Machine learning approaches coupled with variational mode decomposition: a novel method for forecasting monthly reservoir inflows
Earth Science Informatics(2023)
摘要
Reliable and precise reservoir inflow predicting is very significant for water resource management. In this research, different single and hybrid Variational Mode Decomposition (VMD) with estimation models including K-star (K * ), Gaussian Process Regression (GPR), and Long Short-Term Memory (LSTM) models are developed to predict long-term time series of average monthly reservoir inflows in Baroon Dam ( RIBD m ) sited in Maku city, Northwest Iran. Using Pearson’s correlation coefficient ( PCC ) analysis among observed potential meteorological predictors and RIBD m confirms the rainfall ( P ave ) as the only effective input variable. To reduce the influence of overfitting problems and well-configuration of the approaches developed, an algorithm tuning over meta-parameters together with a trial-and-error technique are applied. The outcomes of modeling show that in the both single K * and hybrid VMD-K * models, the optimum value of the global blend parameter ( b ) is 10%, yet, by rising the value of b from 10 to 100%, the accuracy of both models are markedly reduced. In both standard LSTM and hybrid VMD-LSTM models, the ideal dropout rate ( P-rate ) is gained 0.5. Likewise, in both models, as number of hidden neurons ( NHN ) is held constant, increasing P-rate causes to decrease running time, also as P-rate remains constant, increasing NH N causes to increase running time. Results of statistical indicators and visual analysis of comparison plots approve the hybrid VMD-LSTM model as the superior method with an R 2 of 0.8, KGE of 0.87, RMSE of 1.15 (m 3 /s), and MBE of 0.15 (m 3 /s). Nonetheless, under the ideal scenario by the K * model, R 2 is 0.27, RMSE is 2.75 (m 3 /s), KGE is 0.43, and MBE is 0.15 (m 3 /s).
更多查看译文
关键词
Variational mode decomposition (VMD) algorithm,Gaussian process regression (GPR),K-star model,Long Short-Term Memory (LSTM) model,Monthly reservoir inflows
AI 理解论文
溯源树
样例
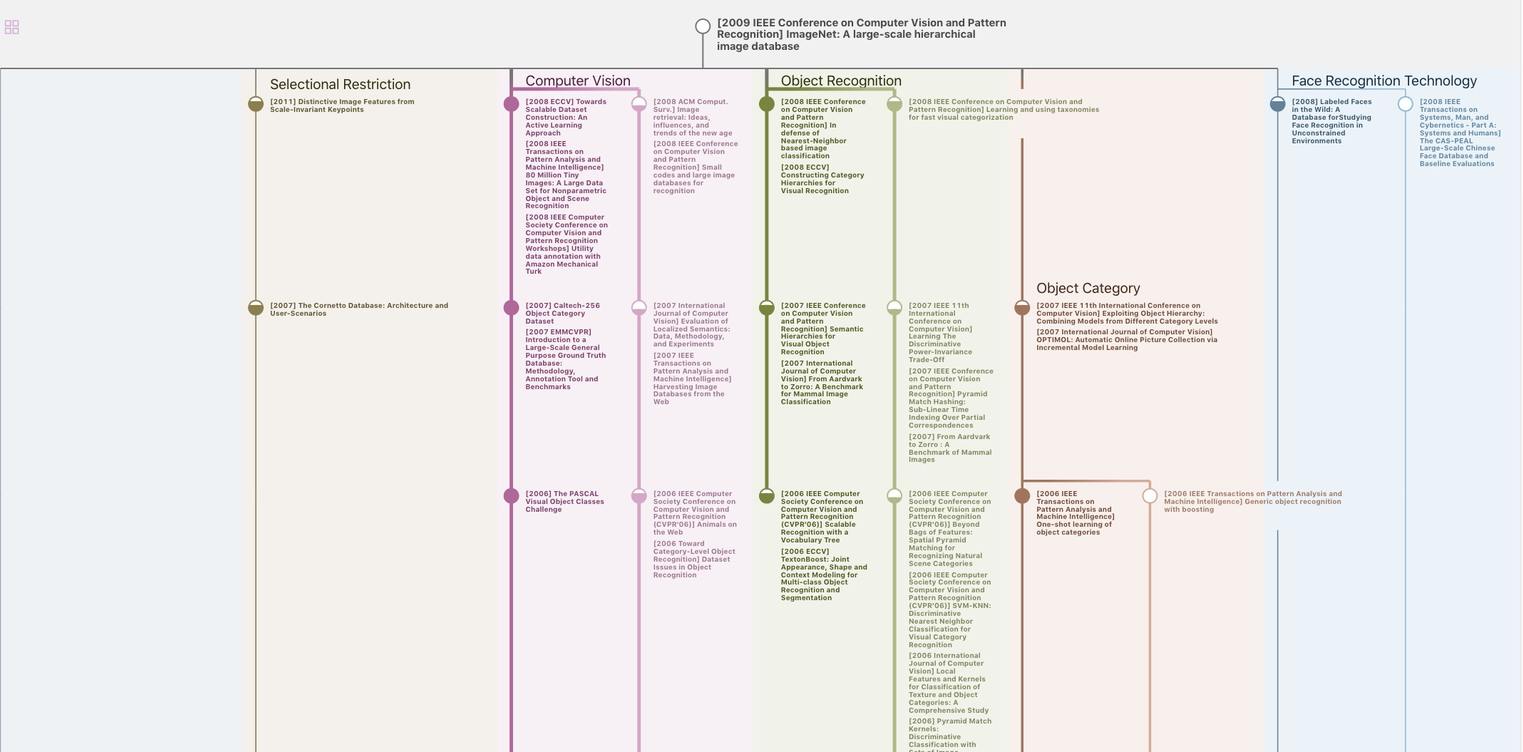
生成溯源树,研究论文发展脉络
Chat Paper
正在生成论文摘要