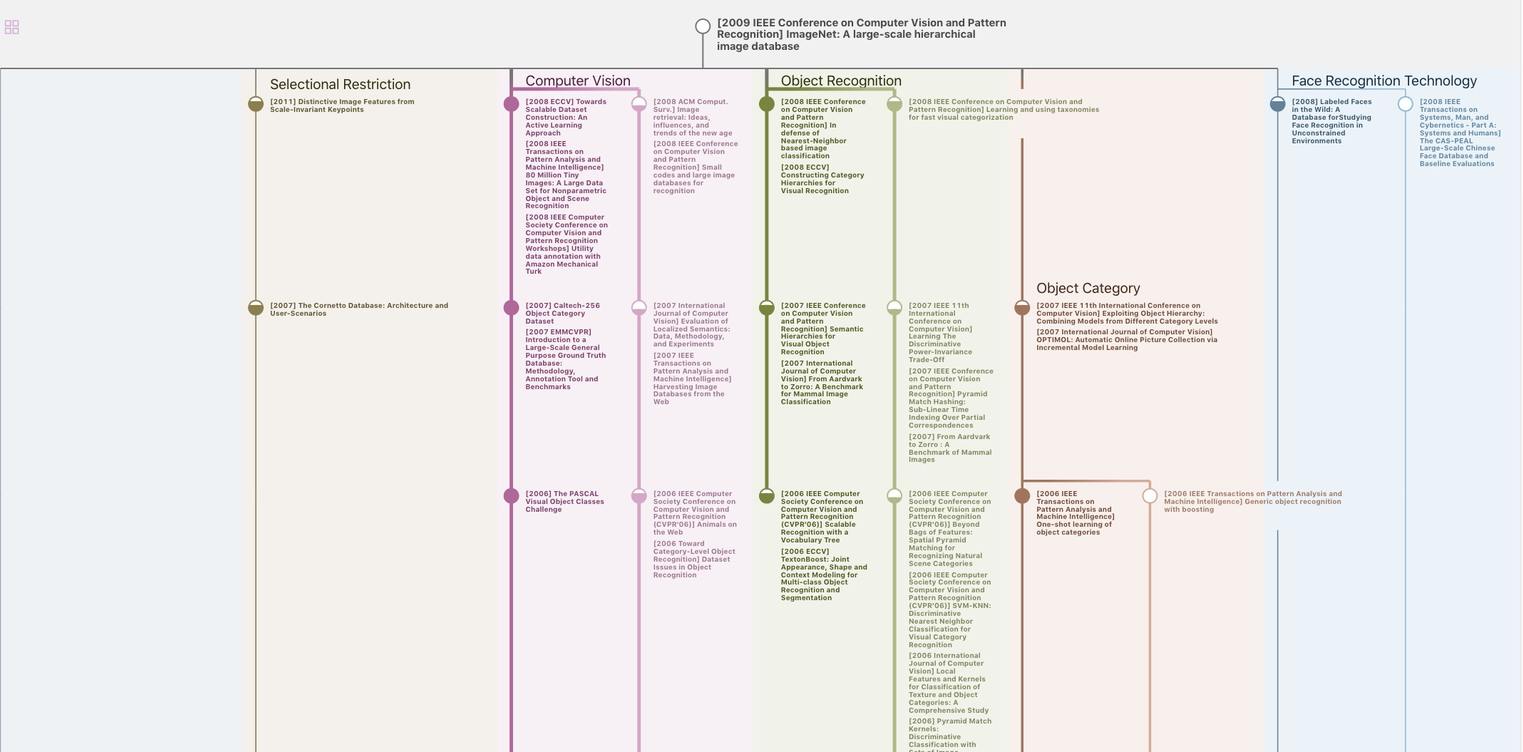
生成溯源树,研究论文发展脉络
Chat Paper
正在生成论文摘要